
AI is unlocking the secrets of life by advancing our understanding of biological systems, from genetics to ecosystems. This page highlights the key AI breakthroughs in Biology that are driving innovation in biological research and applications.
Each section offers insights into how AI is transforming different sectors, providing a comprehensive view of its impact across a wide range of disciplines.
AI Breakthroughs in Computer Science - At a Glance
The Revolution of AI in Healthcare: From Workflow Optimization to Personalized Medicine
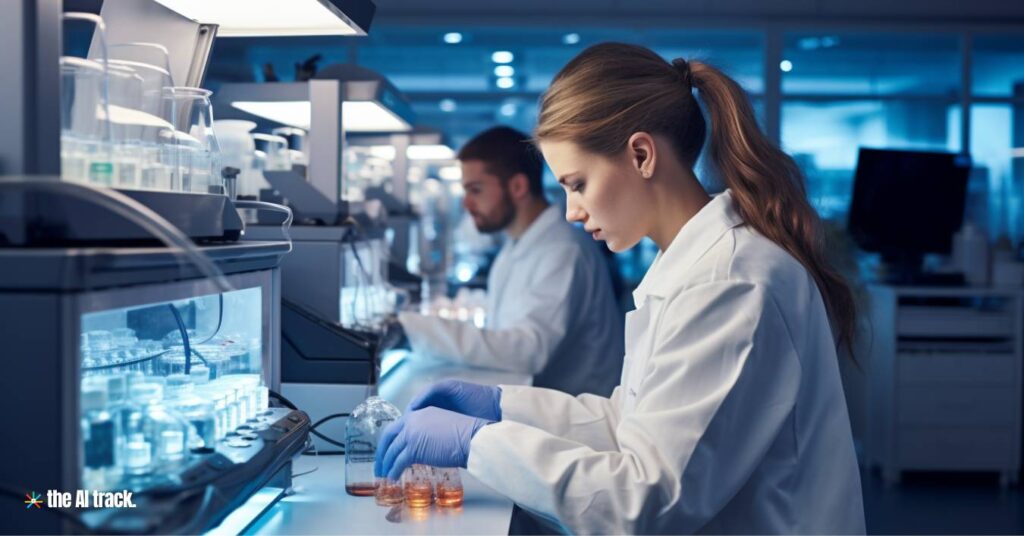
The AI Track’s extensive analysis of the impact of AI in medical technology – how it is transforming healthcare by streamlining workflows, facilitating personalized medicine, and beyond. Explore the profound impact of AI in the medical field.
Scientists have utilized AI to design antibodies from scratch
Original Article Title:
‘A landmark moment’: scientists use AI to design antibodies from scratch
Source: Nature
Date:
Key Takeaway
Scientists have utilized generative AI to design antibodies from scratch, marking a significant advancement in antibody development.
Summary
- Researchers have employed generative AI to create entirely novel antibodies, a groundbreaking achievement.
- The modified protein-design tool facilitated this process, offering potential to address challenging drug targets more effectively.
- Despite this milestone, AI-designed antibodies are still distant from practical application in clinical settings.
- References to related studies and articles provide further context and avenues for exploration in the field of structural biology, machine learning, and drug discovery.
- Job listings in the biotechnology and academic sectors indicate ongoing research and opportunities in relevant fields worldwide.
AI creates new gene-editing tools
Original Article Title:
‘ChatGPT for CRISPR’ creates new gene-editing tools
Source: Nature
Date:
Researchers are now utilizing generative AI to design new CRISPR gene-editing tools, potentially outperforming natural systems. These AIs, trained on vast protein and genome data, are creating effective CRISPR systems, like the promising OpenCRISPR-1. This approach could revolutionize gene editing, particularly for precise medical applications. The new AI-generated tools are poised to enhance the accessibility and efficacy of gene-editing technologies.
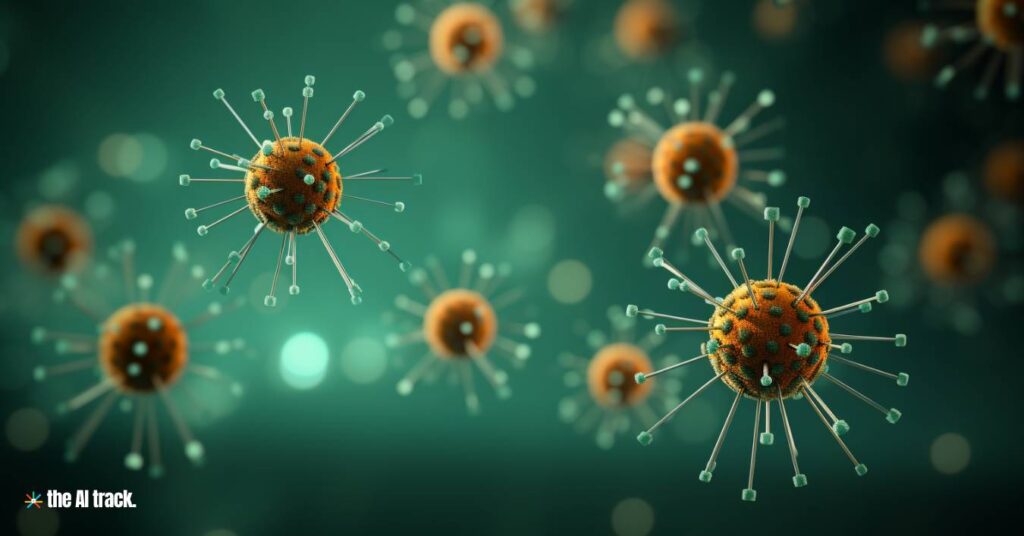
Scientists develop new AI method to create material 'fingerprints'
Original Article Title:
Scientists develop new AI method to create material ‘fingerprints’
Source: ScienceDaily
Date: 16 July 2024
Scientists at the DOE’s Argonne National Laboratory have developed an AI method that creates “fingerprints” of materials, allowing for a deeper understanding of how materials change under stress and relaxation.
Key Points:
- The method uses X-ray photon correlation spectroscopy (XPCS) combined with AI to recognize patterns in materials.
- This approach can capture complex scattering data, transforming it into easily interpretable “fingerprints.”
- The AI technique involves an autoencoder to create latent representations of the data.
Why This Matters: This advancement can significantly enhance the study of material properties, leading to better material design and applications.
Voices of AI: Insights and Quotes from the Top’ offers a unique compilation of quotes and perspectives from top AI executives and thinkers.
Researchers have developed a new type of magnet that does not rely on rare-earth metals
Original Article Title:
A Radical New Magnet Without Rare-Earth Metals Is About to Change Motors Forever
Source: Popular Mechanics
Date: 18 June 2024
Researchers have developed a new type of magnet using AI that does not rely on rare-earth metals, potentially reducing environmental and geopolitical issues.
Key Points:
- The magnet is composed of iron and nickel, eliminating the need for rare-earth elements.
- AI was instrumental in identifying the optimal composition and structure of the magnet.
- This innovation could impact industries reliant on magnets, such as renewable energy and electronics, by providing a more sustainable and cost-effective alternative.
Why This Matters: The development of rare-earth-free magnets can mitigate environmental damage and reduce reliance on geopolitically sensitive materials, promoting sustainable technological advancements.
An AI-powered virtual rat brain allows researchers to simulate and study neurological processes in unprecedented detail
Original Article Title:
Harvard, Google Unveil AI-Powered Virtual Rat for Brain Studies
Source: Tech Times
Date: 12 June 2024
Harvard and Google have unveiled an AI-powered virtual rat brain, a significant breakthrough that allows researchers to simulate and study neurological processes in unprecedented detail.
Key Points:
- Collaboration: The project combines Harvard’s neuroscientific expertise with Google’s AI capabilities.
- Technology: The virtual brain replicates rat brain functions, providing insights into brain activity and potential neurological treatments.
- Research Impact: This tool could accelerate the understanding of brain diseases and the development of new therapies.
Why This Matters: This innovation has the potential to revolutionize neuroscience by offering a new way to explore brain functions and disorders.
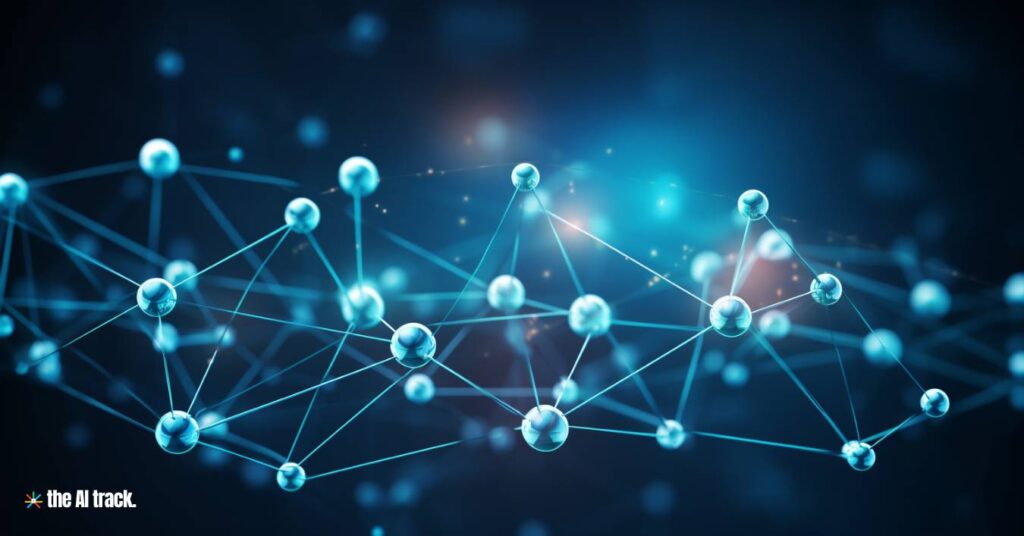
NASA's Perseverance rover is utilizing AI to enhance scientific exploration on Mars, by enabling rovers to autonomously identify and analyze rock samples
Original Article Title:
Here’s How AI Is Changing NASA’s Mars Rover Science
Source: NASA – Jet Propulsion Laboratory
Date: 16 July 2024
NASA’s Perseverance rover is utilizing AI in innovative ways to enhance scientific exploration on Mars. AI is revolutionizing space exploration by enabling rovers to autonomously identify and analyze rock samples, increasing efficiency and scientific discovery. This marks the first time AI has been used on Mars for real-time decision-making based on rock composition analysis.
Key Points:
- AI-powered PIXL: The Perseverance rover’s Planetary Instrument for X-ray Lithochemistry (PIXL) uses AI for ‘adaptive sampling.’ The AI autonomously positions the instrument near the rock and analyzes PIXL scans in real-time to identify minerals of interest for deeper study.
- Efficiency and Autonomy: AI-driven adaptive sampling saves time by allowing PIXL to reach conclusions without human intervention, unlike previous methods that required rescanning rocks based on scientists’ observations.
- Mineral Mapping: Another AI system aids PIXL in creating detailed mineral maps of rock surfaces. PIXL scans a small area, firing an X-ray beam to create a grid of dots, each revealing the chemical composition of minerals.
- Scientific Significance: Minerals offer vital clues about Mars’ past. AI helps scientists identify carbonates, which indicate water formation, and phosphates, potential nutrient sources for past microbial life.
- Long Dwells: PIXL’s AI enables it to automatically perform ‘long dwells,’ collecting more data when specific minerals are detected. Machine learning enhances the list of minerals PIXL can focus on.
- Curiosity Rover: Curiosity, another Mars rover, also employs AI for autonomous laser zapping of rocks based on their shape and color, revealing their chemical composition.
- Future of Space Exploration: Advancements in AI, like those used in Perseverance and Curiosity, are paving the way for greater autonomy in future space missions, especially those venturing deeper into the solar system where communication delays are significant.
Why This Matters:
The integration of AI in Mars rovers signifies a major advancement in space exploration. By enabling autonomous decision-making and real-time data analysis, AI accelerates scientific discovery, optimizes mission efficiency, and lays the groundwork for future missions to explore more distant celestial bodies with greater independence.
Explore our AI Books hub for detailed summaries of the most influential AI books. Discover key insights, actionable takeaways, and viral quotes.
AI Guides Cyborg Worms in Revolutionary Neuroscience Experiment
Original Article Title:
Scientists Make ‘Cyborg Worms’ with a Brain Guided by AI
Source: Scientific American
Date: 2 September 2024
Key Takeaway: Scientists have created “cyborg worms” by integrating AI directly into the nervous systems of tiny worms, enabling them to navigate toward food with the assistance of AI guidance.
Key Points:
- AI Collaboration: Researchers used deep-reinforcement learning to train an AI to control one-millimeter-long Caenorhabditis elegans worms.
- Optogenetic Engineering: The worms were engineered to respond to light, which the AI used to direct their movement toward Escherichia coli.
- Successful Outcomes: In five out of six genetic lines tested, the AI-directed worms reached their targets faster than those left alone.
- Future Applications: The technique may eventually improve human therapies, such as deep-brain stimulation for Parkinson’s disease.
Why This Matters: This research represents a significant step in merging biological and artificial systems, with potential applications in medicine and beyond.
AlphaProteo: The AI System That’s 10x Stronger at Protein Design
Original Article Title:
AlphaProteo generates novel proteins for biology and health research
Source: Google DeepMind
Date: 5 September 2024
Key Takeaway:
DeepMind’s new AI system, AlphaProteo, can design novel proteins from scratch, with the potential to revolutionize biological research and drug discovery. Unlike its predecessor, AlphaFold, which predicted protein structures, AlphaProteo can create proteins that bind to target molecules, accelerating drug design, biosensor development, and disease research.
Key Points:
- AlphaProteo’s Capability: DeepMind’s AlphaProteo is an AI system designed to create entirely new protein binders, with applications in biological research, drug discovery, and disease understanding. By designing these binders, AlphaProteo has the potential to address various research needs, such as creating proteins that interact with targets related to cancer, viruses, and autoimmune diseases.
- Application in Drug Discovery: The AI has already designed proteins that bind to molecules associated with diseases like cancer and diabetes. AlphaProteo successfully generated binders for significant targets, such as VEGF-A, involved in cancer, and SARS-CoV-2 spike protein, linked to COVID-19 infection. These binders are crucial for creating new drugs and therapies.
- Higher Success Rates: AlphaProteo demonstrated better binding affinity compared to traditional methods. For example, it achieved 3 to 300 times better binding success across seven target proteins. When tested on BHRF1, a viral protein, 88% of AlphaProteo’s designed molecules successfully bound to the target in lab experiments.
- Broader Research Applications: Beyond drug development, AlphaProteo can contribute to other fields such as biosensors, diagnostics, and even agriculture. The AI system enables researchers to tackle diverse challenges, including imaging, disease diagnostics, and enhancing crop resistance to pests.
- Experimental Validation: AlphaProteo’s output was validated in DeepMind’s wet lab and by external research groups, including the Francis Crick Institute. The validation confirmed the effectiveness of the designed protein binders, such as those targeting SARS-CoV-2, in preventing viral infections in cells, proving the potential real-world applications of these AI-designed proteins.
- Challenges and Limitations: Despite its success, AlphaProteo faced difficulties in creating binders for TNFα, a protein involved in autoimmune diseases. This demonstrates the complexity of certain targets, but DeepMind is committed to refining the AI to tackle more challenging problems.
- Towards Responsible Protein Design: DeepMind is actively working with the scientific community to ensure responsible development and biosecurity. By collaborating with external experts, DeepMind aims to set ethical guidelines and best practices in AI-driven protein design, balancing innovation with safety.
Why This Matters:
AlphaProteo’s breakthrough in designing proteins could accelerate advances in drug development, disease understanding, and bioengineering. Its high success rate and ability to design strong binders for critical proteins could drastically reduce the time required for biological experiments. By making protein design more efficient and precise, AlphaProteo has the potential to transform numerous fields, from healthcare to agriculture, driving innovation in both research and practical applications.
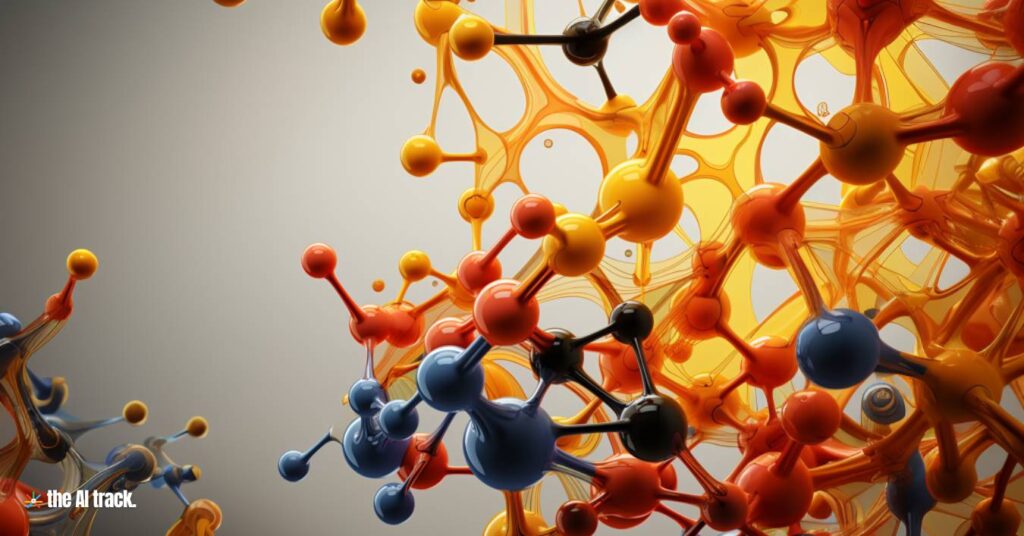
Chai Discovery just launched Chai-1, a multi-modal AI model for molecular structure prediction, outperforming Google DeepMind’s AlphaFold 3
Original Article Title:
Introducing Chai-1: Decoding the molecular interactions of life
Source: Chai Discovery
Date: 9 September 2024
Key Takeaway: Chai Discovery has launched Chai-1, a state-of-the-art multi-modal AI model designed for molecular structure prediction, surpassing existing models like AlphaFold in certain benchmarks. The model aims to revolutionize drug discovery and biomolecular research by offering free access for commercial and non-commercial use.
Key Points:
- Introduction of Chai-1:
- Chai Discovery, a six-month-old startup, launched Chai-1, a multi-modal foundation model for molecular structure prediction. It is designed for various tasks relevant to drug discovery, predicting interactions between proteins, small molecules, DNA, RNA, and more.
- The model is free for both commercial and non-commercial applications, providing researchers and companies with cutting-edge AI tools to accelerate molecular research.
- Performance and Benchmarks:
- Chai-1 outperformed DeepMind’s AlphaFold 3 on the PoseBusters benchmark, scoring 77% versus AlphaFold’s 76%.
- Chai-1 also achieved a Cα LDDT score of 0.849 on the CASP15 protein monomer structure prediction set, exceeding the performance of ESM3-98B (0.801).
- Chai-1 is capable of folding multimers more accurately than AlphaFold-Multimer, boasting a 69.8% DockQ acceptable prediction rate without requiring multiple sequence alignments (MSAs).
- Unique Features and Capabilities:
- Chai-1 operates in single-sequence mode without relying on MSAs while maintaining high accuracy, making it the first model capable of predicting multimer structures using single sequences at AlphaFold-Multimer quality.
- It can also be prompted with real-world lab data, which increases prediction accuracy by double-digit percentage points. This feature is especially impactful in antibody-antigen structure prediction, where epitope conditioning boosts performance.
- Open Access to Chai-1:
- Chai-1 is available through a web interface for direct use in drug discovery and other commercial applications. The model’s weights and inference code are also released as a software library for non-commercial use, encouraging widespread collaboration in the research community.
- The startup’s mission is to collaborate with the research and industrial sectors to benefit the entire AI and biological research ecosystem.
- Chai Discovery’s Vision:
- Founded by veterans from companies like OpenAI, Google X, Meta FAIR, and Stripe, Chai Discovery aims to transform biology from a science into an engineering discipline by building further AI foundation models.
- The company has already raised $30 million in funding from investors like Thrive Capital and OpenAI, with the goal of accelerating drug discovery and biomolecular research through advanced AI technologies.
Why This Matters:
Chai-1 represents a significant advancement in molecular structure prediction, offering a faster and more accessible approach to drug discovery. With its ability to predict biomolecular interactions more accurately than previous models, Chai-1 has the potential to speed up the discovery of new therapeutic compounds. By releasing the model for free, Chai Discovery is democratizing access to advanced AI tools, which could drive innovation across the pharmaceutical industry and beyond. However, despite its promise, the drug discovery process still requires rigorous, human-led testing, making AI just one piece of the larger puzzle in developing new drugs.
AI Reveals Key Mechanism in Sperm-Egg Binding During Fertilization
Original Article Title:
AI reveals how sperm sticks to egg during fertilization
Source: Science
Date: 17 October 2024
Key Takeaway:
Researchers, using AI tool AlphaFold, have identified a complex of three proteins (Izumo1, Spaca6, Tmem81) on sperm that locks onto an egg’s receptor protein, Juno. This discovery helps explain how sperm sticks to an egg, revealing conserved mechanisms across species from zebrafish to mammals.
Key Points:
- Protein Interaction Discovery: AI-powered analysis revealed a sperm protein trimer responsible for binding to the egg’s Juno receptor.
- Verification: Experiments with zebrafish confirmed the trimer’s importance in fertilization.
- Evolutionary Insight: The sperm complex is conserved across vertebrates, while egg receptors vary.
- Clinical Potential: This knowledge could assist infertility treatments and contraceptive development.
Why This Matters:
These findings advance reproductive biology, with potential future applications in treating infertility and developing new contraceptive methods.
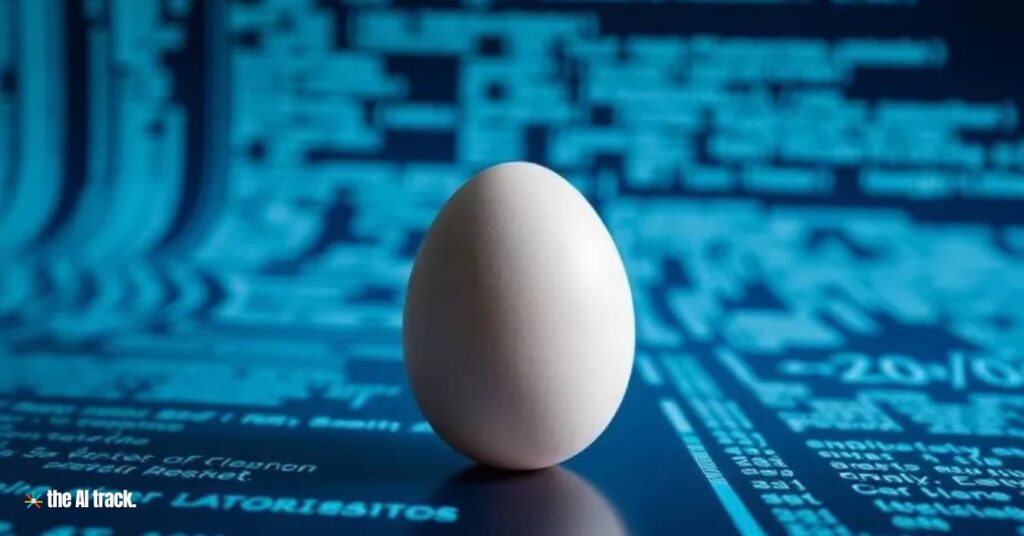
Meta is Accelerating Materials Discovery: Open-Source Boost for Scientists
Original Article Title:
The race to find new materials with AI needs more data. Meta is giving massive amounts away for free
Source: MIT Technology Review
Date: 17 October 2024
Key Takeaway:
Researchers, using AI tool AlphaFold, have identified a complex of three proteins (Izumo1, Spaca6, Tmem81) on sperm that locks onto an egg’s receptor protein, Juno. This discovery helps explain how sperm sticks to an egg, revealing conserved mechanisms across species from zebrafish to mammals.
Key Points:
- Protein Interaction Discovery: AI-powered analysis revealed a sperm protein trimer responsible for binding to the egg’s Juno receptor.
- Verification: Experiments with zebrafish confirmed the trimer’s importance in fertilization.
- Evolutionary Insight: The sperm complex is conserved across vertebrates, while egg receptors vary.
- Clinical Potential: This knowledge could assist infertility treatments and contraceptive development.
Why This Matters:
These findings advance reproductive biology, with potential future applications in treating infertility and developing new contraceptive methods.
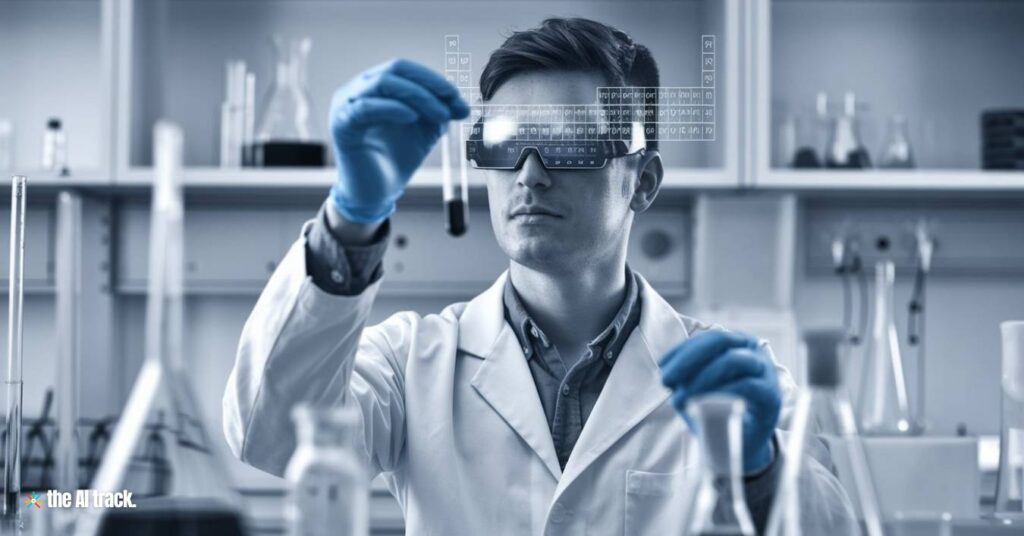
AlphaFold 3 by Google’s DeepMind: A New Era in Biomolecular Modeling and Drug Discovery
Original Article Title:
Accurate structure prediction of biomolecular interactions with AlphaFold 3
Source: Nature
Date: 8 May 2024
Key Takeaway:
AlphaFold 3, developed by Google DeepMind, introduces groundbreaking advancements in biomolecular structure prediction, poised to transform drug discovery and biological research, potentially creating a multi-billion-dollar business landscape.
Key Points:
- Revolutionary Model Advancements: AlphaFold 3, launched by Google DeepMind, significantly enhances the predictive modeling of complex biological structures, such as proteins, DNA, RNA, and their interactions, offering unparalleled accuracy and broadening its application scope beyond its previous iterations.
- Potential Commercial Impact: Demis Hassabis, CEO of DeepMind, projects the AI-driven drug discovery market to exceed $100 billion, with AlphaFold 3 positioned as a central technology. Isomorphic Labs, an Alphabet subsidiary, aims to capitalize on this by applying AlphaFold to real-world drug design challenges.
- Strategic Partnerships: Isomorphic Labs has forged partnerships with pharmaceutical giants like Eli Lilly & Co. and Novartis, potentially valued near $3 billion, highlighting the commercial viability and high stakes of integrating AI in drug development.
- Innovative Platform Deployment: The AlphaFold Server, launched alongside AlphaFold 3, offers an accessible platform for scientists globally to utilize the advanced capabilities of AlphaFold 3 for non-commercial purposes, promoting widespread adoption and innovation in the scientific community.
- Enhanced Accessibility and Utility: The server simplifies the interaction with complex AI models, allowing biologists and researchers easy access without needing deep technical knowledge, thus democratizing high-level scientific inquiry.
- Future Prospects and Challenges: While the technology heralds a new era in biotechnology and AI, the industry faces challenges like proving clinical success, navigating regulatory landscapes, and addressing public and market expectations.
This synthesis incorporates insights from the recent Bloomberg interview and article on DeepMind’s strategic advancements with AlphaFold 3, underscoring its potential to reshape the future of drug discovery and biological research significantly.
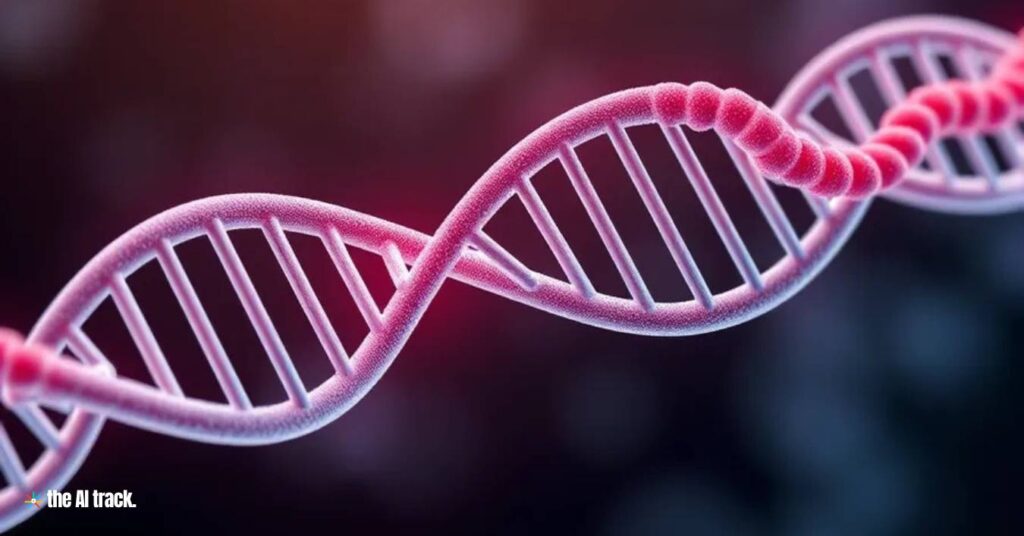
AI Transformed Biology, But It’s Just the Beginning
Original Article Title:
How AI Revolutionized Protein Science, but Didn’t End It
Source: Quanta Magazine
Date: 26 June 2024
Summary:
The discovery of AlphaFold2 by Google DeepMind in 2020 dramatically altered the landscape of protein science by solving one of biology’s greatest challenges: predicting the 3D structure of proteins from their amino acid sequences.
AlphaFold2 outperformed decades of previous attempts by leveraging deep learning to predict protein structures with an unprecedented level of accuracy.
This achievement opened the door to accelerated research, inspiring new AI-driven approaches and altering experimental workflows.
However, while AlphaFold2 has become an essential tool for structural biologists, it hasn’t eliminated the need for traditional experimental methods.
AI systems like AlphaFold2 still have limitations in predicting the dynamic nature of proteins and their interactions with other molecules in living cells.
The future lies not only in predicting protein structures but also in designing novel proteins for new medical and industrial applications.
As AI continues to evolve, the balance between understanding the processes behind these biological structures and using AI as a predictive tool remains a key debate within the scientific community.
Key Points:
- AlphaFold2 Breakthrough: Solved the protein folding problem by using deep learning to predict 3D structures from amino acid sequences, achieving over 90% accuracy.
- Impact on Research: AlphaFold2’s predictions accelerate scientific research, providing faster results and allowing for better hypotheses generation. It also aids in drug discovery by helping to identify protein targets.
- Limitations: AI cannot yet simulate the dynamic changes in proteins within the complex environment of living cells. Tools like AlphaFold2 require validation through experiments and struggle with multifaceted proteins or those that change structure.
- The Next Frontier: Researchers are moving towards protein design, where AI is used not only to predict structures but to design new proteins that don’t exist in nature. This could lead to breakthroughs in medicine and sustainability.