Meta Platforms launched four new multimodal AI models (Llama 4 Scout, Maverick, Behemoth, and Reasoning) positioning them as best-in-class for open AI innovation, while committing $65 billion to infrastructure expansion. With open-weight access, superior systematic reasoning, new “expert” architectures, and broad integration across Meta’s platforms, the company escalates competition with OpenAI, Google, DeepSeek, and emerging rivals. However, concerns about benchmark transparency persist.
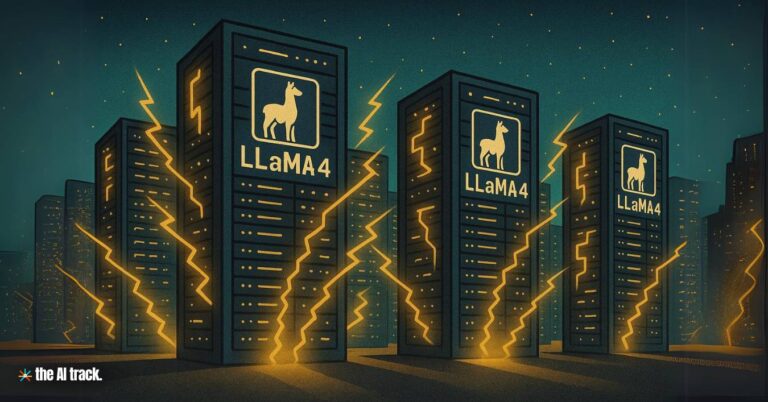
Article – Key Points
- New Releases: Meta unveiled the Llama 4 suite—Scout, Maverick, Behemoth, and Reasoning—on April 5, 2025, describing them as a major leap in open-source and open-weight AI development for document summarization, advanced reasoning, and multimodal tasks.
- Multimodal Strength: All models integrate text, images, video, and audio processing, enabling seamless cross-format content generation and analysis.
- Architecture Upgrade: Built on a “Mixture of Experts” (MoE) architecture, Llama 4 models increase efficiency by selectively activating relevant parameter subsets for each query, enhancing performance while reducing computational costs.
- Open Source and Open-Weight Commitment:
- Scout and Maverick are available as open-weight models via Meta’s Llama website and Hugging Face, allowing local deployment without cloud API reliance (though with potential licensing restrictions for commercial use).
- They are restricted from use or distribution within the European Union due to regulatory concerns over the EU’s AI governance laws.
- Expanded Portfolio:
- Scout: Lightweight model with 17 billion active parameters, 16 experts, a 10 million-token context window; optimized for deployment on a single Nvidia H100 GPU. Outperforms Google’s Gemma 3, Gemini 2.0 Flash-Lite, and Mistral 3.1 in benchmark tests.
- Maverick: 400 billion total parameters (17 billion active across 128 experts); optimized for general AI assistant tasks like creative writing and reasoning. Competes strongly with OpenAI’s GPT-4o, Gemini 2.0 Flash, and DeepSeek V3, achieving high efficiency with fewer active parameters.
- Behemoth: Over 2 trillion total parameters, with 288 billion active across 16 experts; acts as a “teacher model” for smaller Llama 4 models. Excels in STEM tasks, multilingual processing, and image-related tasks, outperforming GPT-4.5, Claude Sonnet 3.7, and Gemini 2.0 Pro in several evaluations.
- Reasoning: Aimed at advancing logical inference capabilities; limited technical details available yet.
- Performance Claims and Controversy:
- Meta asserts Maverick outperforms GPT-4o and Gemini 2.0 in coding, reasoning, and image benchmarks but trails GPT-4.5 and Gemini 2.5 Pro in some areas.
- TechCrunch and AI researchers revealed that the Maverick version showcased on LM Arena was a special “experimental chat version,” optimized differently from the publicly available variant, raising concerns over benchmark transparency and real-world model predictability.
- Development Challenges: The Information reported that early versions of Llama 4 underperformed in reasoning, math, and conversational tasks compared to OpenAI’s leading models, causing launch delays.
- Compute Capacity Leadership:
- Meta operates approximately 350,000 Nvidia H100 GPUs, nearly double the compute resources of OpenAI and xAI (both at ~200,000 H100s).
- Meta is expanding its own data centers and developing custom AI chips to further secure its infrastructure dominance.
- Benchmark Scrutiny: Broader skepticism continues over the benchmarking practices, particularly around inconsistencies between the “tested” and “released” versions of Maverick.
- Strategic Context: Meta’s push for Llama 4 acceleration was fueled by competitive pressure from Chinese lab DeepSeek, which gained prominence with its R1 and V3 models. Meta established internal “war rooms” to close the performance gap.
- Broader Deployment:
- Llama 4 models are integrated across Facebook, Instagram, Messenger, and WhatsApp, enhancing chatbots, ad targeting systems, and content generation models.
- Third-party developers including LinkedIn and Pinterest have started integrating Llama models into their platforms.
- Financial Scale: Meta plans a $65 billion investment in 2025 to scale AI infrastructure and meet growing demands.
- Developer Benefits: The MoE structure enables lower compute requirements for high-performance deployment, facilitating broader access to powerful AI models.
Why This Matters:
Meta’s aggressive expansion with open-weight, multimodal Llama 4 models positions it at the forefront of global AI development. With unmatched compute resources and the ability to locally deploy powerful models, Meta offers developers and enterprises unprecedented flexibility and capability. However, questions around benchmark integrity and regulatory compliance signal increasing challenges in maintaining trust and navigating AI governance. Llama 4’s integration into both consumer platforms and enterprise ecosystems could fundamentally shift the competitive balance across the AI industry.
Explore the key differences between open source vs closed source AI, including their benefits, challenges, and implications for the future of AI
Read a comprehensive monthly roundup of the latest AI news!