AI Breakthroughs in Environment - At a Glance

AI is playing a crucial role in monitoring and protecting our planet. This section explores the key AI innovations in envornment – innovations that are helping us address environmental challenges and protect wildlife.
Each section offers insights into how AI is transforming different sectors, providing a comprehensive view of its impact across a wide range of disciplines.
The Impact of Artificial Intelligence on the Environment: A Tale of Eco-Innovation and Resource Consumption
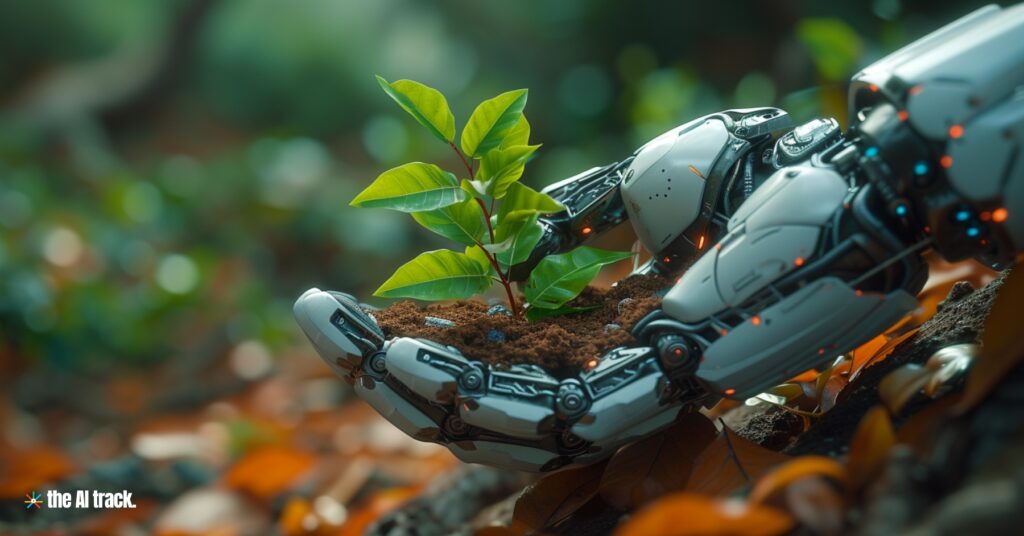
Investigating the transformative effects of artificial intelligence in addressing climate change and environmental sustainability, this article covers AI’s applications in reducing emissions, enhancing agricultural efficiency, and bolstering conservation initiatives, alongside discussing its own environmental footprint.
AI revolutionizes climate forecasting by speeding up modeling and reducing energy costs
Original Article Title:
How AI is improving climate forecasts
Source: Nature
Date: Date: 26 March 2024
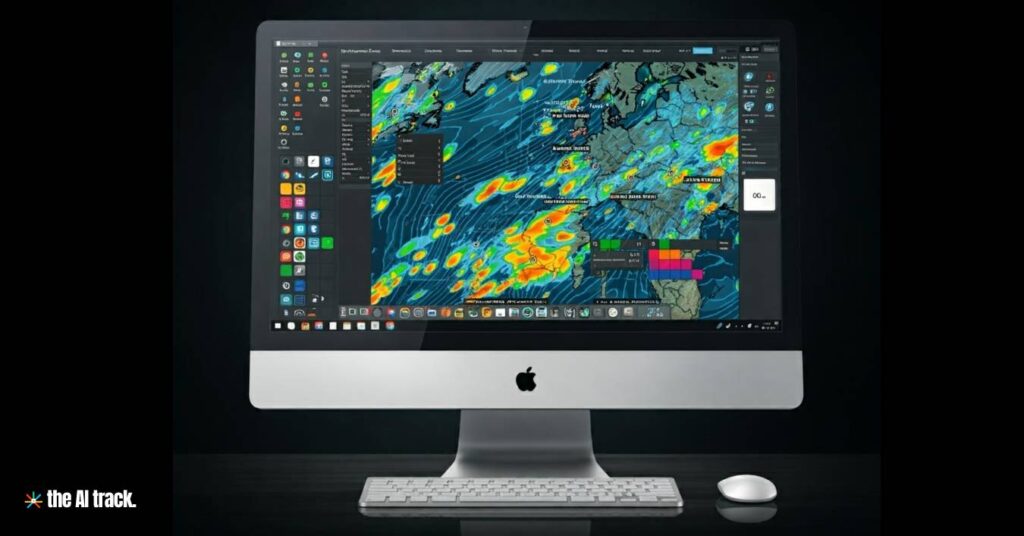
Machine learning and artificial intelligence (AI) are revolutionizing climate forecasting by speeding up modeling, reducing energy costs, and potentially enhancing accuracy.
Summary
- Climate scientist Tapio Schneider from Caltech praises the transformative impact of machine learning and AI on climate modeling, making it faster, more satisfying, and capable of yielding better solutions.
- Conventional climate models, manually built by scientists, are computationally intensive, time-consuming, and struggle to simulate small-scale processes accurately.
- Machine learning, particularly through emulators, offers a promising solution by producing results akin to conventional models without extensive mathematical calculations.
- Emulators like QuickClim can significantly accelerate climate projections, enabling the exploration of multiple scenarios quickly.
- Another approach involves using machine learning to power the core of climate models, creating ‘foundation’ models like ClimaX, which can be fine-tuned for various climate-related tasks.
- While these advancements show promise, challenges remain in convincing stakeholders of their reliability and interpretability, especially concerning the ‘black box’ nature of AI models.
- Hybrid models, combining machine learning with physics-based techniques, aim to address specific shortcomings in conventional models, such as predicting small-scale processes like snow cover.
- Efforts like the Climate Modeling Alliance (CliMA) and projects by NASA and the European Commission aim to develop comprehensive digital models of Earth’s systems powered by AI.
- The ultimate goal is to achieve highly accurate and speedy simulations of all aspects of weather and climate, down to kilometer scales, but this target is still a work in progress.
Researchers have made significant progress in decoding the complex communication system of sperm whales, revealing a sophisticated "phonetic alphabet" used in their vocalizations
Original Article Title:
Exploring the mysterious alphabet of sperm whales
Source: MIT News
Date: 7 May 2024
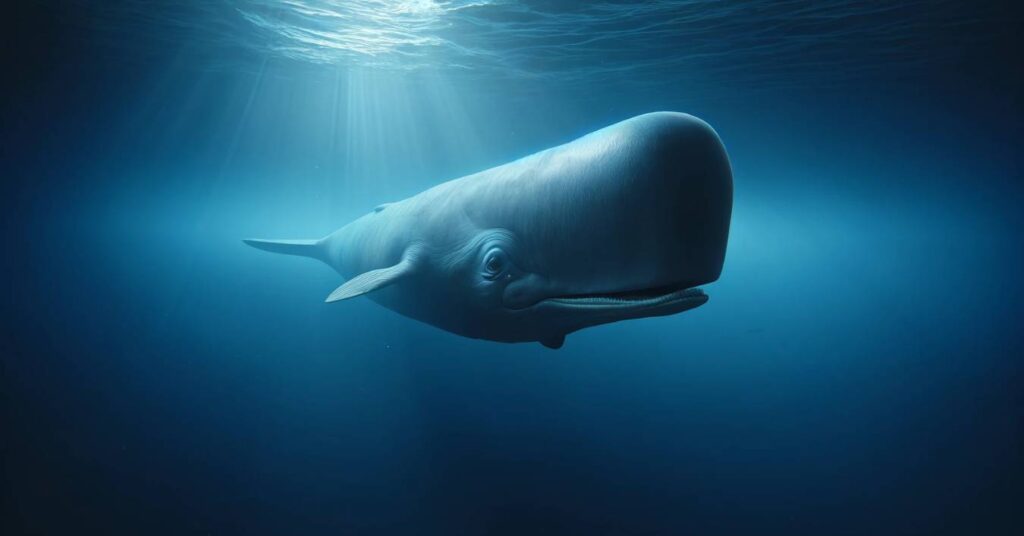
Researchers at MIT CSAIL and Project CETI have made significant progress in decoding the complex communication system of sperm whales, revealing a sophisticated “phonetic alphabet” used in their vocalizations. This study highlights the whales’ ability to produce varied codas that change based on social context, suggesting a level of communicative complexity thought to be unique to humans. This breakthrough enhances our understanding of animal languages and opens new avenues for interdisciplinary research.
Nvidia has developed Earth-2, a digital twin of the planet, to enhance weather forecasting.
Original Article Title:
Nvidia has virtually recreated the entire planet — and now it wants to use its digital twin to crack weather forecasting for good
Source: TechRadar
Date: 18 March 2024
Nvidia has developed Earth-2, a digital twin of the planet, to enhance weather forecasting. This model aims to provide faster and more accurate predictions by leveraging advanced AI and high-resolution simulations.
Key Points:
- Earth-2 uses Nvidia’s CorrDiff AI model, offering 12.5 times higher resolution images 1,000 times faster and 3,000 times more energy-efficiently than current models.
- Applications include early typhoon detection and evacuation planning.
- Utilized by global organizations, including the Taiwan Central Weather Administration.
Why This Matters: Improving weather forecasts can significantly reduce economic losses and enhance disaster preparedness, addressing the growing impact of climate change.
AI-powered forecasting models are being explored to improve the accuracy and timeliness of storm predictions.
Original Article Title:
No physics? No problem. AI weather forecasting is already making huge strides
Source: Ars Technica
Date: 3 June 2024
Key Takeaway: As a potentially severe hurricane season approaches, AI-powered forecasting models are being explored to improve the accuracy and timeliness of storm predictions.
Key Points:
- AI Capabilities: AI models offer more precise forecasting by analyzing vast datasets quickly.
- Potential Benefits: Enhanced predictions could lead to better preparedness and response strategies, potentially saving lives and reducing damage.
- Challenges: AI models still face limitations in accurately predicting the complex dynamics of hurricanes.
Why This Matters: Improved forecasting through AI could revolutionize how we prepare for and respond to natural disasters.
Saving bees with AI!
Original Article Title:
Xailient Protects Australian Bees with Solar-Powered Computer Vision AI
Source: Xailient
Date: 9 March 2022
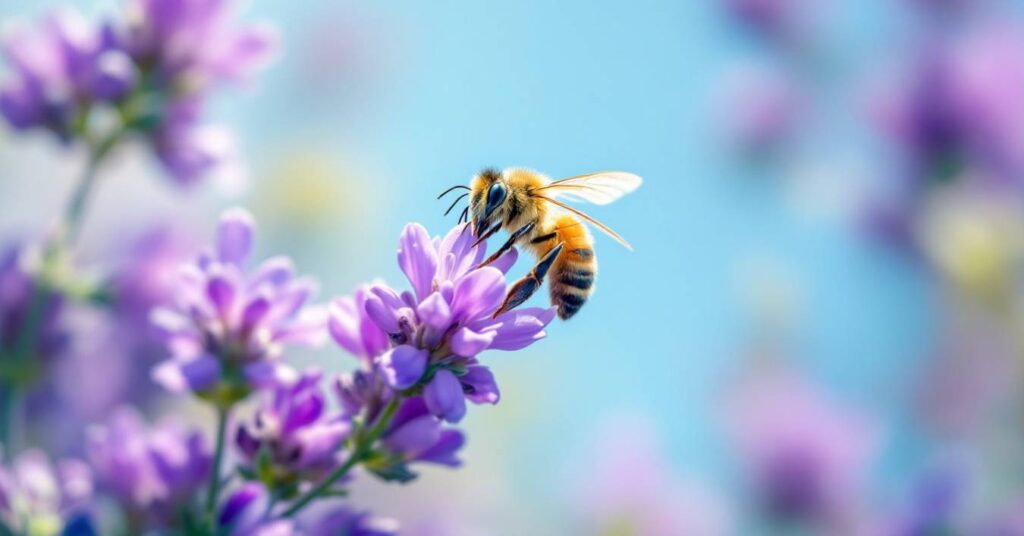
Key Takeaway:
Xailient is leveraging solar-powered computer vision AI to protect bees, particularly by monitoring the Varroa mite, a significant threat to bee populations. This technology allows continuous, real-time surveillance of beehives, ensuring better management and protection of bees.
Key Points:
Technology Implementation:
Xailient’s AI is deployed using solar-powered camera systems integrated with edge computing, enabling 24/7, year-round monitoring. This system operates directly at the hive, ensuring real-time data processing without the need for constant human intervention.
Focus on Varroa Mite:
The primary application of this technology is to monitor the Varroa mite, a parasitic mite that devastates bee colonies. By detecting these mites early, beekeepers can take timely action to protect their hives.
Environmental Impact:
The use of solar power minimizes the environmental footprint of the monitoring system, aligning with sustainable practices while ensuring uninterrupted operation in remote locations.
Deployment Location:
This technology has been specifically implemented in Australia, addressing local beekeeping challenges and contributing to the global effort to protect pollinators.
Real-time Monitoring:
Unlike traditional methods that involve manual checks, Xailient’s system allows continuous, non-invasive monitoring. This reduces the stress on bees and provides more accurate data for beekeepers.
Why This Matters: The decline of bee populations poses a significant threat to global agriculture due to their crucial role in pollination. By integrating advanced AI with sustainable energy sources, Xailient’s solution provides an innovative approach to protecting bees, potentially mitigating the risk of crop failures and preserving biodiversity.
The EU has launched an AI-powered digital twin of the Earth, aiming to predict climate change
Original Article Title:
EU launches AI-powered ‘digital twin’ of the Earth
Source: The Next Web
Date: 10 June 2024
European Union’s (EU) is launching a new AI-powered model to predict climate change. The model is called Destination Earth (DestinE). DestinE uses data from satellites, ground sensors, and other sources to simulate the Earth’s climate. The goal is to improve the accuracy of climate predictions. The EU hopes to use DestinE to predict extreme weather events and other climate issues.
Key Takeaway: The European Union has launched a new AI-powered model to predict climate change.
Key Points:
- The model is called Destination Earth (DestinE).
- DestinE uses data from satellites, ground sensors, and other sources to simulate the Earth’s climate.
- The goal is to improve the accuracy of climate predictions.
- The EU hopes to use DestinE to predict extreme weather events and other climate issues.
Why This Matters: Climate change is a serious threat to the planet. DestinE could help us to better understand climate change and make better decisions about how to mitigate its effects.
NASA and IBM Research have developed the Prithvi-weather-climate AI model for weather and climate predictions
Original Article Title:
NASA, IBM Research to Release New AI Model for Weather, Climate
Source: NASA Science
Date: 22 May 2024
NASA and IBM Research have developed the Prithvi-weather-climate AI model, enhancing weather and climate predictions through advanced AI, improving spatial resolution, and enabling better detection and forecasting of severe weather patterns.
Key Points:
- The model leverages NASA’s data, particularly from the MERRA-2 dataset.
- It improves predictions for severe weather, localized forecasts, and regional climate simulations.
- Developed in collaboration with IBM Research, Oak Ridge National Laboratory, and others.
- Scheduled for release on Hugging Face, promoting open science principles.
Why This Matters: Improving weather and climate predictions helps inform decisions for preparation, response, and mitigation, addressing urgent climate challenges.
Explore our AI Books hub for detailed summaries of the most influential AI books. Discover key insights, actionable takeaways, and viral quotes.
Google has introduced a new AI tool to monitor coral reef health
Original Article Title:
A new AI tool to help monitor coral reef health.
Source: Google – The Keyword
Date: 6 June 2024
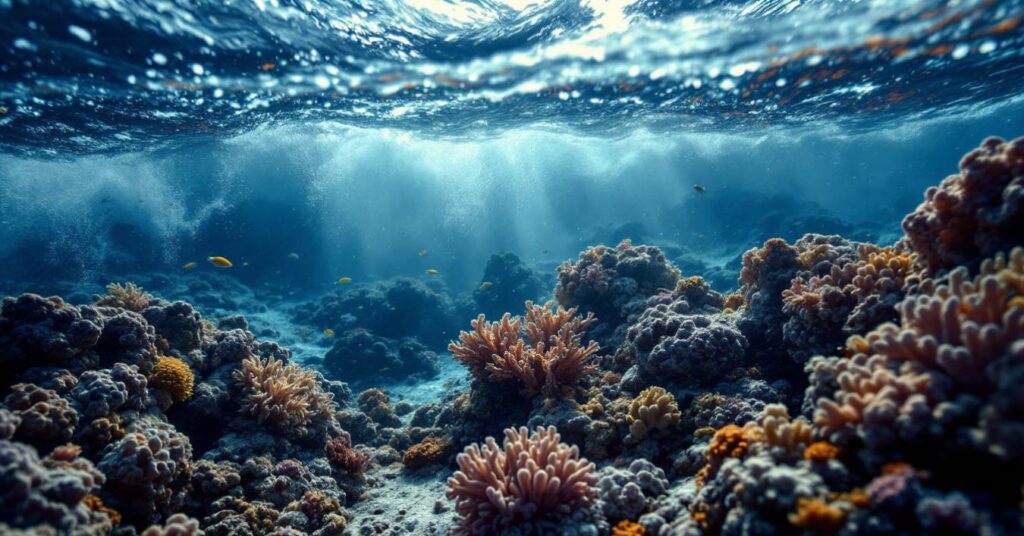
Google has introduced a new AI tool to monitor coral reef health. This technology leverages machine learning to analyze underwater images, helping researchers track changes in coral ecosystems more effectively. By providing detailed insights, the tool aims to support conservation efforts and promote the health of coral reefs globally.
California's SharkEye project utilizes AI for real-time shark detection, enhancing beach safety
Original Article Title:
Sharks are congregating at a California beach. AI is trying to keep swimmers safe
Source: CNN Science
Date: 29 July 2024
California’s SharkEye project utilizes AI for real-time shark detection, enhancing beach safety by identifying and tracking sharks along the coast.
Key Points:
- Technology: Uses drones and AI to detect sharks, providing data to lifeguards and researchers.
- Safety: Improves swimmer and surfer safety by alerting authorities to shark presence.
- Data Collection: Aids in marine research by tracking shark movements and behaviors.
Why This Matters: AI-driven shark detection increases beach safety and contributes valuable data for marine conservation efforts.
Microsoft's AI model, Aurora is the first to predict global air pollution and weather in less than a minute
Original Article Title:
Superfast Microsoft AI is first to predict air pollution for the whole world
Source: Nature
Date: 4 June 2024
Microsoft’s AI model, Aurora, represents a significant advancement in atmospheric science by being the first to predict global air pollution and weather in less than a minute. This innovation marks a leap in computational efficiency and accuracy in environmental forecasting.
Key Points:
- AI Development: Microsoft’s Aurora is an AI model that forecasts global weather and air pollution with unprecedented speed and accuracy.
- Pollutant Prediction: Aurora predicts six major air pollutants (CO, NO, NO₂, SO₂, O₃, and particulate matter) for five days, crucial for health risk management.
- Efficiency: Aurora operates at a much lower computational cost compared to traditional models, enhancing accessibility and scalability.
- Training Data: Trained on over a million hours of weather and climate data, Aurora offers high-quality predictions similar to conventional models.
- Comparison and Future Research: Aurora’s performance suggests potential superiority over other AI models like Google DeepMind’s GraphCast, though further research is needed.
Why This Matters: Aurora’s ability to predict air pollution and weather globally within seconds is crucial for timely decision-making in public health and environmental protection. Efficient AI models like Aurora can help mitigate the adverse health impacts of air pollution and improve climate resilience, potentially leading to better policy decisions and preventive measures.
The University of Southampton is using AI and drones to search for the endangered female Encephalartos woodii
Original Article Title:
AI helping find ‘world’s loneliest plant’ a partner
Source: BBC
Date: 29 May 2024
Artificial intelligence, in conjunction with drone technology, is being employed by the University of Southampton to locate a female partner for the world’s loneliest plant, Encephalartos woodii (E. woodii), in South Africa’s Ngoye Forest. This ancient species, which predates dinosaurs, consists solely of male clones, preventing natural reproduction. The project, led by Dr. Laura Cinti, leverages AI to analyze drone footage of the forest canopy, hoping to discover a female E. woodii and enable the species to reproduce naturally.
NASA's SPoRT program used a new AI-powered lightning prediction tool at the Rock the South music festival to forecast lightning up to 15 minutes in advance
Original Article Title:
SPoRT’s Lightning Prediction Tool Provides Critical Weather Forecasting Support at Rock the South
Source: NASA
Date: 9 August 2024
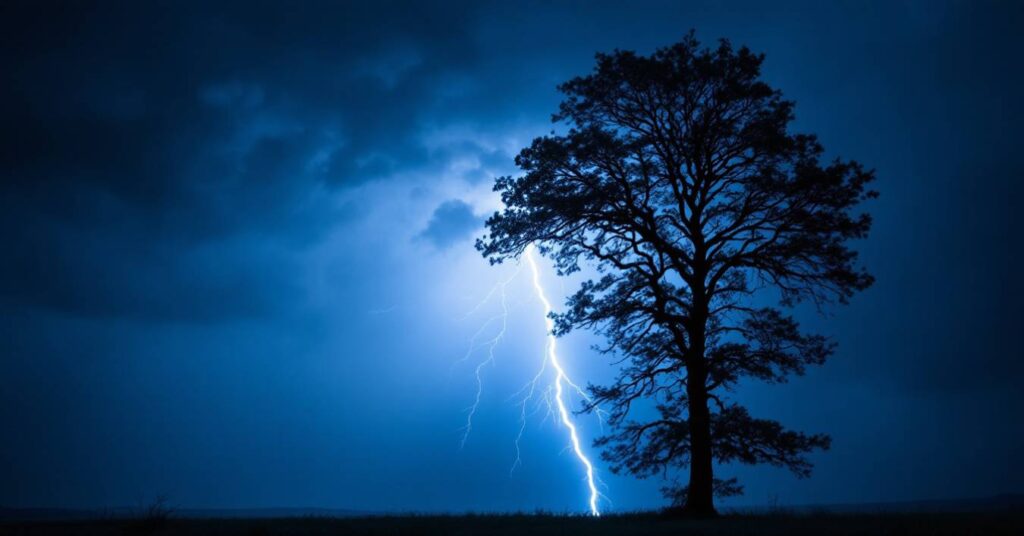
NASA’s SPoRT program used a new AI-powered lightning prediction tool at the Rock the South music festival to forecast lightning up to 15 minutes in advance. This tool provided critical weather support, helping to ensure the safety of over 35,000 attendees by predicting potential lightning strikes and informing event organizers to take precautionary measures. The tool aims to enhance public safety by providing more lead time for sheltering.
The NeuralGCM Model integrates a differentiable solver for atmospheric dynamics with machine-learning components, achieving competitive accuracy in weather and climate forecasts
Original Article Title:
Neural general circulation models for weather and climate
Source: Nature
Date: July 22, 2024
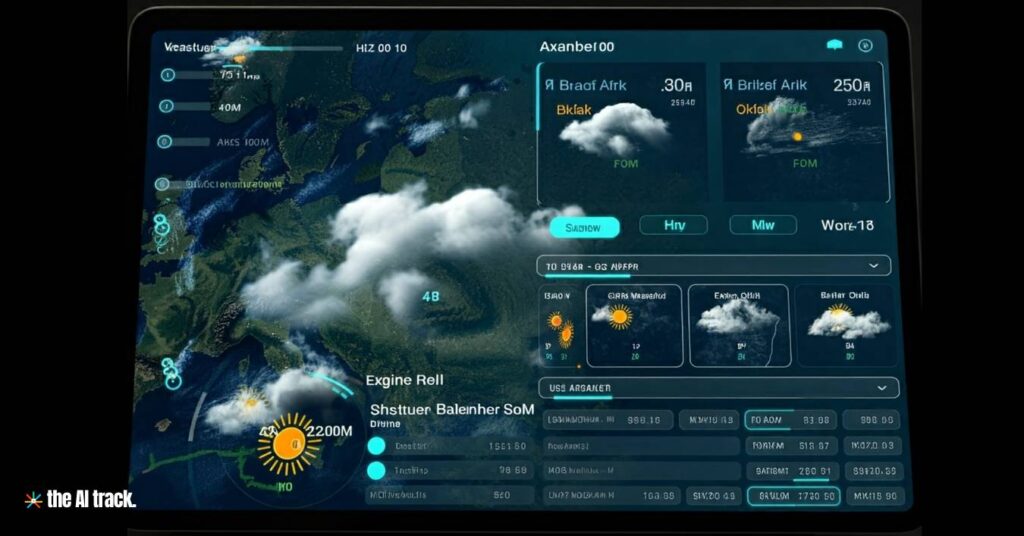
The Neural General Circulation Model (NeuralGCM) integrates a differentiable solver for atmospheric dynamics with machine-learning components, achieving competitive accuracy in weather and climate forecasts with significant computational efficiency.
Key Points:
- Innovative Hybrid Model: NeuralGCM combines traditional physics-based modeling with neural networks, resulting in high accuracy for both short-term weather and long-term climate forecasts.
- Enhanced Performance: NeuralGCM matches or surpasses the European Centre for Medium-Range Weather Forecasts (ECMWF) for 1- to 15-day weather predictions and tracks climate metrics accurately over decades.
- Computational Efficiency: The model offers substantial computational savings compared to traditional general circulation models (GCMs), making it feasible for widespread use.
- Emergent Phenomena: NeuralGCM successfully simulates critical atmospheric phenomena like tropical cyclones and seasonal cycles with realistic frequency and trajectories.
- Stability and Adaptability: The model maintains stability for long-term simulations and adapts well to unseen weather data, showing potential for broader applications.
Why This Matters: NeuralGCM represents a significant advancement in climate and weather forecasting, offering a more efficient and accurate tool for understanding and predicting atmospheric dynamics, which is crucial for climate mitigation and adaptation strategies.
Google-inspired AI model improves Cape Canaveral space launch weather forecasts by 50%
Original Article Title:
Google-inspired AI model improves Cape Canaveral space launch weather forecasts by 50%
Source: Space
Date: 31 July 2024
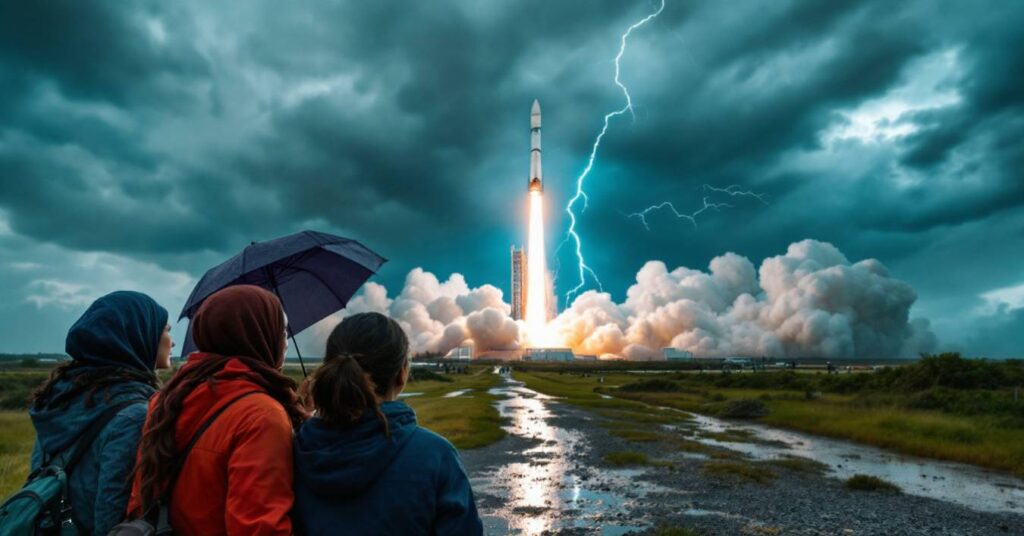
An AI model inspired by Google’s language-processing technology has significantly improved weather forecasts for Cape Canaveral rocket launches, increasing accuracy by 50% and speeding up predictions.
Key Points:
- Improved Forecasting Accuracy: The AI model developed by Atmo AI enhances weather predictions for key metrics like wind, temperature, and humidity by up to 50%.
- Speed and Efficiency: The model runs up to 40,000 times faster than traditional weather models, producing forecasts in seconds instead of hours.
- Practical Applications: This technology aids Cape Canaveral’s 45th Weather Squadron in managing launch schedules more effectively, reducing disruptions caused by adverse weather.
Why This Matters: Enhanced weather forecasting using AI improves the reliability of rocket launches, ensuring safer and more efficient space missions.
AI Chases the Storm: New NVIDIA Research Boosts Weather Prediction, Climate Simulation
Original Article Title:
AI Chases the Storm: New NVIDIA Research Boosts Weather Prediction, Climate Simulation
Source: NVIDIA
Date: 19 August 2024
NVIDIA Research has introduced StormCast, a generative AI model poised to revolutionize mesoscale weather forecasting by offering unprecedented accuracy and fidelity in predicting atmospheric dynamics, which is crucial for disaster preparedness and mitigation.
Key Points:
- Mesoscale Weather Prediction Challenge: Mesoscale weather prediction, focusing on events larger than storms but smaller than cyclones, poses a significant challenge due to the complex interplay of atmospheric factors at this scale. Traditional numerical weather prediction (NWP) models often struggle to accurately predict these phenomena.
- StormCast’s Generative AI Approach: StormCast employs generative AI techniques, trained on extensive NOAA climate data from the central U.S., to emulate high-fidelity atmospheric dynamics and enable reliable weather prediction at the mesoscale.
- High-Fidelity Predictions: StormCast generates outputs showcasing physically realistic heat and moisture dynamics, and can predict over 100 variables like temperature, moisture, wind, and rainfall radar reflectivity at various altitudes.
- 3D Evolution of Storm Buoyancy: A groundbreaking capability of StormCast is its ability to demonstrate the realistic 3D evolution of a storm’s buoyancy, a feat previously unattained in AI weather simulation.
- Hourly Autoregressive Prediction: The model employs hourly autoregressive prediction, allowing it to generate future weather forecasts based on historical weather data, thereby improving prediction accuracy and resolution.
- Early Successes: Though still under development, StormCast has already demonstrated its potential in accurately predicting complex weather events such as thunderstorms and winter precipitation.
Why This Matters:
- Enhanced Disaster Preparedness: Accurate mesoscale weather prediction is essential for effective disaster planning and mitigation, potentially saving lives and reducing property damage.
- Advancement in Climate Research: StormCast’s ability to simulate realistic atmospheric dynamics could contribute to significant advancements in climate research and our understanding of weather patterns.
- AI’s Potential in Meteorology: This development highlights the transformative power of AI in addressing complex scientific challenges, opening doors for further innovation in meteorology and related fields.
NASA “Wildfire Digital Twin” Pioneers New AI Models and Streaming Data Techniques for Forecasting Fire and Smoke
Original Article Title:
NASA “Wildfire Digital Twin” Pioneers New AI Models and Streaming Data Techniques for Forecasting Fire and Smoke
Source: NASA Science
Date: 21 May 2024
NASA’s “Wildfire Digital Twin” project is an innovative tool designed to enhance wildfire management and forecasting. By utilizing advanced AI, machine learning, and real-time data from multiple sensors, this project aims to provide firefighters and researchers with highly accurate and timely predictions of wildfire behavior and its broader environmental impacts.
Key Points:
- Advanced AI and Machine Learning Integration: The Wildfire Digital Twin leverages AI and machine learning to predict wildfire paths and air pollution events in real-time. This system combines data from ground, airborne, and satellite sensors to create highly detailed models, offering a spatial resolution of 10 to 30 meters per pixel—a significant improvement over current global models, which operate at 10 kilometers per pixel.
- Rapid Response Capabilities: The new system can generate models within minutes, compared to the hours required by existing methods. This speed and accuracy are crucial for first responders and wildfire managers who need immediate, reliable information to make informed decisions during active fires.
- Portable and Accessible Technology: The Wildfire Digital Twin is designed to be used on a wide range of devices, including laptops and tablets, making it accessible even in remote areas without access to large computing resources. This adaptability ensures that critical data can be utilized in the field where it’s needed most.
- Global Wildfire Monitoring: Beyond immediate fire management, the project aims to provide a comprehensive tool for scientists studying global wildfire trends. This includes monitoring carbon emissions from boreal wildfires, which are increasing due to climate change and are responsible for a significant portion of global CO2 emissions.
- Collaboration and Field Testing: The project, led by Milton Halem at the University of Maryland, Baltimore County, involves collaboration with over 20 researchers from six universities. The team has already conducted field tests, such as the FireSense field campaign, to refine their models using real-world data from controlled burns.
- Health and Environmental Impacts: A significant focus of the project is on tracking PM 2.5 aerosols—tiny particles from wildfire smoke that pose serious health risks. The project aims to improve understanding of how these particles travel and impact air quality, even far from the source of the fire.
- Broader Climate Implications: The data gathered and modeled by the Wildfire Digital Twin will also help quantify the relationship between wildfire aerosols and precipitation, providing insights into how wildfires influence weather patterns and climate at regional and global scales.
Why This Matters: The Wildfire Digital Twin represents a major advancement in how wildfires are managed and studied, offering a powerful tool to predict and mitigate the impacts of these increasingly frequent and severe events. By improving real-time data integration and model accuracy, this project has the potential to save lives, protect property, and contribute valuable insights into the broader environmental and health impacts of wildfires.
ArTreeficial, an AI-powered device designed to combat the invasive spotted lanternfly
Original Article Title:
This High Schooler Invented an A.I.-Powered Trap That Zaps Invasive Lanternflies
Source: Smithsonian
Date: 8 March 2024
Selina Zhang, an 18-year-old high schooler, invented ArTreeficial, an AI-powered device designed to combat the invasive spotted lanternfly. This solar-powered, self-cleaning “tree” uses an electronic mesh to eliminate the pest, addressing the ecological damage caused by traditional methods like insecticides.
Key Points:
- Innovation: ArTreeficial mimics the tree of heaven, a known lure for the lanternfly, and uses AI to deliver targeted electric shocks.
- Impact: The device offers a more eco-friendly solution, potentially applicable to other invasive species.
Researchers at The University of Texas at Austin have developed an AI that successfully predicted 70% of earthquakes a week in advance
Original Article Title:
AI-Driven Earthquake Forecasting Shows Promise in Trials
Source: University of Texas News
Date: 5 October 2023
Earthquake prediction remains elusive despite significant scientific advances. While researchers understand how earthquakes occur, accurately predicting when, where, and with what magnitude they will strike is still beyond current capabilities. Machine learning models, such as one developed at the University of Texas, have shown promise by predicting 70% of earthquakes during a trial in China, yet challenges remain, including incomplete data and understanding of early warning signs.
Why This Matters: Accurate earthquake prediction could save countless lives, but the complexity and unpredictability of seismic events make this goal difficult to achieve.
AI-Powered VespAI Helps Detect Invasive Asian Hornets with Near-Perfect Accuracy
Original Article Title:
AI helps to detect invasive Asian hornets
Source: University of Exeter
Date: 3 April 2024
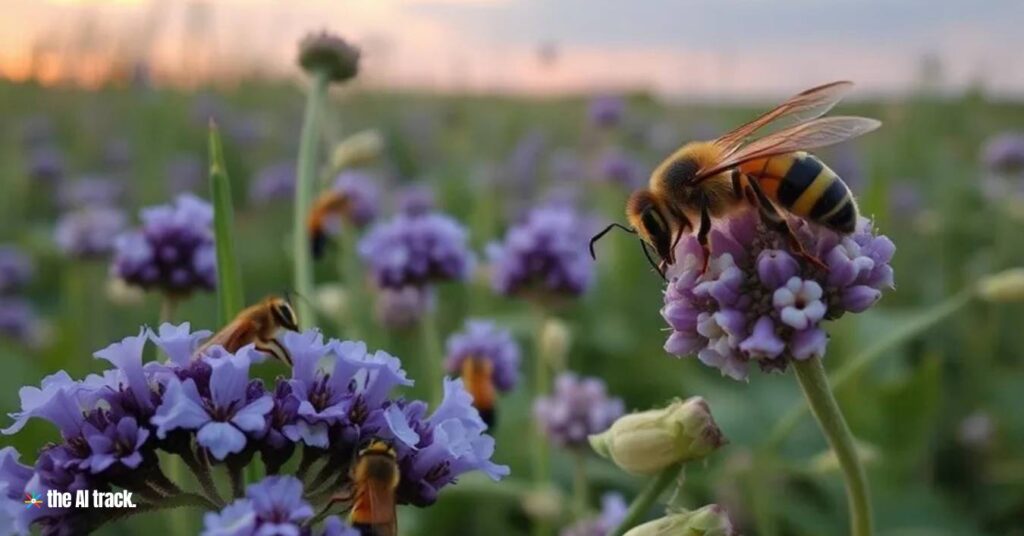
Key Takeaway:
Researchers from the University of Exeter have developed “VespAI,” an artificial intelligence-based system that accurately detects and monitors invasive Asian hornets (Vespa velutina), helping authorities and beekeepers combat their spread. The system is nearly 100% accurate in distinguishing between Asian hornets and other insects, providing a non-invasive solution to track and address this environmental threat.
Key Points:
- Hornet Invasion Problem: The U.K. has been battling invasive Asian hornets, which first appeared in Europe via France in 2004. These hornets pose a serious threat to honeybees, which are essential for pollination and plant life in the region.
- Traditional Methods: Current strategies rely heavily on civilians reporting sightings of Asian hornets, but this leads to many false identifications, making it inefficient for tracking the real threat.
- VespAI System: The AI-powered system attracts hornets and captures images, only activating when it detects an insect of the right size. VespAI then sends the images to researchers for confirmation.
- The system does not harm non-target species, reducing the ecological impact compared to traditional traps.
- The AI exhibited “almost perfect accuracy” in identifying Asian hornets.
- Environmental and Operational Benefits: Unlike other methods that trap and kill various insect species, VespAI focuses on the Asian hornets without harming other insects. Once hornets are detected, they can be tracked back to their nests for destruction, the only effective way to combat the hornet populations.
- Testing Success: VespAI has been extensively tested on the island of Jersey, which sees frequent incursions of Asian hornets due to its proximity to France. The system performed exceptionally well, accurately distinguishing between European hornets, Asian hornets, and other insects, even in large numbers.
- Collaborative Efforts: The project involved collaboration with key organizations such as Defra, the National Bee Unit, the British Beekeepers Association, and Vita Bee Health. The research was funded by the Biotechnology and Biological Sciences Research Council (BBSRC).
Why This Matters:
The development of VespAI offers a highly accurate and environmentally friendly solution to the growing issue of Asian hornet invasions, which threaten both local ecosystems and honeybee populations. By allowing precise tracking and identification, the system aids in more effective pest control measures, potentially preventing significant agricultural and ecological damage. Its deployment could play a crucial role in managing and mitigating future invasive species threats in the U.K. and other affected regions.
Zabble Zero AI Boosts Waste Sorting with 97.6% Specificity
Original Article Title:
How Good is the Latest Zabble Zero AI™ in Identifying Contaminants?
Source: Zabble Blog
Date: 22 April 2024
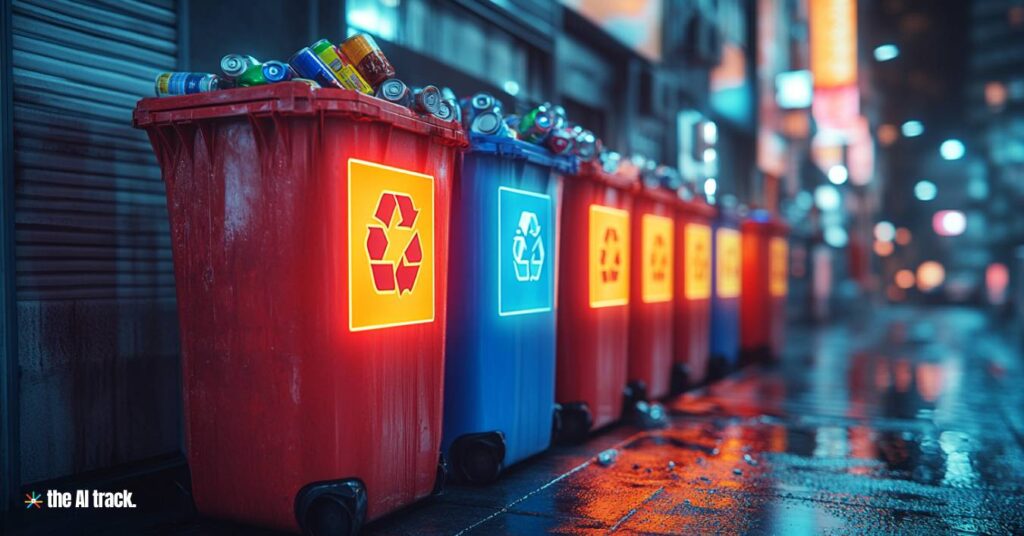
Key Points:
- Introduction of Zabble Zero AI™:
- Zabble Zero AI™ uses object detection to identify waste items and contaminants in photos, helping organizations track waste streams more efficiently. The AI was tested across 10 item categories, such as aluminum cans, food, and cardboard, using mobile tagging to scan bins and detect contaminants.
- Testing Methodology:
- The testing involved placing selected waste items into bins, scanning them using the AI system, and noting whether the AI accurately identified the item. Criteria for accuracy included the appearance of a detection box around the item, a correct label, and a listing of the item as an unacceptable contaminant.
- Detection Results:
- The AI system achieved an overall accuracy rate of 73%, with aluminum cans having a perfect detection score. However, categories like food, plates, and bottles had significantly lower accuracy, with only half of the items correctly identified.
- The specificity rate of the AI was 97.6%, meaning the model is highly effective at recognizing when items are not present in the photo, reducing false positives.
- Challenges with False Positives:
- In some cases, items like paper and cardboard were wrongly detected, despite not being present in the image. Bottles, containers, cups, and food were also occasionally misclassified, leading to some confusion in the AI’s predictions.
- Future Improvements:
- Zabble plans to continuously retrain the AI model with additional images and item categories to improve accuracy. With more use, the AI system is expected to enhance its object detection capabilities, reducing errors and increasing its reliability for waste monitoring.
Why This Matters:
Accurately detecting contaminants in waste streams is crucial for improving recycling and waste management efficiency. Zabble Zero AI™ presents a powerful tool for streamlining waste monitoring, though its current limitations highlight the need for further development. As the AI learns from more data, it has the potential to significantly enhance the accuracy of waste classification, benefiting both environmental initiatives and operational efficiency for organizations.
Bryde’s Whales Identified as Source of Biotwang Calls in the North Pacific
Original Article Title:
Bryde’s whales produce Biotwang calls, which occur seasonally in long-term acoustic recordings from the central and western North Pacific
Source: Frontiers
Date: 18 September 2024
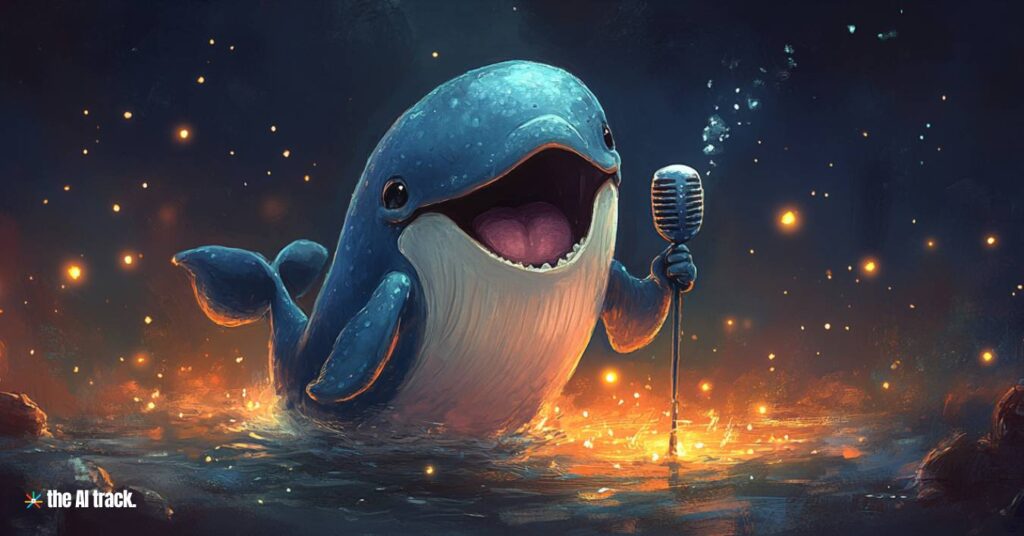
Key Takeaway:
Researchers have identified Bryde’s whales as the producers of unique Biotwang calls in the North Pacific. These calls, detected seasonally and geographically dispersed across the region, provide insights into the whales’ migration patterns and population structure.
Key Points:
Discovery of Biotwang Calls:
Biotwang calls, a distinct type of vocalization, were first detected in 2014 near the Mariana Archipelago. The calls consist of five parts, ranging from low to high frequencies, including a metallic sound. Initially attributed to baleen whales, recent studies confirmed that these calls are produced by Bryde’s whales.
Geographic Distribution and Seasonal Presence:
Biotwangs were consistently detected at four main sites: Saipan, Tinian, Pagan, and Wake Island, with occasional detections in the Northwestern Hawaiian Islands and near the equator at Howland Island. The calls were most frequent between February-April and August-November, aligning with the whales’ migration between low and mid-latitudes.
Bryde’s Whale Populations and Movement Patterns:
The seasonal patterns of Biotwang occurrences suggest that Bryde’s whales in the Western North Pacific (WNP) have a broad yet complex range influenced by changing oceanographic conditions. This population does not undertake long-range migrations like other baleen whales but instead moves widely within ocean basins, feeding regularly in response to prey distribution.
Use of Acoustic Monitoring:
Passive Acoustic Monitoring (PAM) was used to collect long-term data on Biotwang occurrences. Both manual and machine learning techniques helped identify and verify the calls, revealing the distribution and movements of these elusive whales over time. The data showed variations in calling rates and migration patterns, which are influenced by environmental conditions.
Significance for Conservation and Research:
The findings highlight the importance of acoustic data in understanding Bryde’s whale behavior, especially given the species’ cryptic nature and complex population structure. This research contributes valuable insights into their seasonal movements and helps distinguish different populations within the North Pacific.
Why This Matters:
Understanding the vocal patterns and migration behavior of Bryde’s whales is crucial for their conservation, particularly in managing their habitats and mitigating potential human impacts. This research also enhances our knowledge of whale communication and population dynamics in the North Pacific.
Eyes on the Ocean: How AI is Catching Maritime Crimes
Original Article Title:
The Algorithmic Ocean: How AI is Revolutionizing Marine Conservation
Source: MIT Press
Date: 11 April 2024
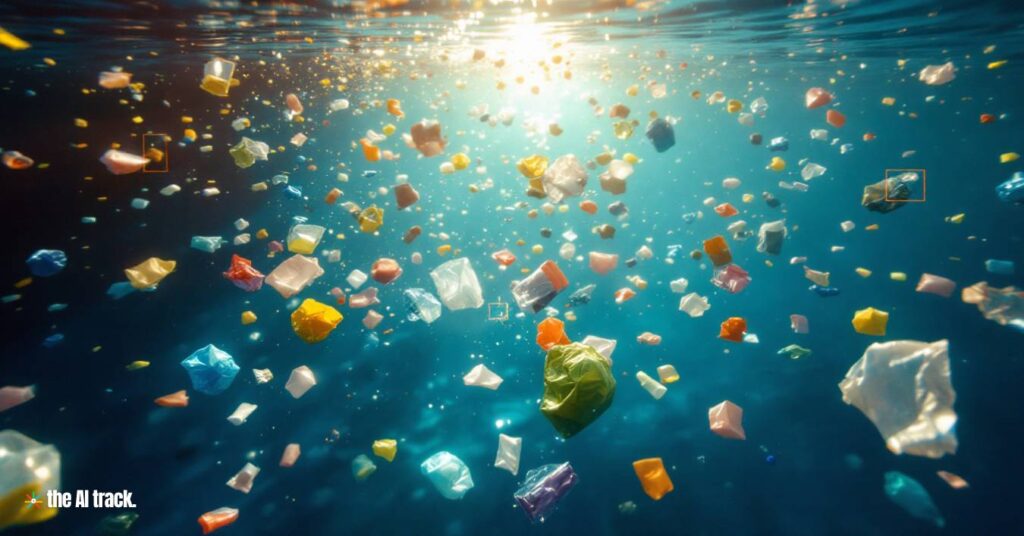
Key Takeaway:
AI is transforming marine conservation efforts by enabling the tracking of illegal fishing and other maritime crimes through real-time vessel monitoring and predictive algorithms. These technologies offer powerful tools for environmental protection and law enforcement in remote ocean regions.
Key Points:
- Nautical Crimes Investigation Service (NCIS): Founded in 2021, NCIS uses AI to track illegal fishing and maritime crimes, providing enforcement data to nonprofits, national coast guards, and Interpol.
- Belhabib’s Background: Dyhia Belhabib, a marine scientist, co-founded NCIS and developed the world’s largest registry of illegal fishing vessels, tackling overfishing through AI-powered risk assessments.
- AI Tools: NCIS’s tools—ADA (AI-powered crime detection) and GRACE (risk assessment)—use AIS signals to monitor vessel movements, identifying illegal activities in real-time.
- Global Fisheries Crisis: Over 90% of global fish stocks are overfished, with large fleets from countries like China exacerbating the issue. Local governments and organizations like Global Fishing Watch use AI to monitor and regulate these activities.
- Digital Conservation vs. Overfishing: While AI helps conservationists, fishers also use digital technologies to exploit marine resources, worsening the overfishing crisis.
- Governance Challenges: Although digital tools assist in monitoring, overfishing remains a governance issue that AI alone cannot solve. International treaties and regulation reforms are necessary to protect marine ecosystems effectively.
Why This Matters:
AI offers a promising solution to one of the most persistent problems in marine conservation—illegal fishing. By enhancing transparency and accountability, it aids environmental enforcement agencies globally. However, AI must work alongside governance reform to address the root causes of overfishing, ensuring a sustainable future for marine life.
New Algorithm Could Slash Energy Use by 95%
Original Article Title:
Integer addition algorithm could reduce energy needs of AI by 95%
Source: TechXplore
Date: 12 October 2024
Key Takeaway:
BitEnergy AI has developed a new method called Linear-Complexity Multiplication that replaces energy-intensive floating-point multiplication with integer addition, reducing AI applications’ energy needs by 95%.
Key Points:
- Energy Consumption: AI models like ChatGPT consume immense amounts of energy—around 564 MWh per day, equivalent to powering 18,000 homes.
- New Method: The new technique replaces floating-point multiplication with integer addition, significantly lowering energy demand without reducing performance.
- Hardware Requirements: Adoption requires different hardware, but prototypes have already been built and tested.
- Impact: Nvidia’s response to this innovation could impact market adoption.
Why This Matters:
The reduction in energy usage can lead to more sustainable AI operations, lowering costs and environmental impacts.
AI in Geoscience: Cracking Earth’s Code with Algorithms
Original Article Title:
Advancing Geoscience with AI: Opportunities and Challenges
Source: Nature
Date: 9 October 2024
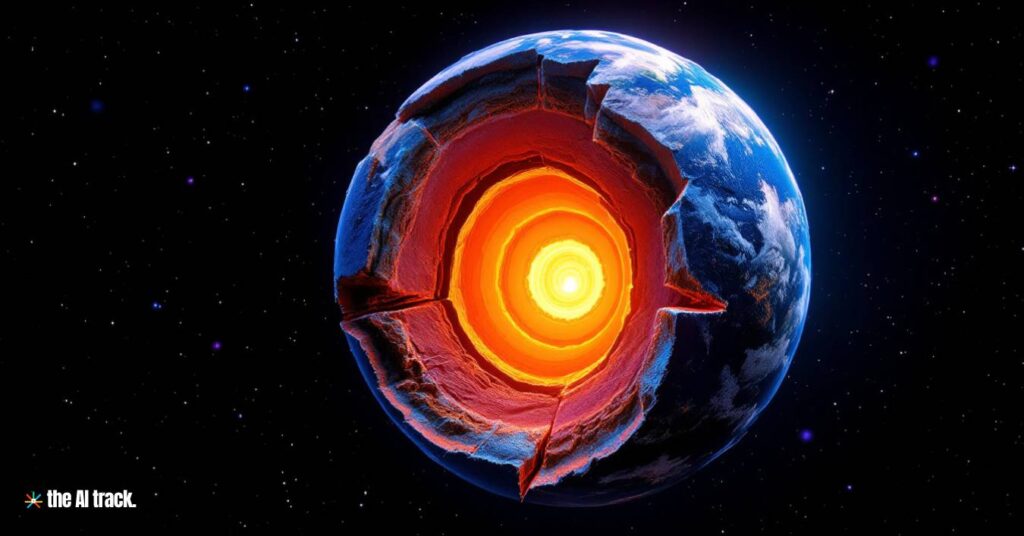
Key Takeaway:
Artificial intelligence (AI), particularly machine learning, is revolutionizing geoscience by enabling advanced data analysis and model building. However, there are challenges related to data complexity, AI expertise in the geoscience community, and transparency, which need to be addressed for broader adoption and trust.
Key Points:
Growing Use of AI in Geoscience:
Since 2015, AI techniques, such as machine learning, have been increasingly used in geoscience research. These methods are now a vital part of many Earth science studies, including those published in Nature Geoscience. AI provides efficient tools for processing vast datasets, analyzing patterns, and tuning model parameters, offering unprecedented insights into complex geoscience data.
Variety of AI Methods and Applications:
AI methods vary from simple classification algorithms to sophisticated deep learning models. AI is especially useful in analyzing “big data” generated from remote sensing, in-situ sensing, and numerical modeling, which are becoming more prevalent in geoscience. In some cases, AI can directly identify dominant variables influencing complex processes.
Challenges in AI Integration:
Despite its potential, integrating AI into geoscience is not without difficulties. Many geoscience datasets have biases and multimodal attributes that complicate AI analysis. Additionally, real-world processes are complex and require the integration of AI with physical models, especially for tasks like multiscale Earth system modeling. A major challenge is the limited AI expertise within the geoscience community, which could lead to flawed results and misapplication of AI methods.
Building Trust Through Transparency:
Transparency is essential for fostering trust in AI within the geoscience community. Experts recommend sharing data, code, and models while using explainable AI techniques, avoiding black-box methods that obscure the reasoning behind results. Journals like Nature Geoscience encourage this transparency by promoting the FAIR principles—data that is findable, accessible, interoperable, and reusable—and facilitating the sharing and peer review of code.
Education and Cross-Disciplinary Collaboration:
A key to advancing AI in geoscience is improving education and cross-disciplinary collaboration. Experts suggest that reforms in education and research funding are necessary to prioritize AI knowledge within the geoscience field. Cross-disciplinary collaboration between AI researchers and geoscientists can help overcome the current knowledge gap and drive advancements in both AI and Earth science.
Why This Matters:
AI presents significant opportunities for advancing geoscience, but realizing its full potential depends on overcoming challenges related to data complexity, trust, and expertise. By focusing on education, transparency, and collaboration, the geoscience community can better harness AI’s power to enhance our understanding of the Earth and its systems.
AI improves significantly the reliability of predicting extreme flood events in ungauged watersheds
Original Article Title:
Global prediction of extreme floods in ungauged watersheds
Source: Nature
Date: 20 March 2024
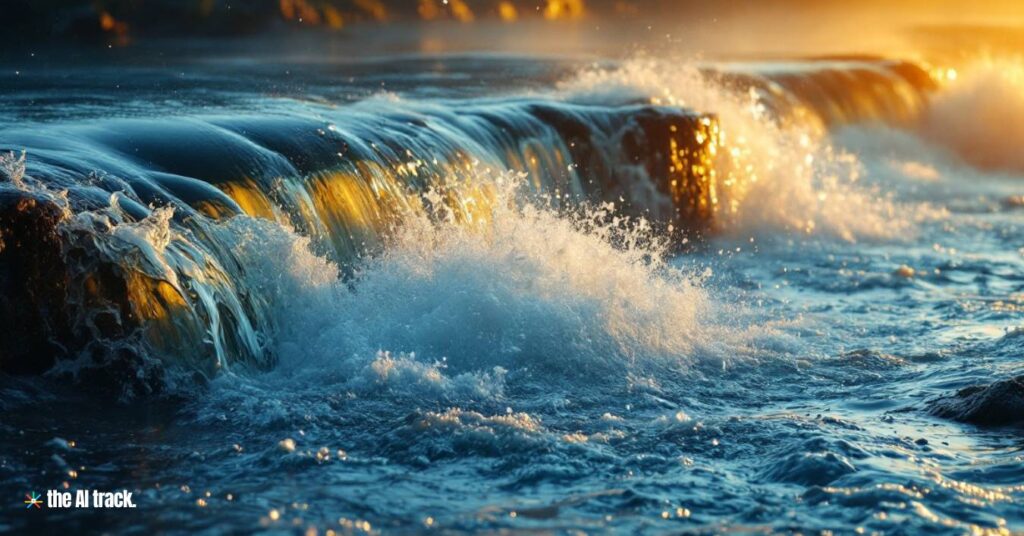
Artificial intelligence-based forecasting significantly improves the reliability of predicting extreme flood events in ungauged watersheds, providing early warnings up to five days in advance, which is comparable to or better than current systems.
Summary
- Floods, being one of the most common natural disasters, disproportionately affect developing countries with sparse streamflow gauge networks.
- Accurate and timely flood warnings are crucial for mitigating risks, but hydrological simulation models typically require lengthy calibration processes.
- Artificial intelligence-based forecasting demonstrates reliability in predicting extreme riverine events in ungauged watersheds with lead times of up to five days.
- The AI model achieves accuracies over five-year return period events comparable to or better than current accuracies over one-year return period events.
- Incorporating AI into operational early warning systems enables the production of publicly available forecasts in real-time in over 80 countries.
- There’s a pressing need to increase the availability of hydrological data to enhance global access to reliable flood warnings.
- The study evaluates the effectiveness of AI trained on open, public datasets to improve global access to flood forecasts.
- Only a few percent of the world’s watersheds are gauged, posing challenges for reliable flood forecasting, especially in vulnerable regions.
- The AI model utilizes long short-term memory (LSTM) networks to predict daily streamflow through a seven-day forecast horizon.
- It is challenging to predict where one model performs better than another based on catchment attributes.
- AI-based forecasting offers better reliability in most places, but it’s essential to predict where different models can be expected to be more or less reliable.
- Both the AI model and GloFAS show differences in reliability across continents and return periods.
- Improving global flood predictions and early warning systems is critical for millions of people worldwide, and increasing access to data is key to achieving this goal.
- The study emphasizes the importance of disseminating flood warnings in a timely manner and provides open-access real-time forecasts to support notifications.
- There is still room for improvement in global flood predictions, and increasing access to hydrological data is essential for advancing forecasting models.
AI Revolutionizes Marine Science: Studying Plankton - Key to Carbon Sequestration
Original Article Title:
AI in the Ocean Twilight Zone: Understanding Plankton Through Deep Learning
Source: Oceans
Date: 31 May 2023
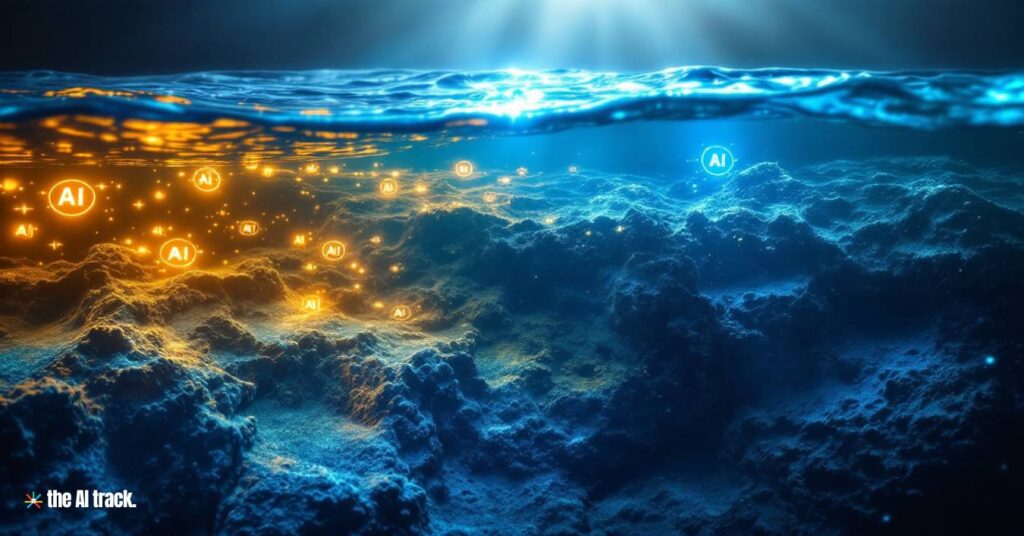
Key Takeaway:
AI is revolutionizing marine research, especially in studying plankton, key to carbon sequestration. Researchers at Woods Hole Oceanographic Institution (WHOI) are using AI to analyze data from the ocean’s twilight zone, where plankton reside, to understand their impact on climate and ocean ecosystems.
Key Points:
- AI Applications: AI processes high-speed images of plankton, classifying species, and detecting patterns, a vast improvement over traditional methods.
- Carbon Role: Plankton helps absorb 40% of human-emitted carbon dioxide, making their study crucial for climate research.
- Technological Integration: AI tools work with autonomous vehicles and acoustic systems to gather data on species migration, distribution, and behavior.
- AI Tools: Led by Biologist Heidi Sosik, AI algorithms analyze millions of plankton images, revealing insights into their species, vertical migrations, and their role in the ecosystem.
Why This Matters:
This cutting-edge AI research is critical for understanding the ocean’s role in regulating global climate, enabling better stewardship of marine resources. As plankton are crucial for carbon capture, protecting them helps mitigate climate change.