
AI is not just a tool but a transformative force within computer science itself. This page highlights the most important AI breakthroughs in computer science – advancements that are pushing the boundaries of computing.
Browse all the other fields in our curated collection of the most important AI Breakthroughs. Each section offers insights into how AI is transforming different sectors, providing a comprehensive view of its impact across a wide range of disciplines.
AI Breakthroughs in Computer Science - At a Glance
The Essential Guide to AI Infrastructure: All you need to know
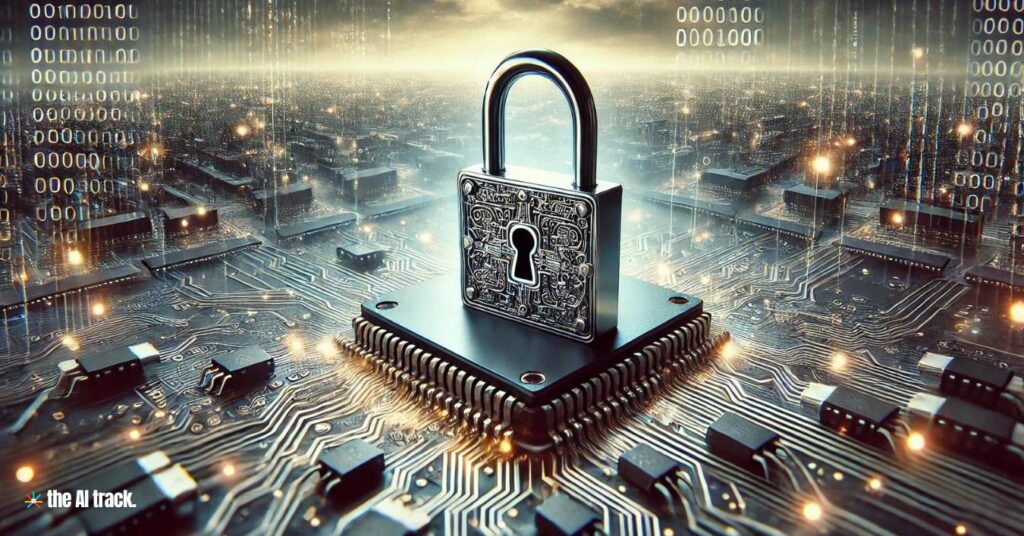
The AI Track’s extensive analysis of the critical components of AI infrastructure, including hardware, software, and networking, that are essential for supporting AI workloads. The article also examines the benefits, challenges, and emerging trends in AI infrastructure.
KnowHalu is a cutting-edge AI method to detect hallucinations in text generated by LLMs
Original Article Title:
KnowHalu: A Novel AI Approach for Detecting Hallucinations in Text Generated by Large Language Models (LLMs)
Source: MarkTechPost
Date: 12 May 2024
A team of researchers from the University of Illinois Urbana-Champaign, UChicago, and UC Berkeley has developed KnowHalu, a novel AI method for detecting hallucinations in text generated by large language models (LLMs). Hallucinations occur when AI produces content that seems accurate but is incorrect or irrelevant. KnowHalu improves accuracy through a two-phase process: checking for non-fabrication hallucinations and employing structured and unstructured external knowledge sources for deeper factual analysis. Rigorous testing shows KnowHalu significantly outperforms existing methods, enhancing the reliability of AI-generated content in critical fields like medicine and finance.
Russian researchers have developed Headless-AD, a self-adapting AI model capable of learning new tasks without human intervention
Original Article Title:
Russian researchers unveil AI model that adapts to new tasks without human input
Source: Natural News
Date: 30 July 2024
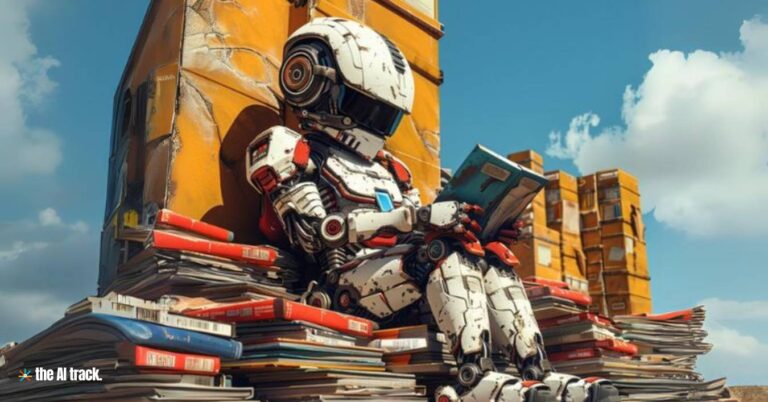
Russian researchers have developed Headless-AD, a self-adapting AI model capable of learning new tasks without human intervention, enhancing flexibility and applicability in various fields.
Key Points:
- Development and Presentation: The Headless-AD AI model was developed by the T-Bank AI Research Laboratory and the Moscow-based Artificial Intelligence Research Institute. It was presented at the International Conference on Machine Learning in Vienna.
- Innovative Capability: Headless-AD can adapt to new tasks and contexts without requiring human input, overcoming the limitations of traditional AI models that need extensive data and re-learning.
- Algorithm Distillation: The model uses an enhanced version of algorithm distillation, enabling it to perform five times more actions than it was originally trained for.
- Versatility and Applications: The AI can adapt to specific conditions based on generalized data, making it suitable for various fields, including space technologies and smart home assistants.
- Passing the Coffee Test: The model’s ability to handle diverse tasks without re-learning suggests it might pass Steve Wozniak’s “coffee test,” showcasing its practical adaptability in everyday scenarios.
Why This Matters: Headless-AD represents a significant advancement in AI technology, potentially transforming various industries by providing a highly adaptable and efficient AI model. This innovation can reduce the cost and time associated with AI training and deployment, enhancing the practicality of AI in dynamic and unpredictable environments.
AI achieves silver-medal standard solving International Mathematical Olympiad problems.
Original Article Title:
Google claims math breakthrough with proof-solving AI models
Source: Ars Technica
Date: 26 July 2024
Google DeepMind’s AI systems, AlphaProof and AlphaGeometry 2, achieved a performance equivalent to a silver medal in the International Mathematical Olympiad (IMO) by solving four out of six problems. This marks a significant milestone in AI’s ability to tackle complex mathematical problems.
Key Points:
- AI Performance: AlphaProof and AlphaGeometry 2 solved four out of six IMO problems, earning 28 out of 42 points, just shy of the gold medal threshold (29 points).
- Problem Types Solved: AlphaProof tackled two algebra problems and one number theory problem, while AlphaGeometry 2 solved the geometry problem.
- Speed and Process: The AI solved some problems within minutes and others in up to three days. Problems were translated into formal mathematical language for the AI to process.
- Historical Success: AlphaGeometry 2 improved from solving 53% to 83% of historical IMO geometry problems over the past 25 years.
- Human Involvement: Prominent mathematicians scored the AI’s solutions. Humans translated problems into the formal language Lean before the AI’s processing.
- Expert Commentary: Sir Timothy Gowers noted the AI’s achievement but highlighted that it required significantly more time and faster processing than human competitors. He suggested the AI hasn’t “solved mathematics” but has the potential to become a valuable research tool.
Why This Matters: The achievement demonstrates AI’s growing capabilities in complex problem-solving and its potential to support mathematical research. However, human expertise and intervention remain crucial in translating problems and interpreting results.
Explore the vital role of AI chips in driving the AI revolution, from semiconductors to processors: key players, market dynamics, and future implications.
A new AI language model method eliminates the need for matrix multiplication, significantly reducing power consumption and reliance on GPUs
Original Article Title:
Researchers upend AI status quo by eliminating matrix multiplication in LLMs.
Source: Ars Technica
Date: 26 June 2024
Researchers from the University of California Santa Cruz, UC Davis, LuxiTech, and Soochow University have developed a new AI language model method that eliminates the need for matrix multiplication, significantly reducing power consumption and reliance on GPUs. This innovative approach, detailed in their preprint paper, could revolutionize the efficiency and accessibility of AI technology.
Key Points:
- New Methodology:
- Researchers developed a custom language model using ternary values and a MatMul-free Linear Gated Recurrent Unit (MLGRU).
- This model can run efficiently on simpler hardware like FPGA chips, reducing energy use compared to traditional GPU-dependent models.
- Performance and Efficiency:
- The MatMul-free model showed competitive performance with significantly lower power consumption and memory usage.
- Demonstrated a 61% reduction in memory consumption during training.
- A 1.3 billion parameter model ran at 23.8 tokens per second using only 13 watts of power on an FPGA chip.
- Comparison with Conventional Models:
- Compared to a Llama-2-style model, the new approach achieved similar performance with lower energy consumption.
- The model, while smaller in scale (up to 2.7 billion parameters), suggests potential for scaling up to match or exceed state-of-the-art models like GPT-4.
- Implications for AI Deployment:
- This method could make AI technology more accessible and sustainable, especially for deployment on resource-constrained hardware like smartphones.
- Potential to drastically reduce the environmental impact and operational costs of running AI systems.
Why This Matters: The development of AI language models that do not rely on matrix multiplication presents a significant advancement in the field. By reducing power consumption and the need for expensive GPUs, this innovation makes AI technology more accessible and sustainable. This can lead to broader deployment possibilities, including on devices with limited computational resources, such as smartphones. Additionally, the reduction in energy use addresses growing concerns about the environmental impact of large-scale AI operations.
Meta 3D Gen is a new state-of-the-art pipeline for text-to-3D asset generation
Original Article Title:
Meta 3D Gen
Source: Meta
Date: 2 July 2024
Meta 3D Gen is a new state-of-the-art pipeline for text-to-3D asset generation. It creates high-quality 3D shapes and textures in under a minute, supporting physically-based rendering (PBR) and generative retexturing. Integrating Meta 3D AssetGen and Meta 3D TextureGen, it achieves a win rate of 68% compared to single-stage models, outperforming industry baselines in prompt fidelity and visual quality for complex textual prompts.
Cerebras Systems has announced the release of Cerebras Inference, that claims to be the world’s fastest AI inference system
Original Article Title:
Cerebras CS-3: the world’s fastest and most scalable AI accelerator
Source: Cerebras
Date: 12 March 2024
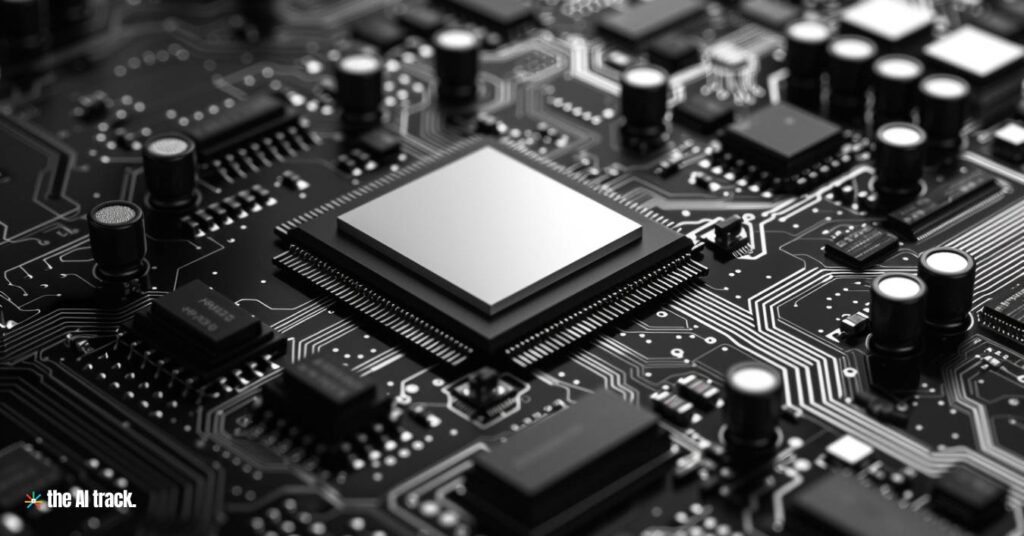
Cerebras Systems has announced the release of what it claims to be the world’s fastest AI inference system, known as Cerebras Inference.
This system is designed to significantly enhance the performance of large language models (LLMs) by leveraging their innovative hardware architecture.
Key Features and Performance
- Architecture: The Cerebras system utilizes the Wafer Scale Engine 3 (WSE-3), which is the largest AI chip currently available, allowing for significant memory integration directly onto the chip. This design minimizes the need for external memory interactions, which is a bottleneck in traditional GPU architectures.
- Speed: Cerebras Inference reportedly delivers 1,800 tokens per second for the Llama 3.1 8B model and 450 tokens per second for the Llama 3.1 70B model. This performance is stated to be 20 times faster than comparable NVIDIA GPU-based solutions, making it a substantial advancement in AI inference speed.
- Accuracy: The system maintains high accuracy by operating in a native 16-bit precision throughout the inference process. This is significant because many competing systems often sacrifice accuracy for increased speed.
- Unprecedented Power: The CS-3 boasts over 4 trillion transistors, 57x more than the largest GPU. The CS-3 system can be configured to link up to 2048 units, enabling the creation of supercomputers capable of handling extremely large AI models, potentially up to 24 trillion parameters. This scalability is essential for training next-generation models that require vast computational resources.
- Innovation in AI Supercomputing: The Condor Galaxy 3 supercomputer, powered by 64 CS-3 systems, delivers 8 exaflops and simplifies AI development by functioning as a single logical device.
- Cost-Efficient Inference: Collaboration with Qualcomm ensures that models trained on CS-3 achieve up to 10x faster inference.
- Pricing: Cerebras Inference is priced competitively, starting at 10 cents per million tokens for the Llama 3.1 8B model and 60 cents per million tokens for the Llama 3.1 70B model. This pricing structure provides a 100x higher price-performance ratio compared to traditional GPU solutions.
Why This Matters: The CS-3 redefines the limits of AI development, allowing researchers to scale AI models faster and more efficiently, setting new benchmarks in the industry.
NVIDIA has unveiled its new massive AI model, designed to take on GPT-4. This open model aims to revolutionize AI development. Read all about it.
Meta’s Transfusion model handles text and images in a single architecture.
Original Article Title:
Meta’s Transfusion model handles text and images in a single architecture.
Source: VentureBeat
Date: 30 August 2024
Meta, in collaboration with the University of Southern California, introduces the Transfusion model, which processes text and images simultaneously using a single architecture. Unlike traditional methods that use separate architectures or quantize images into tokens, Transfusion combines language modeling and diffusion for more accurate text-to-image and image-to-text generation. This breakthrough outperforms models like Chameleon and DALL-E 2 with less computational cost, paving the way for multi-modal AI applications.
Google DeepMind Introduces Self-Correction via Reinforcement Learning (SCoRe) to Enhance AI Models
Original Article Title:
Training Language Models to Self-Correct via Reinforcement Learning
Source: Cornell University – ArXiv
Date: 19 September 2024
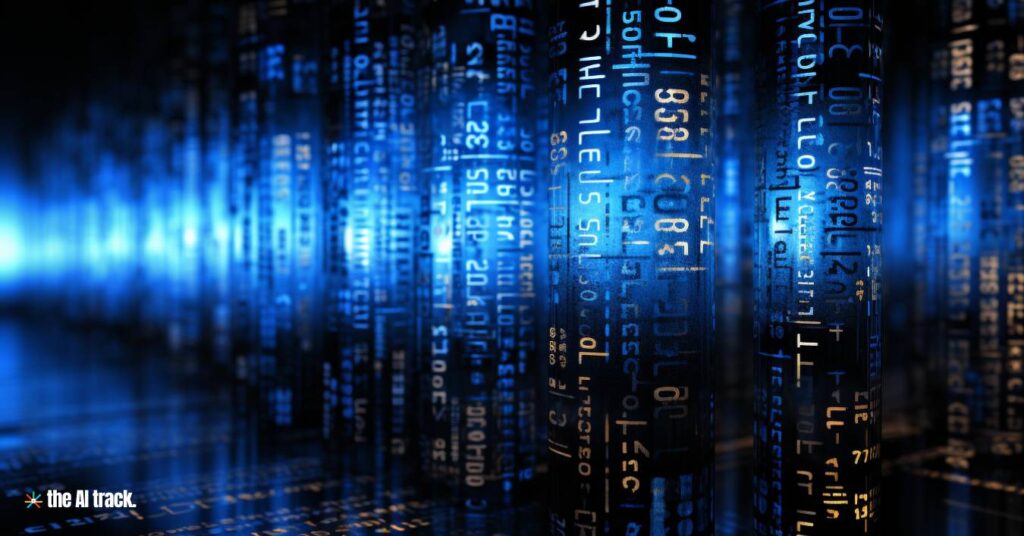
Key Takeaway:
Google DeepMind has developed a new AI method called Self-Correction via Reinforcement Learning (SCoRe), which significantly enhances large language models’ (LLMs) accuracy in complex mathematical and coding tasks by enabling self-correction without external supervision.
Key Points:
Problem with Current LLMs:
Large language models struggle with self-correction, often generating incomplete or incorrect answers in complex tasks like mathematics and coding. They lack the ability to consistently detect and correct their mistakes, particularly in multi-step reasoning scenarios, leading to cumulative errors.
Existing Limitations:
Current self-correction techniques rely on supervised fine-tuning or multiple models, which are computationally expensive, amplify biases, and often fail to adapt effectively to real-world queries. These approaches require external data or verifier models, limiting their practicality.
Introduction of SCoRe:
Google DeepMind’s SCoRe method uses reinforcement learning to enable LLMs to self-correct based on their responses, without needing external supervision. SCoRe’s multi-turn reinforcement learning trains models to learn from their own data, improving their ability to handle real-world tasks.
Two-Stage Process:
- Initialization Training: The model learns an initial correction strategy, avoiding minor, ineffective edits.
- Reinforcement Learning: The model is trained to refine its corrections in a multi-turn setup, with rewards shaping to emphasize substantial accuracy improvements. This training ensures the model focuses on meaningful changes rather than superficial adjustments.
Performance Improvements:
SCoRe demonstrated significant performance boosts in tests with Gemini 1.0 Pro and 1.5 Flash models:
- Achieved a 15.6% improvement in mathematical reasoning tasks and a 9.1% improvement in coding tasks.
- Increased first-attempt accuracy to 60.0% and second-attempt accuracy to 64.4%, showcasing effective self-revision capabilities.
- Reduced incorrect-to-correct answer changes in subsequent attempts, addressing a common issue in previous methods.
Impact on AI Development:
SCoRe represents a major shift in LLM training, moving away from dependency on external supervision and addressing data mismatch issues. The approach enhances the reliability of LLMs in practical applications, particularly in areas requiring complex, multi-step reasoning.
Why This Matters:
SCoRe’s self-correction capability offers a breakthrough in making AI models more accurate and autonomous in complex tasks, paving the way for more reliable applications in real-world scenarios such as coding, mathematics, and beyond.
GameNGen: Google’s Breakthrough AI Real-Time Gaming
Original Article Title:
Diffusion Models Are Real-Time Game Engines
Source: Google
Date: 27 August 2024
🚀 Google has developed GameNGen, the world’s first AI-powered game engine that can simulate real-time gameplay with astonishing accuracy. Using neural models, GameNGen can predict the next frame of a complex game like DOOM at 20 FPS, matching human-perceived quality. The game engine operates entirely on AI, making it a revolutionary step forward for real-time game engines. 🕹️
Here’s how it works:
- An RL agent learns to play the game, recording its gameplay.
- A diffusion model generates the next frame based on past actions and frames.
🎮 Why this matters:
- High-quality simulations of complex environments.
- Potential to reshape how games are built—AI could replace traditional, manually programmed game engines.
- Opens doors for more interactive and adaptive gaming experiences.
🤖 What’s next? Neural game engines like GameNGen could pave the way for automatically generated games—similar to how images are generated by AI today. A new paradigm is on the horizon for gaming!
Our AI Books hub offers comprehensive guides to essential AI literature, providing key insights and actionable takeaways. Explore the transformative potential of AI through expertly summarized books.
Brain on a Chip: Boosting AI Efficiency by 460x!
Original Article Title:
New brain-on-a-chip platform to deliver 460x efficiency boost for AI tasks
Source: NetworkWorld
Date: 13 September 2024
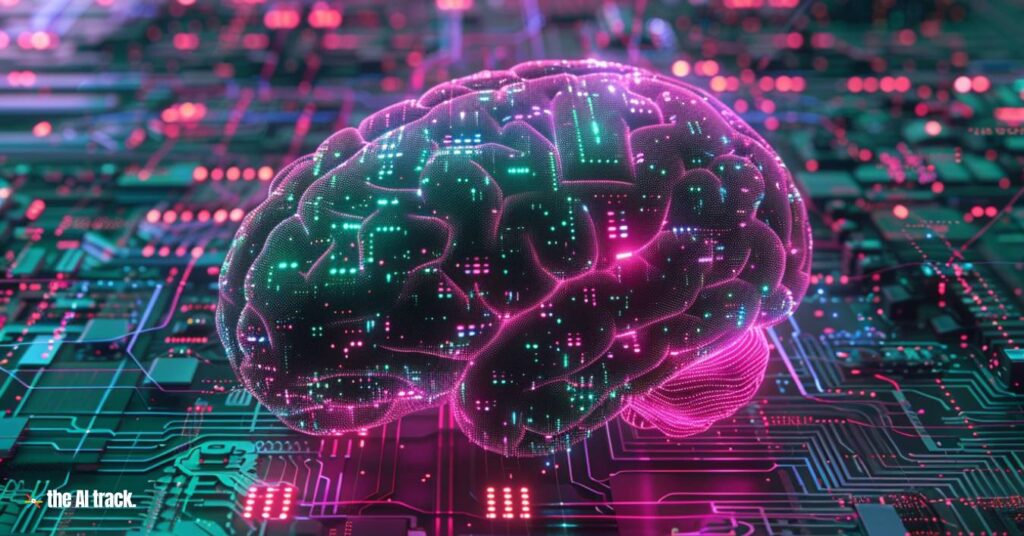
The Indian Institute of Science (IISc) has developed a groundbreaking neuromorphic computing platform that dramatically enhances energy efficiency and processing speed for AI tasks. This brain-inspired technology, capable of handling 16,500 conductance states, is set to revolutionize AI hardware by working alongside existing systems to achieve unprecedented performance improvements.
Key Points:
Neuromorphic Computing Innovation:
The new platform developed by IISc mimics the human brain’s structure and processes, allowing it to store and process data across 16,500 conductance states in a molecular film. This is a significant leap from traditional digital systems, which are limited to binary states (on and off).
Efficiency and Speed Improvements:
The platform reduces the steps required for core AI operations, such as vector-matrix multiplication, from n² steps to just one, greatly boosting speed and energy efficiency. It delivers 4.1 TOPS/W, making it 460 times more efficient than an 18-core Haswell CPU and 220 times more efficient than an Nvidia K80 GPU, commonly used in AI workloads.
Potential Applications:
The platform’s ability to process complex data with high precision (14-bit accuracy) makes it ideal for advanced AI tasks, including machine learning, data analysis, and robotics. It is also expected to enhance brain-computer interfaces by accurately decoding specific brain patterns related to movements or mental states.
Integration with Existing AI Hardware:
Unlike many emerging technologies, IISc’s neuromorphic platform is designed to work alongside existing AI systems rather than replace them. It is particularly suited to offloading repetitive tasks like matrix multiplication, complementing the strengths of GPUs and TPUs to create faster, more efficient AI models.
Developing Indigenous Neuromorphic Chips:
IISc is advancing towards creating a fully indigenous neuromorphic chip supported by India’s Ministry of Electronics and Information Technology. This effort covers the complete development cycle from materials to systems, positioning the platform as a crucial home-grown technology.
Demonstrated Capabilities:
The team has already showcased the platform’s power by recreating NASA’s “Pillars of Creation” image from the James Webb Space Telescope on a tabletop computer, achieving results that would traditionally require a supercomputer, but with much less time and energy.
Impact on Future AI Hardware:
As AI tasks become increasingly demanding, traditional silicon-based processors are reaching their limits. IISc’s platform offers a new path forward, leveraging brain-inspired analog computing to deliver faster, more energy-efficient solutions, potentially transforming industries such as cloud computing and autonomous systems.
Why This Matters:
IISc’s neuromorphic platform represents a significant technological breakthrough in AI hardware, offering vast improvements in energy efficiency and computational speed. By integrating this new technology with existing AI systems, the platform has the potential to drive future advancements in AI, making high-performance AI applications more accessible and sustainable.
Introducing OneGen: A Revolutionary AI Framework Combining Retrieval and Generation
Original Article Title:
OneGen: Efficient One-Pass Unified Generation and Retrieval for LLMs
Source: Cornell University – ArXiv
Date: 8 September 2024
Key Takeaway:
OneGen is a novel AI framework developed by researchers from Zhejiang University that integrates retrieval and generation tasks within a single Large Language Model (LLM). This unified approach significantly reduces computational overhead and improves efficiency, making LLMs better suited for real-time applications that require both information retrieval and text generation.
Key Points:
Current Challenges in LLMs:
Traditional LLMs excel in generating text but struggle with retrieval tasks, which involve fetching relevant data before generating responses. Existing models often separate these tasks, leading to increased computational complexity, slower inference times, and higher error rates, especially in complex queries like question-answering or multi-turn dialogues.
Limitations of Previous Methods:
Approaches like Retrieval-Augmented Generation (RAG) use separate models for retrieval and generation, which creates inefficiencies. These models operate in distinct representational spaces, requiring additional computational steps that slow down the process and increase the risk of errors in complex tasks.
Introduction of OneGen:
OneGen addresses these limitations by unifying retrieval and generation within a single forward pass of an LLM. It incorporates autoregressive retrieval tokens directly into the model, allowing retrieval and generation to occur simultaneously. This approach eliminates the need for separate models and multiple forward passes, enhancing speed and reducing computational load.
Technical Foundation:
OneGen augments the LLM’s vocabulary with special retrieval tokens generated during text generation. These tokens are fine-tuned using contrastive learning, ensuring that retrieval processes integrate smoothly with generation tasks. The unified training method enables the model to handle both tasks seamlessly in real-time.
Performance and Evaluation:
OneGen was tested on datasets like HotpotQA and TriviaQA for question-answering and Wikipedia-based datasets for entity linking. The framework outperformed existing models, such as Self-RAG and GRIT, showing a 3.2-point accuracy improvement in entity-linking and a 3.3-point increase in F1 scores in multi-hop QA tasks. This highlights OneGen’s efficiency in managing both retrieval and generation without compromising performance.
Implications and Future Potential:
By merging retrieval and generation processes into a single system, OneGen streamlines LLM operations, making them faster and more accurate for real-world applications. This breakthrough could revolutionize how LLMs handle complex tasks that demand real-time retrieval and generation, expanding their applicability in high-speed, high-accuracy environments.
Why This Matters:
OneGen’s unified framework marks a significant leap forward in AI, addressing longstanding inefficiencies in LLMs. Its ability to handle both retrieval and generation within a single pass not only boosts performance but also paves the way for more advanced, real-time AI applications that rely on accurate, context-driven information generation.
OpenAI introduces ‘Swarm’: A multi-agent collaboration framework
Original Article Title:
Swarm – some initial insights
Source: OpenAI Developer Forum
Date: 12 October 2024
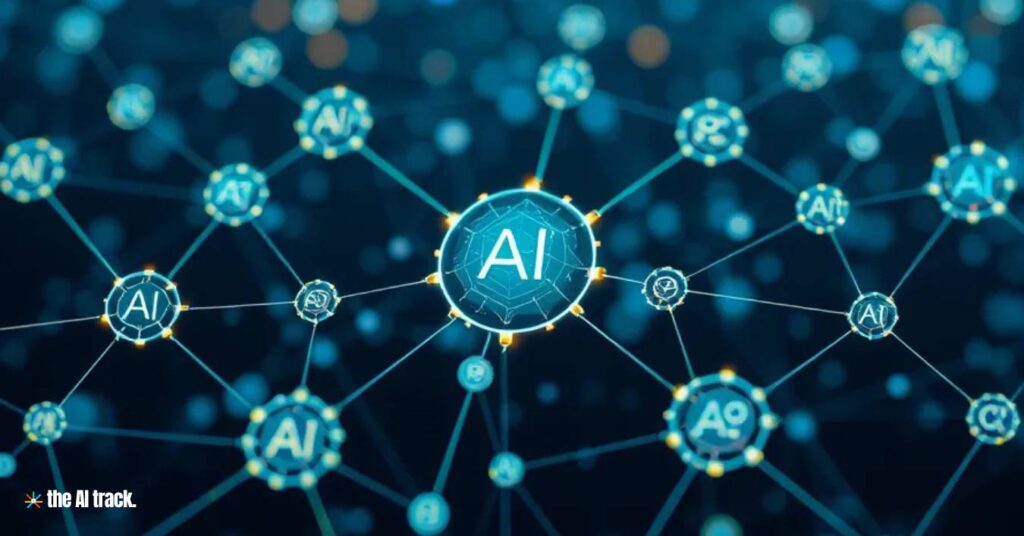
Key Takeaway:
OpenAI’s experimental “Swarm” framework focuses on coordinating networks of autonomous AI agents to tackle complex tasks, creating significant potential for enterprise automation.
Key Points:
- Swarm Functionality: Multi-agent systems collaborate autonomously, reducing human involvement in complex processes.
- Enterprise Application: Could revolutionize business operations by automating multi-departmental tasks.
- AI Community Debate: Concerns include security, ethical implications, and job displacement due to increased automation.
Why This Matters:
Swarm could transform industries through AI-driven collaboration but requires careful consideration of societal impacts.
Everything you need to know about the AI chip business and the geopolitical factors shaping the global race for AI (chip) dominance
Altera uses GPT-4o to build a new area of human collaboration
Original Article Title:
Altera uses GPT-4o to build a new area of human collaboration
Source: OpenAI
Date: 7 October 2024
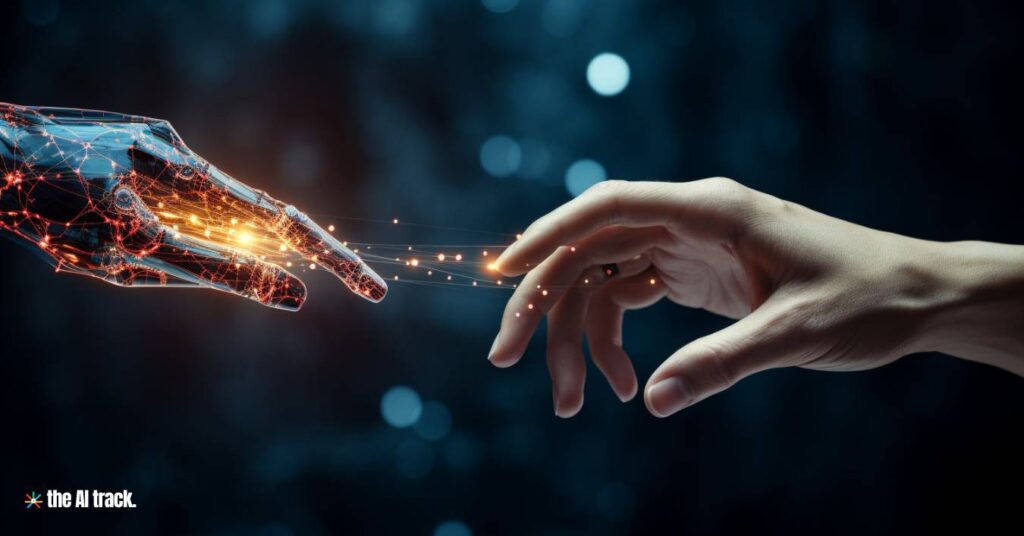
Key Takeaway:
Altera is leveraging OpenAI’s GPT-4 to develop digital humans capable of cognitive and emotional interactions, focusing on long-term autonomy and collaboration in virtual environments. These AI agents can operate for hours autonomously, perform complex tasks, and even play Minecraft with users.
Key Points:
- Founding Vision: Dr. Robert Yang, former MIT professor, founded Altera in 2023 to develop “digital humans” that collaborate with humans and interact emotionally.
- AI Integration: Utilizing OpenAI’s GPT-4 and neural model simulations, Altera addresses data degradation in AI for long-term performance.
- Minecraft Agents: Altera’s first AI agents can play Minecraft, with potential future applications in multi-agent worlds, productivity, and more.
Why This Matters:
Altera’s advancements represent a leap in AI-human interaction, potentially revolutionizing virtual collaboration, gaming, and productivity tools by integrating long-lasting, emotionally responsive AI agents.
Transforming 2D into 3D with Apple’s Depth Pro
Original Article Title:
Depth Pro: Sharp Monocular Metric Depth in Less Than a Second
Source: Github
Date: 4 October 2024
Key Takeaway: Apple’s AI research team has developed Depth Pro, a new AI-powered model that can generate detailed, high-resolution 3D depth maps from single 2D images. This breakthrough technology achieves these results in just 0.3 seconds without the need for traditional camera metadata, making it a significant innovation for fields like augmented reality (AR) and self-driving cars, where spatial awareness and real-time depth perception are crucial.
Key Points:
- Depth Pro Overview:
- Depth Pro is a monocular depth estimation model that generates sharp, high-frequency, and metric depth maps from a single 2D image. The depth maps provide absolute scale without relying on camera intrinsics or metadata.
- The model runs extremely fast, producing a 2.25-megapixel depth map in 0.3 seconds on a standard GPU, which enables real-time applications in various sectors like AR and autonomous vehicles.
- Technical Contributions:
- Depth Pro integrates multi-scale vision transformers for dense prediction, ensuring that depth maps are sharp and include fine details.
- A unique training protocol uses both real and synthetic datasets, enhancing metric accuracy and precise boundary tracing.
- The model includes state-of-the-art focal length estimation from a single image, which is crucial for accurate depth mapping without metadata.
- Use Cases and Applications:
- Augmented Reality (AR): With real-time depth mapping, AR experiences can become more immersive and spatially aware, blending digital objects seamlessly into real-world environments.
- Self-driving Cars: Enhanced depth perception enables better object detection, obstacle avoidance, and route planning.
- Other applications include robotics, 3D modeling, and cinematography, where accurate 3D scene understanding is essential.
- Open-Source Implementation:
- The model has been released on GitHub under an open-source license, allowing developers and researchers to integrate it into various applications. The GitHub repository provides tools to run the model from the command line or through Python scripts.
- Researchers can also evaluate the model using boundary accuracy metrics, which are important for fine-tuning and testing its performance.
- Real-Time Performance:
- The model’s ability to generate high-resolution depth maps in under a second is a significant improvement over traditional depth estimation techniques, which often require multiple inputs or longer processing times.
- This innovation could lead to significant advancements in real-time 3D spatial awareness, critical for a variety of industries like autonomous systems and AR applications.
Why This Matters:
The release of Depth Pro is a major step forward in the field of computer vision, particularly for applications that require rapid and accurate depth estimation. By leveraging AI and vision transformers, the model overcomes the limitations of traditional depth mapping methods and opens the door to new possibilities in areas like autonomous driving, augmented reality, and robotics. Its fast performance and open-source availability make it accessible for widespread use and further development.