Mistral AI has introduced Mistral Small 3, a 24-billion-parameter open-source AI model that matches the performance of larger models, such as Meta’s Llama 3.3 70B, while being significantly faster and cheaper to operate. This could transform the economics of AI deployment, making advanced AI more accessible and cost-effective, especially for enterprises prioritizing privacy and efficiency.
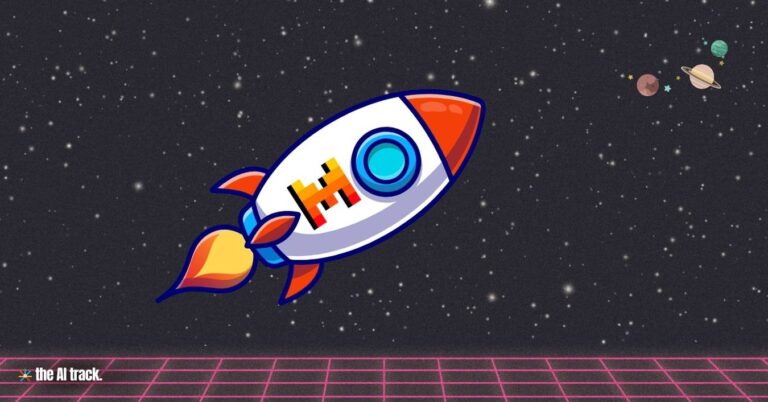
Mistral releases Small 3 24B model – Key Points
- Mistral Small 3 Overview:
- Mistral Small 3 is a 24-billion-parameter model optimized for low-latency performance, making it one of the most efficient in its class.
- It achieves over 81% accuracy on MMLU benchmarks and processes 150 tokens per second.
- Released under the Apache 2.0 license, allowing businesses to freely modify and deploy it.
- Matches or exceeds the performance of Meta’s Llama 3.3 70B and Qwen 32B despite being three times smaller.
- Provides an open-source alternative to proprietary models like GPT-4o Mini, positioning it as an attractive option for developers and businesses.
- Performance and Efficiency:
- Processes text more than 30% faster than GPT-4o Mini, with similar or superior accuracy scores.
- Optimized through innovative training techniques, focusing on efficiency rather than scale.
- Trained on 8 trillion tokens, significantly fewer than the 15 trillion used for comparable models, cutting down on computational costs.
- No reliance on reinforcement learning or synthetic training data, ensuring reliability and minimizing biases.
- Human evaluations show that Mistral Small 3 competes well in tasks like coding, math, and general knowledge, often outperforming larger models.
- Enterprise Applications:
- Designed for on-premises deployment, making it ideal for privacy-conscious sectors such as finance, healthcare, and manufacturing.
- Efficient enough to run on a single GPU, covering 80-90% of typical business use cases.
- Provides a robust foundation for businesses to customize the model further, enhancing its capabilities over time.
- Open-source nature allows for widespread innovation, reducing dependence on proprietary AI vendors.
- Market Context:
- Mistral’s release comes amid growing concerns over AI development costs, with DeepSeek’s claim of training a competitive model for just $5.6 million prompting scrutiny of Big Tech’s spending on AI infrastructure.
- The company’s focus on smaller, efficient models contrasts with the trend of large-scale, expensive AI systems.
- Evaluations using proprietary coding and generalist prompts confirm Mistral Small 3’s competitive edge, as demonstrated through benchmarks like Wildbench and Arena Hard.
- In direct comparisons, Mistral Small 3 proves to be a high-performing, cost-effective solution.
- Strategic Positioning:
- Valued at $6 billion, Mistral AI is positioning itself as Europe’s AI leader, with backing from Microsoft and plans for a future IPO.
- Mistral’s vision revolves around the democratization of AI through open-source models and permissive licenses, making advanced AI tools more accessible.
- The company promotes open-source adoption, encouraging greater collaboration within the AI community.
- Future Developments:
- Mistral plans to release additional models with improved reasoning capabilities, further proving the potential of its efficiency-driven approach.
- The company aims to push for pretraining advancements to refine generative and instructional features, continuing to reduce reliance on reinforcement learning.
Why This Matters:
Mistral Small 3 represents a critical shift in AI development, offering an open-source, high-performance model that challenges the status quo of big tech’s massive AI systems. By making AI more affordable and accessible, Mistral is lowering barriers for enterprises and fostering a new era of innovation. With its efficiency-first model, Mistral AI is poised to influence AI adoption across industries, reshape the competitive landscape, and drive greater control and customization for businesses.
The AI landscape is increasingly defined by the contrasting approaches of open source and closed source models. This article examines the nuances of each approach, exploring their benefits, challenges, and implications for businesses, developers, and the future of AI.
Read a comprehensive monthly roundup of the latest AI news!