
AI is revolutionizing healthcare by enhancing diagnostics, personalizing treatments, and streamlining operations. Discover the most groundbreaking of AI Breakthroughs in Health, that are transforming the way we understand and approach human health.
Browse all the other fields in our curated collection of the most important AI Breakthroughs. Each section offers insights into how AI is transforming different sectors, providing a comprehensive view of its impact across a wide range of disciplines.
AI Breakthroughs in Computer Science - At a Glance
The Revolution of AI in Healthcare: From Workflow Optimization to Personalized Medicine
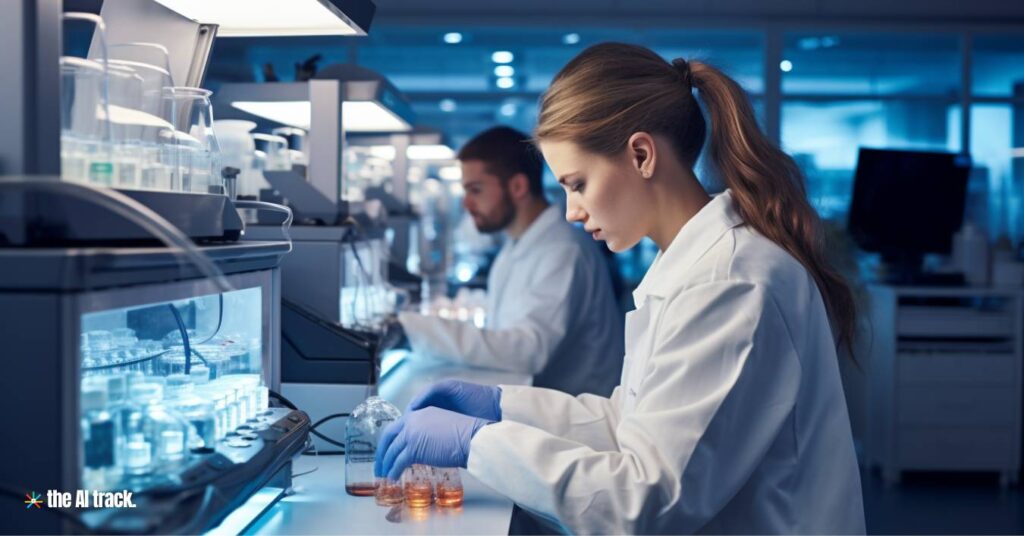
The AI Track’s extensive analysis of the impact of AI in medical technology – how it is transforming healthcare by streamlining workflows, facilitating personalized medicine, and beyond. Explore the profound impact of AI in the medical field.
Diagnostics and Early Detection
AI tool predicts kidney failure six times faster than human expert analysts
Original Article Title:
AI tool predicts kidney failure six times faster than human expert analysts
Source: Medical Express
Date: March 4, 2024
Key Takeaway
Artificial intelligence (AI) is being utilized by kidney doctors and clinical scientists at Sheffield Teaching Hospitals NHS Foundation Trust to significantly enhance the prediction of kidney failure, with the AI tool being six times faster than manual analysis.
Summary
- Kidney doctors and clinical scientists at Sheffield Teaching Hospitals NHS Foundation Trust are leveraging artificial intelligence (AI) to improve the prediction of kidney failure.
- The AI tool, which provides rapid analysis of total kidney volume, is particularly beneficial for patients with autosomal dominant polycystic kidney disease (ADPKD).
- ADPKD affects a significant number of individuals in the UK, with current monitoring relying on labor-intensive manual processes involving MRI scans.
- Manual segmentation of MRI scans is time-consuming, requiring analysts to trace around the kidneys on numerous slices, taking up to an hour per patient.
- By automating the segmentation process, the AI tool delivers results in less than a minute, significantly reducing the time and effort required.
- The AI tool performed comparably to human expert analysts, but with a sixfold increase in speed.
- Development of the AI tool involved training an algorithm on hundreds of kidney MRI scans, resulting in accurate and efficient kidney volume measurements.
- The software has been in use at Sheffield Teaching Hospitals NHS Foundation Trust since 2022 and has the potential for widespread adoption in kidney clinics globally.
- Rapid and accurate prediction of kidney failure is crucial for patients with ADPKD, enabling timely intervention and management to prevent irreversible kidney damage.
- The AI tool facilitates quick and precise measurement of kidney volume, addressing challenges associated with a lack of specialist expertise in some clinics.
- Professor Albert Ong, consultant nephrologist and clinical lead for genetics at Sheffield Teaching Hospitals NHS Foundation Trust, highlights the importance of early prediction of kidney failure to improve patient outcomes and quality of life.
Google AI could soon use a person’s cough to diagnose disease
Original Article Title:
Google AI could soon use a person’s cough to diagnose disease
Source: Nature
Date: 21 March 2024
Google AI has developed a machine-learning tool trained on millions of human audio clips, which shows promise for detecting diseases like COVID-19 and tuberculosis by analyzing coughing and breathing sounds.
Summary
- Google AI has created a machine-learning system capable of detecting and monitoring health conditions by analyzing sounds like coughing and breathing.
- The system has been trained on millions of audio clips of human sounds.
- It could potentially be used by physicians to diagnose diseases such as COVID-19 and tuberculosis, and to assess lung function.
- References to related research papers are provided for further reading.
- The article also mentions other advancements in AI in medicine, including better diagnoses and disease detection using AI algorithms.
- Job postings related to bioinformatics and ecological modeling are listed at the end of the page.
- Nature Portfolio information and services are provided at the bottom of the page, including publishing policies, author services, and research solutions.
Breakthrough AI Can Now Predict Alzheimer's Up to 7 Years in Advance
Original Article Title:
Breakthrough AI Can Now Predict Alzheimer’s Up to 7 Years in Advance
Source: ScienceAlert
Date: 14 March 2024
Breakthrough AI models trained on health records can predict Alzheimer’s development up to 7 years in advance with 72% accuracy, aiding in early detection and potential preventative measures.
Key Points
- Breakthrough AI models developed by researchers from UCSF and Stanford University can predict Alzheimer’s development up to 7 years in advance.
- The AI system was trained on more than 5 million health records to spot patterns connecting Alzheimer’s to other health conditions.
- While not perfect, the AI accurately predicted Alzheimer’s development 72% of the time in tested cases.
- Machine learning was utilized to identify connections between Alzheimer’s and various health conditions such as high blood pressure, high cholesterol, depression, etc.
- The predictive power of the AI model stems from its ability to analyze different risk types and calculate the likelihood of Alzheimer’s development.
- The research aims to use AI on routine clinical data to identify risks early and understand the biology behind them.
- Identified conditions such as high blood pressure, high cholesterol, vitamin D deficiency, and depression were significant factors in predicting Alzheimer’s risk.
- Erectile dysfunction and an enlarged prostate were significant factors in men, while osteoporosis was significant for women.
- The combination of diseases allows the model to predict Alzheimer’s onset, highlighting the interplay between various health factors.
- Osteoporosis, Alzheimer’s in women, and a gene variant were found to be connected, offering new opportunities for studying Alzheimer’s development.
- The research has been published in Nature Aging, showcasing the potential of AI in predicting and understanding Alzheimer’s disease.
New AI Tool Mines Cancer Patients’ Pathology Data
Original Article Title:
New AI Tool Mines Cancer Patients’ Pathology Data
Source: Cedars-Sinai
Date: 1 March 2024
Artificial intelligence (AI) has been employed by Cedars-Sinai investigators to enable computers to access and analyze crucial information from cancer patients’ pathology reports, facilitating research and clinical trial recruitment.
Key Points
- Cedars-Sinai investigators utilized AI to make pathology reports, containing vital cancer-related data, accessible to computers.
- The method, described in the peer-reviewed data science journal Patterns, involves cleaning up scanned pathology reports for machine readability.
- This approach enables the extraction of information from pathology reports for research purposes and clinical trial recruitment.
- The dataset created through this process, encompassing data from nearly 10,000 cancer patients, is now publicly available and suitable for machine learning applications.
- The AI-enhanced optical character recognition improves the extraction of clinically relevant data from patient records.
- The project received support from the National Institute of General Medical Sciences of the National Institutes of Health.
- The implications of this AI tool extend to improving precision medicine efforts and enhancing computer interpretation of clinical language.
- Cedars-Sinai investigators are focusing on further refining the tool to extract specific information like cancer staging from pathology reports.
- Future applications include integrating this method into the Molecular Twin Precision Oncology Platform and making other clinician notes machine-readable.
- Collaboration and interdisciplinary research are crucial for leveraging AI tools effectively in medical research and clinical practice.### Key Takeaway Artificial intelligence (AI) has been employed by Cedars-Sinai investigators to make cancer patients’ pathology reports machine-readable, enabling the extraction of crucial information for research and clinical trial recruitment.
AI tool called Mia successfully identified tiny signs of breast cancer in 11 women during an NHS test
Original Article Title:
NHS AI test spots tiny cancers missed by doctors
Source: BBC
Date: 21 March 2024
An AI tool called Mia successfully identified tiny signs of breast cancer in 11 women during an NHS test, highlighting its potential to assist clinicians in early cancer detection.
Key Points
- An AI tool named Mia, tested by the NHS, identified tiny signs of breast cancer in 11 women that had been missed by human doctors.
- Mia was piloted alongside NHS clinicians and analyzed mammograms of over 10,000 women, successfully flagging all those with symptoms and an additional 11 missed by doctors.
- The tool’s ability to detect early-stage cancers, which can be extremely small and hard to spot, was showcased during the trial.
- Patients whose cancers were flagged by Mia had the advantage of early detection, leading to less invasive treatment and better survival rates.
- Mia has the potential to reduce waiting times for results from 14 days to three, significantly expediting diagnosis and treatment.
- Each case in the trial underwent both AI and human review, indicating a collaborative approach to diagnosis.
- Mia’s development involved inclusive data collection and training, emphasizing the importance of diversity in AI for healthcare.
- Despite its capabilities, Mia isn’t perfect and had limitations such as the inability to access patient history and the disabled machine learning element due to health regulations.
- The Mia trial, while promising, is one of many ongoing AI trials in healthcare, aiming to improve diagnostics and reduce pressure on NHS staff.
- Experts emphasize the need for further research to optimize the use of AI technology in improving cancer patient outcomes.
Overall, Mia’s successful identification of early-stage breast cancers underscores the potential of AI tools in assisting clinicians and improving cancer detection rates, though further research and development are needed to fully realize this potential.
AI improves accuracy of skin cancer diagnoses in Stanford Medicine-led study
Original Article Title:
AI improves accuracy of skin cancer diagnoses in Stanford Medicine-led study
Source: Stanford Medicine
Date: 11 April 2024
A recent study by Stanford Medicine has shown that AI can significantly improve the accuracy of skin cancer diagnoses. The study revealed that AI assistance increased diagnostic sensitivity to 81.1% and specificity to 86.1%. This technology not only improves patient care but also has potential benefits in reducing physician burnout and enhancing doctor-patient relationships
Portable and AI-enabled ultrasound devices are transforming maternal health in low-resource settings by allowing midwives to perform critical assessments and identify high-risk pregnancies
Original Article Title:
Ultrasounds in hand, midwives are transforming maternal health
Source: Bill & Melinda Gates Foundation
Date: 1 May 2024
Portable and AI-enabled ultrasound devices are transforming maternal health in low-resource settings by allowing midwives to perform critical assessments and identify high-risk pregnancies, significantly improving maternal and newborn outcomes.
Key Points:
- New handheld ultrasound devices, costing 5% of traditional machines, are now accessible to midwives.
- AI capabilities help in identifying pregnancy risks, improving decision-making.
- A study in Kenya showed midwives effectively using these devices, reducing fetal deaths and complications.
Why This Matters: Increasing access to affordable, advanced ultrasound technology in low-resource settings can save lives and improve maternal health care quality, supporting global health equity.
New AI System Detects Rare Epileptic Seizures
Original Article Title:
New AI System Detects Rare Epileptic Seizures
Source: USC Viterbi | School of Engineering
Date: 4 June 2024
USC researchers have developed an AI system that significantly improves the detection of rare epileptic seizures by analyzing brain interactions from EEG data. This technology promises to enhance early diagnosis and treatment, potentially saving lives by identifying complex seizure patterns that are difficult for humans to detect.
Key Points:
- Prevalence and Impact of Epilepsy:
- Over 3.4 million people in the U.S. and 65 million worldwide have epilepsy.
- Epilepsy causes seizures and affects the nervous system, with 1 in 26 people developing epilepsy in their lifetime.
- Approximately 1,000 people with epilepsy die unexpectedly each year.
- AI System Development:
- The AI model analyzes brain interactions using EEG data, integrating multiple sources of information like electrode positions and brain regions.
- It demonstrates a 12% improvement over existing models in detecting rare seizures, enhancing diagnostic accuracy.
- Clinical Application:
- The system supplements doctors’ diagnoses by providing additional data to identify seizures that might be missed.
- It could eventually be incorporated into wearable sensors for real-time monitoring, offering continuous support for epilepsy patients.
Why This Matters: Early and accurate detection of epileptic seizures is crucial for effective treatment and improving the quality of life for patients. With 70% of people with epilepsy potentially living seizure-free if adequately diagnosed and treated, this AI system can lead to timely medical responses, reducing risks associated with undiagnosed seizures. Integrating this technology into wearable devices can provide continuous monitoring, helping patients and healthcare providers manage epilepsy more effectively. This advancement showcases the potential for AI to improve healthcare outcomes and save lives.
Google has developed an AI model called HeAR to detect diseases by analyzing sounds such as coughs
Original Article Title:
This AI Model is Helping Researchers Detect Disease Based on Coughs
Source: Google The Keyword
Date: 19 August 2024
Google has developed an AI model called Health Acoustic Representations (HeAR) to detect diseases by analyzing sounds such as coughs. This model is designed to assist in early detection of conditions like tuberculosis (TB) and chronic obstructive pulmonary disease (COPD).
Key Points:
- Technology: HeAR is trained on 300 million audio samples, including 100 million cough sounds, to recognize disease patterns.
- Applications: Salcit Technologies in India is using HeAR to enhance TB detection.
- Impact: This AI model aims to make healthcare more accessible, especially in low-resource settings.
ChatGPT-4 produces 'near perfect' pancreatic cancer radiology reports
Original Article Title:
This AI Model is Helping Researchers Detect Disease Based on Coughs
Source: AuntMinnie.com
Date: 18 June 2024
ChatGPT-4, OpenAI’s language model, has demonstrated the capability to produce nearly perfect radiology reports for pancreatic cancer, showcasing its potential in medical imaging and diagnostics.
Key Points:
- ChatGPT-4 generates highly accurate pancreatic cancer radiology reports.
- The AI model’s performance in this task highlights its potential utility in medical diagnostics.
- This achievement represents a significant advancement in the application of AI in healthcare, particularly in medical imaging.
Why This Matters: The ability of AI to produce accurate radiology reports can streamline the diagnostic process, reduce the workload for radiologists, and potentially improve patient outcomes through faster and more precise diagnoses.
Potential to improve early detection and management in glaucoma treatment
Original Article Title:
The Future of AI in Glaucoma Is Around the Corner, Though Not Without Some Challenges.
Source: American Academy of Ophthalmology
Date: 19 March 2024
Artificial intelligence is poised to revolutionize glaucoma management, offering significant advancements in disease diagnosis, monitoring, and treatment. Despite promising potential, several challenges must be addressed before AI can be fully integrated into clinical practice.
Key Points:
- Diagnostic Accuracy:
- AI algorithms have shown high accuracy in diagnosing glaucoma, often surpassing human experts.
- Machine learning models can analyze large datasets from visual field tests, optical coherence tomography (OCT) scans, and fundus photographs to detect early signs of glaucoma.
- Monitoring Disease Progression:
- AI can assist in monitoring glaucoma progression by analyzing sequential imaging data.
- Automated systems can detect subtle changes over time, potentially leading to earlier intervention and better outcomes.
- Personalized Treatment Plans:
- AI has the potential to tailor treatment plans based on individual patient data, improving the precision of therapeutic interventions.
- Predictive models can forecast disease progression and response to treatment, aiding clinicians in decision-making.
- Challenges and Barriers:
- Integration of AI into clinical workflows requires addressing issues related to data standardization, interoperability, and validation.
- Ethical and regulatory considerations, including patient privacy and algorithm transparency, need careful management.
- Future Prospects:
- Ongoing research and development are expected to enhance the robustness and reliability of AI tools in glaucoma care.
- Collaboration between AI developers, clinicians, and regulatory bodies will be crucial to overcoming current limitations and ensuring safe implementation.
Why This Matters: The integration of AI in glaucoma management could lead to earlier detection, more accurate monitoring, and personalized treatment, ultimately improving patient outcomes and reducing the burden of blindness caused by the disease. However, the path to full implementation involves overcoming significant technical, ethical, and regulatory challenges.
Artificial intelligence outperforms clinical tests at predicting progress of Alzheimer’s disease
Original Article Title:
Artificial intelligence outperforms clinical tests at predicting progress of Alzheimer’s disease
Source: University of Cambridge
Date: 12 July 2024
A new AI tool developed by Cambridge scientists predicts the progression of Alzheimer’s disease more accurately than current clinical methods, potentially improving early intervention and reducing unnecessary tests.
Key Points:
- AI model predicts Alzheimer’s progression with 82% accuracy using non-invasive data.
- Early detection could lead to better patient outcomes and reduce misdiagnosis.
- The model was validated with data from 1,900 individuals across the UK and Singapore.
- The tool helps differentiate between patients likely to remain stable and those who will progress rapidly.
Why This Matters: Improving Alzheimer’s prediction accuracy can enhance early treatment, reduce healthcare costs, and alleviate patient and family anxiety.
Scientists have developed an AI model that can predict the risk of a heart attack within the next 10 years by analyzing CT scan
Original Article Title:
‘Game changer’ AI detects hidden heart attack risk, say scientists
Source: BBC
Date: 5 August 2024
A new AI model developed by Caristo Diagnostics, an Oxford University spinout, can analyze CT scans to predict a patient’s risk of heart attack within the next 10 years by detecting cardiac inflammation that is not visible in traditional scans. This technology is seen as transformative and could soon be implemented within the NHS.
Key Points:
Technology Overview:
The AI model, called CaRi-Heart, identifies coronary inflammation and plaque buildup, which are indicators of a higher risk for heart attacks. Traditional CT scans fail to show this inflammation, making the AI tool a significant advancement.
Pilot Project:
A pilot program, supported by NHS England, is currently running at five hospital trusts: Oxford, Milton Keynes, Leicester, Liverpool, and Wolverhampton. The program aims to evaluate the AI’s effectiveness and its potential for widespread use in the NHS.
Research Findings:
The Orfan study, involving 40,000 patients, demonstrated that individuals with detected coronary inflammation were 20 to 30 times more likely to suffer a fatal cardiac event within 10 years. The AI model allowed for early intervention, with 45% of high-risk patients receiving medications or lifestyle recommendations.
Economic Impact:
The British Heart Foundation (BHF) estimates that heart disease affects 7.6 million people in the UK, with an annual cost to the NHS of £7.4 billion. The AI’s potential to prevent heart attacks could significantly reduce these costs.
Patient Impact:
Ian Pickard, a 58-year-old participant in the Orfan study, was identified as at high risk of a heart attack after an AI analysis of his CT scan. Following the diagnosis, he was prescribed statins and advised to quit smoking and increase his exercise, marking a significant change in his health management.
Future of AI in Healthcare:
The National Institute for Health and Care Excellence (NICE) is currently assessing whether to implement this AI technology across the NHS. It is also being reviewed in the US and has already been approved in Europe and Australia.
Why This Matters:
This AI technology represents a significant leap forward in preventive cardiology, potentially saving thousands of lives by enabling earlier and more accurate risk assessments for heart attacks. Its adoption could lead to a major shift in how cardiovascular diseases are managed, reducing healthcare costs and improving patient outcomes.
Tongue Disease Prediction Based on Machine Learning Algorithms
Original Article Title:
Tongue Disease Prediction Based on Machine Learning Algorithms
Source: MDPI
Date: 28 June 2024
A new study presents an AI-based imaging system designed to diagnose tongue diseases by analyzing color features under various lighting conditions. Utilizing 5,260 images and six machine learning algorithms, XGBoost achieved the highest accuracy at 98.71%, making it the preferred method for real-time disease prediction.
Key Points:
- AI Application: The system analyzes tongue color across five color space models.
- Accuracy: XGBoost outperformed other models with a 98.71% accuracy rate.
- Real-Time Use: The system is integrated with a GUI for immediate diagnostics.
Why This Matters: This technology enhances non-invasive diagnostic capabilities, potentially improving point-of-care health systems.
Researchers claim an 80% accuracy rate for a new AI-based screening system that could help identify toddlers under two years of age who may be autistic
Original Article Title:
AI could help identify toddlers who may be autistic, researchers say
Source: The Guardian
Date: 19 August 2024
Researchers claim an 80% accuracy rate for a new AI-based screening system that could help identify toddlers under two years of age who may be autistic.
Key Points:
- AI Model and its potential:
- Researchers have developed an AI model based on machine learning to identify toddlers at risk of autism.
- The model uses easily obtainable data such as age at first smile, potty training, etc.
- Dr. Kristiina Tammimies believes it can help with early identification and intervention but emphasizes that the final diagnosis should be done clinically.
- The model demonstrated an accuracy of 78.5% for children aged up to two years old, 84.2% for those aged two to four, and 79.2% for those aged four to 10.
- Data and Methodology:
- The model was trained and tested on data from the Spark study involving 15,330 children with autism and 15,330 without.
- 28 easily obtainable measures were used, focusing on parent-reported information from questionnaires.
- Machine learning models were created to look for patterns in combinations of these features among autistic and non-autistic children.
- Significant Measures:
- Problems with eating foods.
- Age at first construction of longer sentences.
- Age at achieving potty training.
- Age at first smile.
- Limitations and Concerns:
- The model’s accuracy in identifying non-autistic people is 80%, meaning 20% could be wrongly flagged.
- Experts caution against pushing for early diagnosis, as it is difficult to differentiate between serious impairments and developmental delays that children might catch up with.
- Professor Ginny Russell recommends against applying psychiatric labels below two years of age based on a few signifiers.
Why This Matters:
- Early identification of autism can lead to timely intervention and support, significantly improving outcomes for children.
- AI-powered tools have the potential to streamline the screening process and aid healthcare professionals in identifying children who might benefit from further evaluation.
- However, the limitations of the model, especially the potential for false positives and the complexities of early diagnosis, need to be carefully considered and addressed.
New AI Tool Revolutionizes Cancer Detection and Prognosis
Original Article Title:
A New Artificial Intelligence Tool for Cancer
Source: Harvard Medical School
Date: 4 September 2024
Key Takeaway: Harvard Medical School has developed a groundbreaking AI model called CHIEF (Clinical Histopathology Imaging Evaluation Foundation) that can perform a wide range of cancer diagnostic tasks across 19 cancer types. This versatile AI system significantly enhances cancer detection, prognosis, and treatment response predictions, outperforming existing AI methods by up to 36 percent.
Key Points:
- AI for Cancer Diagnostics: CHIEF, a new AI tool, was designed to handle various cancer-related tasks, including detecting cancer cells, predicting tumor origin, and patient outcomes. It can even analyze the tumor microenvironment, which is crucial for treatment decisions.
- Wide Application Across Cancer Types: Tested on 19 cancer types, including lung, breast, brain, and colon cancers, CHIEF’s versatility mirrors large AI language models like ChatGPT, making it adaptable across diverse cancer forms.
- Training on Massive Data: CHIEF was trained on 15 million unlabeled images and 60,000 whole-slide images from multiple cancer types, providing it with a deep understanding of both specific regions and the overall context of tumor tissues.
- Global Validation and Superior Performance: CHIEF was tested on over 19,400 images from 32 datasets across 24 hospitals globally. It outperformed other AI tools by up to 36 percent in key tasks like cancer detection and prediction of treatment responses.
- Adaptability Across Techniques: CHIEF was equally effective, regardless of how tumor cells were obtained (biopsy or surgical excision) and how the samples were digitized, making it suitable for various clinical environments.
- Potential for Personalized Treatment: The AI tool not only improves cancer diagnostics but also holds promise for identifying patients who could benefit from experimental treatments targeting specific molecular variations, a capability not widely available today.
Why This Matters:
CHIEF represents a significant advancement in cancer diagnostics, offering clinicians a powerful tool to enhance accuracy in detecting and treating various cancers. Its ability to predict patient outcomes and treatment responses could lead to more personalized cancer care, improving survival rates and the quality of life for patients worldwide. The AI model’s versatility and adaptability across different clinical settings mark a crucial step toward integrating AI in everyday medical practice.
AI-powered tool Chartwatch is saving lives at St. Michael's Hospital in Toronto, reducing unexpected deaths by 26%!
Original Article Title:
AI Tool Reduces Unexpected Deaths by 26% in Canadian Hospitals
Source: CBC
Date: 16 September 2024
Key Takeaway: The AI-based early warning system, Chartwatch, developed by Unity Health Toronto, has shown a 26% reduction in unexpected deaths at St. Michael’s Hospital, Toronto. The AI tool monitors patients’ vital signs, lab results, and other data to predict potential deterioration, enabling medical teams to intervene early and prevent severe outcomes.
Key Points:
- Chartwatch’s Early Warning System:
- Chartwatch is an in-house AI system that monitors patient data, such as vital signs and lab results, to predict deterioration in real-time, providing early alerts to medical teams.
- In a case at St. Michael’s Hospital, the system detected a high white blood cell count in a patient, leading to early diagnosis and treatment for cellulitis, potentially preventing severe complications.
- Study Results:
- A study published in the Canadian Medical Association Journal revealed that using Chartwatch led to a 26% reduction in unexpected deaths among 13,000 admissions to St. Michael’s general internal medicine ward.
- The system monitors around 100 data points per patient and provides an hourly prediction of deterioration, allowing for quicker medical interventions.
- AI Enhances, Not Replaces, Clinical Judgment:
- Chartwatch complements clinical judgment by providing real-time insights, helping nurses and doctors catch deteriorating conditions early.
- In some cases, the system has prompted life-saving escalations in treatment or early palliative care.
- Broader Implications and Future Deployment:
- While the study was conducted in a single hospital during the COVID-19 pandemic, the team at Unity Health Toronto aims to roll out Chartwatch across other hospitals in Ontario, through the GEMINI data-sharing network.
- AI in healthcare shows promise in addressing staff shortages, improving patient outcomes, and reducing preventable deaths, but more research is needed to assess its impact across diverse patient populations.
Why This Matters:
The success of Chartwatch highlights the potential of AI to significantly improve patient care by reducing unexpected deaths. As healthcare systems globally face staffing shortages and increased pressure, AI tools like Chartwatch offer innovative solutions to enhance clinical decision-making, improve patient outcomes, and save lives.
From Movement to Mood: AI Decodes Specific Brain Signals
Original Article Title:
New AI can ID brain patterns related to specific behavior
Source: ScienceDaily
Date: 9 September 2024
Key Takeaway:
Researchers from the University of Southern California (USC) have developed a groundbreaking AI algorithm called DPAD (Dissociative Prioritized Analysis of Dynamics) that can separate and identify specific brain patterns linked to individual behaviors. This breakthrough could enhance brain-computer interfaces (BCIs) and aid in the treatment of movement disorders and mental health conditions.
Key Points:
Development of DPAD Algorithm:
Maryam Shanechi, the Sawchuk Chair in Electrical and Computer Engineering at USC, and her team developed the DPAD algorithm. It dissociates brain patterns linked to a specific behavior, such as arm movement, from other simultaneous brain patterns, allowing for more accurate decoding of brain activity.
Challenge of Mixed Brain Patterns:
The human brain encodes multiple simultaneous behaviors, like moving, speaking, and feeling internal states such as hunger, resulting in complex, mixed patterns of electrical activity. Dissociating these patterns is crucial for developing BCIs that can accurately decode specific behaviors, such as intended movements in paralyzed patients.
Implications for Brain-Computer Interfaces (BCIs):
BCIs aim to restore function in paralyzed patients by decoding intended movements from brain activity and translating them into actions, such as moving a robotic arm. DPAD improves the accuracy of these decodings by focusing on relevant brain patterns, enhancing the performance of BCIs.
Flexibility in Decoding Various Behaviors and Mental States:
The DPAD algorithm prioritizes patterns related to the behavior of interest during the training of neural networks, preventing other patterns from masking or confounding the target patterns. This flexibility allows DPAD to potentially decode other mental states, such as pain or depression, in the future.
Potential Applications in Mental Health:
DPAD could extend beyond movement disorders to track symptom states in mental health conditions, providing real-time feedback for tailoring therapies. This could lead to innovative BCIs that assist in treating mental health issues by monitoring and responding to a patient’s specific symptom states.
Why This Matters:
This technology represents a significant advancement in understanding brain activity, with potential applications in medical fields ranging from movement disorder rehabilitation to personalized mental health treatments. By accurately decoding specific brain patterns, DPAD could revolutionize how BCIs interact with the brain and improve the quality of life for individuals with various neurological and psychiatric conditions.
HeartBEiT is Revolutionizing Cardiac Diagnostics
Original Article Title:
HeartBEiT Cardiac Diagnostics: Transforming ECG Analysis
Source: The AI Track
Date: 9 July 2024
Summary:
HeartBeIT is revolutionizing cardiac diagnostics by leveraging deep learning technologies to enhance the accuracy and efficiency of heart disease detection. This innovative platform uses advanced AI algorithms to analyze medical images and electrocardiogram (ECG) data, providing doctors with critical insights to make better-informed decisions regarding patient care.
Key Points:
- Advanced Deep Learning Models for Cardiac Diagnostics: HeartBeIT employs cutting-edge deep learning models that are specifically trained to detect subtle patterns in cardiac data that might be overlooked by traditional diagnostic methods. These AI models can identify abnormalities in heart function by analyzing vast amounts of data from ECGs, MRIs, and other cardiac imaging modalities. The deep learning algorithms have been trained on thousands of annotated cardiac images, allowing the system to recognize various heart conditions, including arrhythmias, heart failure, and coronary artery disease.
- Streamlining the Diagnostic Process: The platform significantly reduces the time required for cardiac diagnostics by automating the analysis process. Traditional diagnostic methods often involve manual interpretation of ECGs and imaging scans, which can be time-consuming and subject to human error. HeartBeIT’s AI-driven approach provides real-time analysis, delivering diagnostic results faster and more accurately. This rapid processing capability is especially valuable in emergency settings where timely diagnosis is critical for patient outcomes.
- Improving Accuracy and Reducing Errors: One of the main advantages of HeartBeIT is its ability to reduce diagnostic errors. The AI system is designed to cross-check multiple data points, minimizing the risk of misinterpretation that can occur with manual analysis. Studies have shown that HeartBeIT’s diagnostic accuracy often matches or surpasses that of experienced cardiologists, making it a reliable tool for enhancing clinical decision-making.
- Enhanced Patient Monitoring and Predictive Analytics: Beyond diagnostics, HeartBeIT’s AI models are equipped with predictive analytics capabilities that can forecast potential cardiac events based on the patient’s data history. This proactive approach helps in early intervention and personalized treatment planning.
- Integration with Healthcare Systems: HeartBeIT is designed to seamlessly integrate with existing healthcare infrastructures, including electronic health records (EHR) and hospital information systems.
Why It Matters: HeartBeIT’s use of deep learning in cardiac diagnostics represents a significant advancement in medical technology. The platform’s ability to quickly and accurately analyze cardiac data can lead to better patient outcomes, reduce diagnostic errors, and streamline healthcare operations. As the demand for precision medicine continues to grow, solutions like HeartBeIT are poised to play a critical role in the future of healthcare.
Treatment
An interesting project aims to bridge the communication gap between Deaf and hearing individuals using AI
Original Article Title:
How Can AI Bridge Deaf-Hearing Communication?
Source: YouTube – Kevin Thomas
Date: 26 May 2024
Kevin Thomas, a grade 11 student, presents a project aimed at bridging the communication gap between hearing and deaf individuals using American Sign Language (ASL).
Key points include:
- ASL and English Differences: Kevin explains that ASL is not the same as English; it has its own syntax, grammar, and vocabulary. This misconception is a challenge for existing translation tools.
- Current Translation Tools’ Limitations: Most tools only convert speech to text and vice versa, which adds steps for deaf individuals to be part of a conversation. Kevin’s project seeks to eliminate these additional steps.
- Project Demonstration: Kevin demonstrates how a conversation looks with and without translation tools. His project aims to maintain ASL as the primary language throughout the conversation.
- Technological Solutions: Kevin discusses the complexities and solutions for his project. He used Google MediaPipe and Point Cloud classification models to enhance accuracy, consistency, and real-time processing.
- Receptive and Expressive Technology: The receptive part translates ASL into English, while the expressive part translates English into ASL. He highlights challenges like vocabulary size and proposes using semantic search to find contextually similar signs.
- Future Improvements: Kevin plans to improve accuracy and speed, incorporate more parameters of ASL, and develop consumer applications like a Chrome extension for YouTube captions and a webcam client for ASL interpretation in real-time.
- Acknowledgments and Motivation: He thanks his teachers and mentors, noting that learning ASL and understanding Deaf culture motivated him to work on this project.
- Open Source and Community Engagement: Kevin invites others to contribute to his open-source project and join a community of high school developers.
The project showcases the potential for AI and machine learning to create more inclusive communication tools for the Deaf community.
FDA approves new cancer treatment for patients with solid tumors, Amtagvi
Original Article Title:
A watershed moment for cancer therapies has arrived
Source: Axios
Date: Feb 21, 2024
Key Takeaway
The FDA’s accelerated approval of a new cancer treatment, Amtagvi, marks a significant milestone in cancer therapy, offering hope for patients with solid tumors.
Summary
- A new class of cancer treatments, tumor-infiltrating lymphocytes (TIL), utilizes the body’s immune system to combat tumors.
- While CAR-T therapy has been successful in some blood cancers, TIL therapy targets solid tumors, which constitute 90% of cancer types.
- The FDA granted accelerated approval to Iovance Biotherapeutics’ Amtagvi, a melanoma therapy utilizing TILs, indicating a breakthrough in cancer therapy.
- TIL therapy involves extracting T-cells from tumors, strengthening them, and infusing them back into patients, resulting in a long-lasting defense against cancer.
- Clinical trials have shown promising results, with response rates ranging from 31.5% to 56% among patients with melanoma.
- Several companies are conducting trials for TIL therapy targeting various cancers, including melanoma, lung, ovarian, and kidney cancers.
- Affordability and accessibility remain concerns due to the high cost of TIL therapy, priced at $515,000, and the resource-intensive manufacturing process.
- Despite challenges, the accelerated approval is expected to make TIL therapy more widely available, with plans to establish treatment centers across the US.
- Future research aims to extend the application of TIL therapy to other tumor types, promising further advancements in cancer treatment.
OpenAI Expands Healthcare Push With Color Health’s Cancer Copilot
Original Article Title:
OpenAI Expands Healthcare Push With Color Health’s Cancer Copilot
Source: WSJ
Date: 17 June 2024
OpenAI has partnered with Color Health to introduce a new AI-powered tool called Cancer Copilot. This initiative aims to assist cancer patients with personalized healthcare guidance and support.
Key Points:
- OpenAI collaborates with Color Health on the Cancer Copilot.
- The AI tool provides personalized health advice for cancer patients.
- The initiative expands OpenAI’s presence in the healthcare sector.
- The tool aims to improve patient outcomes and streamline treatment processes.
Why This Matters: This collaboration leverages AI to offer innovative solutions in healthcare, potentially transforming cancer care by providing tailored support and improving patient management.
Lexi Bogan, who lost her voice due to a brain tumor, now uses OpenAI’s Voice Engine to speak again
Original Article Title:
Illness took away her voice. AI created a replica she carries in her phone
Source: AP
Date: 14 May 2024
Lexi Bogan, who lost her voice after a brain tumor surgery, has regained her ability to speak using OpenAI’s Voice Engine, a voice-cloning technology. This innovation, trained on a 15-second clip of her high school voice, allows her to communicate with a synthetic voice that closely mimics her original tone. While AI voice-cloning technology raises concerns about misuse, Bogan’s case highlights its positive impact, offering a significant improvement in her quality of life and serving as an inspiration for future applications in speech recovery for other patients
How AI is Diagnosing and Treating Mental Health Disorders
Original Article Title:
Artificial intelligence in positive mental health: a narrative review
Source: National Library of Medicine
Date: 18 March 2024
Key Takeaway: AI is transforming mental health care by enhancing diagnosis, treatment, and emotional regulation, with machine learning, natural language processing (NLP), and reinforcement learning playing significant roles. Despite its potential, ethical issues like privacy, biases, and the need for human oversight persist.
Key Points:
- AI in Diagnosis and Treatment:
- AI aids in diagnosing mental health conditions such as depression, anxiety, and schizophrenia. Machine learning models analyze patient data to recommend tailored treatments. In schizophrenia, AI can detect early speech pattern changes indicating symptoms.
- Personalized treatment recommendations are made based on individual characteristics. For example, AI chatbots can engage patients in conversations and suggest behavioral interventions based on emotional state monitoring.
- Deep learning techniques analyze brain scans to identify early markers of neurodegenerative disorders like Alzheimer’s and Parkinson’s.
- AI in Emotional and Cognitive Health:
- AI-powered applications help in emotional regulation by offering real-time interventions. AI-driven emotion-sensing systems use facial expressions, voice, and physiological data to assess emotional states.
- For emotional dysregulation, AI assists in guiding individuals through cognitive-behavioral exercises, improving emotional intelligence, and managing stress or depression symptoms. AI tools, such as virtual therapists and biofeedback systems, are used to teach emotional regulation skills.
- Cognitive impairments are managed by AI systems that improve diagnosis accuracy. Machine learning techniques like support vector machines (SVM) and neural networks are used in cognitive assessments for early detection.
- AI for Specific Disorders:
- Autism Spectrum Disorder (ASD): AI analyzes video interactions, facial expressions, and gestures to detect early signs of ASD. This helps clinicians identify and intervene earlier in a child’s development.
- Schizophrenia: AI tracks speech patterns to detect subtle signs of schizophrenia. Predictive models can foresee relapses based on emotional or linguistic changes.
- Seizures: Machine learning processes electroencephalography (EEG) recordings to detect seizure patterns, helping predict and manage epileptic episodes.
- Ethical Considerations and Challenges:
- Privacy and Data Security: Handling sensitive data such as patient health records requires strict privacy measures.
- Bias in AI Algorithms: AI systems trained on biased data can perpetuate inequities in diagnosis and treatment, especially for minority or underrepresented populations.
- Human Oversight: AI in mental health should support, not replace, human professionals. Maintaining a balance between AI-generated recommendations and human judgment ensures responsible care.
- Patient Trust and Transparency: AI systems need to be transparent in their decision-making processes to build trust among users and clinicians.
Why This Matters: AI’s role in mental health is expanding rapidly, offering more accurate diagnoses, personalized treatments, and accessible emotional regulation tools. As AI continues to evolve, collaboration between mental health professionals and AI experts will be essential to ensure it serves humanity without compromising ethical standards.
Healthcare Operations
The UK government is leveraging AI technology to cut cancer waiting lists
Original Article Title:
AI technology to help cut cancer waiting lists
Source: GOV.UK
Date: 21 May 2024
The UK Government has announced it will roll out AI technology to all radiography departments in England to help cut cancer waiting lists. This game-changing technology, backed by £15.5 million in new funding, can locate cancer cells 2.5 times quicker than doctors alone. It automatically reviews CT or MRI scans, allowing doctors to quickly identify cancerous cells and prevent healthy organs from being damaged during radiation treatment. This innovation is expected to benefit tens of thousands of cancer patients each year, reducing waiting times and improving treatment outcomes.
The World Health Organization (WHO) has launched the S.A.R.A.H. (Stay Aware, Respond, Act, Help) campaign to enhance public awareness and preparedness for health emergencies.
Original Article Title:
Meet S.A.R.A.H, a Smart AI Resource Assistant for Health
Source: World Health Organization
Date: –
The World Health Organization (WHO) has launched the S.A.R.A.H. (Stay Aware, Respond, Act, Help) campaign to enhance public awareness and preparedness for health emergencies. This initiative aims to provide clear, actionable information on how individuals and communities can respond to various health crises, including pandemics and natural disasters. By leveraging digital platforms and community engagement, S.A.R.A.H. focuses on building resilience and promoting health security worldwide.
Doccla Raises $46M to Expand 'Hospital at Home' Tech Across Europe
Original Article Title:
‘Hospital at home’ startup Doccla raises $46 million for its European expansion
Source: TechCrunch
Date: 2 September 2024
Key Takeaway:
Doccla is a European startup providing “hospital at home” technology that enables remote patient monitoring, helping alleviate hospital bed shortages. Using a combination of devices and a mobile app, the platform allows doctors to track patient health data in real-time, reducing the need for in-person visits while maintaining high-quality care.
Key Points:
- Remote Patient Monitoring: Doccla provides monitoring devices and a mobile app that collect health data from patients at home. The data integrates with electronic health records, allowing doctors to monitor patient conditions via a clinician dashboard and receive alerts when issues arise. This setup helps free up hospital beds for those in more urgent need.
- Virtual Hospitals: By focusing on “virtual beds,” Doccla’s technology supports early discharge patients and those who can avoid hospitalization entirely. The platform allows healthcare providers to remotely manage patients, offering peace of mind to both overworked medical staff and patients recovering at home.
- Device-Agnostic and User-Friendly Interface: Doccla emphasizes accessibility, offering large-font displays, simple navigation, in-app messaging, and video call capabilities. These features ensure patients of varying tech-literacy levels can use the system effectively, while doctors can stay connected with them remotely.
- Pharmaceutical Trials Integration: In addition to healthcare services, Doccla’s technology is being used in pharmaceutical clinical trials. The platform reduces or eliminates the need for trial participants to attend clinics in person, making the process faster, more efficient, and cost-effective compared to traditional research methods.
- Geographic Expansion: After gaining traction in the U.K. and Ireland, Doccla is expanding its services into other European markets, including Germany, Austria, and France. The company’s approach to entering new markets involves securing partnerships with healthcare providers before launching in a region, ensuring that it meets local healthcare needs and reimbursement models.
- Future Growth and European Expansion: The company recently raised $46 million in funding, which it plans to use for expanding across Europe, hiring local teams, and advancing its technology. Doccla’s business model is focused on practical growth, entering new markets with the support of signed customers before establishing operations there.
Why This Matters:
Doccla’s “hospital at home” technology addresses pressing healthcare challenges like hospital bed shortages by allowing remote patient management, offering a safer, more efficient alternative to traditional hospitalization. Its versatility in both clinical care and pharmaceutical research presents an opportunity for significant impact in healthcare delivery across Europe.
Google's medical AI destroys GPT's benchmark and outperforms doctors
Original Article Title:
Google’s medical AI destroys GPT’s benchmark and outperforms doctors
Source: New Atlas
Date: 6 May 2024
Google’s Med-Gemini AI, a highly specialized medical AI, has shown impressive capabilities in clinical diagnostics, surpassing existing benchmarks and even outperforming doctors in certain assessments. Utilizing self-training and web search tools, Med-Gemini achieved notable accuracy in complex medical scenarios, such as the USMLE. Its advanced multimodal functionalities allow it to process and reason from diverse data inputs, pushing the boundaries of medical AI applications.
Large language models (LLMs), particularly GPT-4, demonstrate significant advancements in ophthalmological knowledge and reasoning, reaching a level comparable to or exceeding that of expert ophthalmologists
Original Article Title:
Large language models approach expert-level clinical knowledge and reasoning in ophthalmology: A head-to-head cross-sectional study
Source: PLOS Digital Health
Date: 17 April 2024
Large language models (LLMs), particularly GPT-4, demonstrate significant advancements in ophthalmological knowledge and reasoning, reaching a level comparable to or exceeding that of expert ophthalmologists and ophthalmology trainees.
Key Points
- Introduction:
- LLMs like GPT-3.5 and GPT-4 are trained on extensive datasets to process natural language.
- LLMs have shown promise in medical contexts, including answering medical queries and passing medical examinations.
- Methods:
- FRCOphth Part 2 questions were used to assess LLMs’ ophthalmological knowledge and reasoning.
- Questions were categorized based on subject and type.
- Performance of LLMs was compared to expert ophthalmologists, ophthalmology trainees, and junior doctors.
- Results:
- GPT-4 outperformed GPT-3.5, LLaMA, and PaLM 2 in ophthalmology knowledge and reasoning.
- GPT-4’s performance was statistically similar to expert ophthalmologists and exceeded that of ophthalmology trainees and junior doctors.
- Agreement between LLMs and human doctors was generally weak, reflecting differences in knowledge and reasoning.
- Discussion:
- LLMs like GPT-4 show potential in providing medical advice and assistance, especially where access to expert ophthalmologists is limited.
- Further research is needed to explore the clinical deployment of LLMs in healthcare settings.
- Conclusion:
- State-of-the-art LLMs, particularly GPT-4, demonstrate expertise in ophthalmological knowledge and reasoning, suggesting their potential utility in clinical practice.
K Health is revolutionizing healthcare by integrating AI-powered chatbots into primary and urgent care
Original Article Title:
K Health’s AI Revolution in Healthcare: A New Era of Healthcare
Source: The AI Track
Date: 30 July 2024
Summary:
K Health is revolutionizing healthcare by integrating AI-powered chatbots into primary and urgent care, making medical diagnostics and treatment more efficient. Founded to counteract the overwhelming and often misleading medical information available online, K Health’s AI-driven platform assists doctors by analyzing patient symptoms and histories to suggest possible conditions. This approach helps healthcare professionals make more informed decisions and reduces the administrative burden on clinicians.
Key Points:
- AI as a Collaborative Tool: K Health’s AI-powered chatbot functions as a collaborative assistant to doctors rather than a replacement. It processes patient data and medical histories, compares them to a comprehensive database, and suggests potential diagnoses. The chatbot gathers essential information, allowing doctors to focus on diagnosis and treatment, while human expertise finalizes medical decisions.
- Data-Driven AI Foundation: K Health’s AI foundation is built on robust data, initially sourced from a vast dataset licensed from Israel’s Maccabi Health Insurance spanning over two decades. This data enables the AI to predict the “next best question” for accurate diagnosis. K Health’s AI technology aims to reduce human error and misdiagnoses, making healthcare more accessible and efficient by reducing the administrative workload of clinicians.
- Expanding Influence and Collaborations: K Health’s platform has reached over 3.1 million patients across 48 states, offering services that range from primary care to chronic disease management. The company has formed significant partnerships, including a $59 million investment collaboration with Cedars-Sinai to embed their AI technology into the hospital’s care system. K Health is also working with Mayo Clinic on personalized hypertension treatments and has partnered with Anthem and Blackstone Group to expand access to affordable healthcare.
- Global Market and Future Outlook: The global AI healthcare market is expected to grow significantly, from USD 15.1 billion in 2022 to an estimated USD 187.95 billion by 2030. This growth is driven by the increasing demand for AI-powered healthcare solutions to improve outcomes and reduce costs. K Health exemplifies how AI can enhance healthcare delivery by making care more personalized, efficient, and accessible.
- Challenges and Ethical Considerations: While AI offers tremendous benefits, challenges such as data privacy, potential biases in AI algorithms, and the need to ensure equitable healthcare access remain critical concerns. Efforts are being made to address these issues, such as the RAISE-Health initiative by Stanford Medicine, which focuses on responsible and equitable AI deployment in healthcare.
Why It Matters: K Health’s integration of AI in healthcare is a significant step towards more efficient, accurate, and accessible medical care. The company’s innovations represent the broader potential of AI to transform the healthcare landscape, from reducing misdiagnoses to empowering patients to take control of their health.