AI Breakthroughs in Technology - At a Glance

AI is at the forefront of technological and infrastructural advancements. Discover the most impactful AI breakthroughs in technology: innovations that are shaping the future of technology and the infrastructure that supports modern life.
Each section offers insights into how AI is transforming different sectors, providing a comprehensive view of its impact across a wide range of disciplines.
The Essential Guide to AI Infrastructure: All you need to know
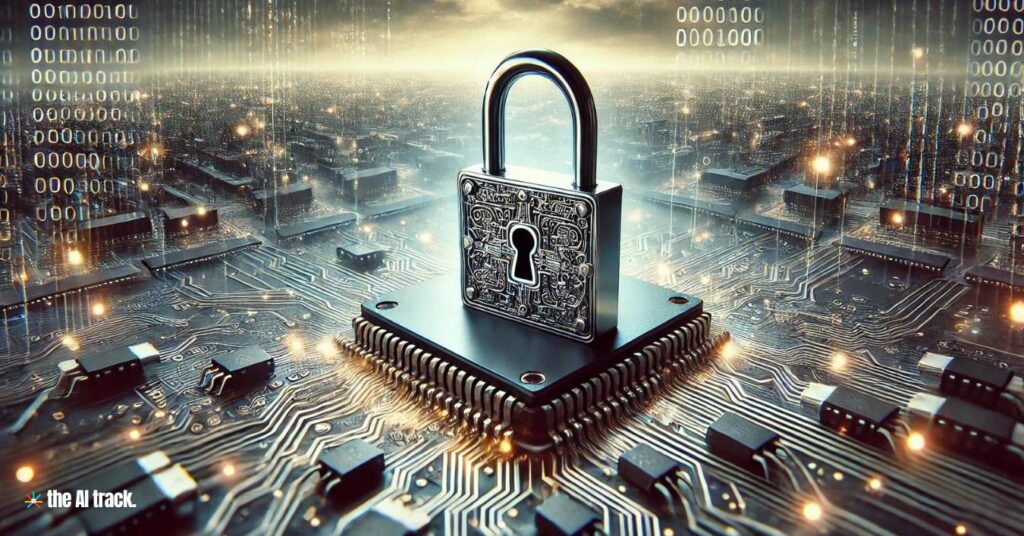
The AI Track’s extensive analysis of the critical components of AI infrastructure, including hardware, software, and networking, that are essential for supporting AI workloads. The article also examines the benefits, challenges, and emerging trends in AI infrastructure.
Scientists Claim AI Breakthrough to Generate Boundless Clean Fusion Energy
Original Article Title:
Scientists Claim AI Breakthrough to Generate Boundless Clean Fusion Energy
Source: Vice
Date: 21 February 2024
Princeton researchers have developed an AI model that predicts and prevents plasma instability in fusion reactors, a significant breakthrough in the pursuit of clean fusion energy.
Summary
- Fusion energy faces numerous challenges, including generating more energy than consumed, reactor-proof materials, impurity control, and plasma containment.
- Princeton researchers created an AI model to address plasma instability, a critical issue in fusion reactors.
- The AI model predicts tearing mode instabilities, allowing for intervention before disruptions occur.
- Tested on the DIII-D National Fusion Facility, the AI-controlled reactor successfully maintained plasma stability.
- The AI model was trained on real data from fusion experiments, learning optimal pathways to maintain high-powered reactions while avoiding instabilities.
- Fusion reactors face various instability challenges, but tearing mode instabilities are a major obstacle.
- AI is expected to play a significant role in controlling and optimizing fusion reactions.
- The study is a proof-of-concept, with potential applications in other reactors and energy optimization.
AI Technology Spots Damaged Power Line Parts with 90% Accuracy
Original Article Title:
Detection of Missing Insulator Caps Based on Machine Learning and Morphological Detection
Source: MDPI
Date: 31 January 2023
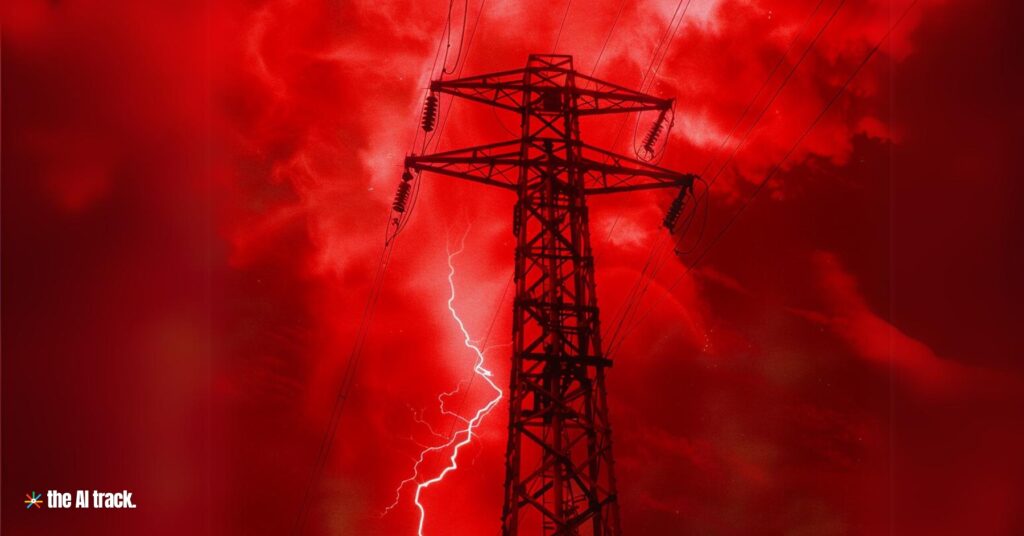
Key Takeaway:
Researchers from Dongguan University of Technology have developed a machine learning-based method to detect missing insulator caps in glass and porcelain insulators used in high-voltage transmission lines. The approach uses a combination of Support Vector Machine (SVM) classification and morphological detection to identify insulators and determine if caps are missing, providing an efficient solution for power line inspections.
Key Points:
- Machine Learning-Based Detection: The method applies SVM, a machine learning algorithm, to detect missing insulator caps by extracting grayscale and color features from images captured by Unmanned Aerial Vehicles (UAVs). This approach enhances accuracy in locating and identifying faulty insulators.
- High Accuracy: The proposed method achieves over 90% accuracy, recall, and average precision, making it suitable for high-voltage transmission line inspections. It is particularly effective for glass and porcelain insulators, which are prone to damage due to environmental conditions like wind and acid rain.
- Morphological Processing: The system uses morphological image processing to assess the condition of insulators, focusing on shape and texture features. This allows for the detection of missing caps and helps quantify the degree of insulator damage.
- UAV Integration for Real-Time Monitoring: UAVs equipped with the detection system can transmit fault data in real time, reducing the need for large data transfers to workstations and making the solution practical for real-time online detection.
- Noise Resilience and Angle Adjustments: The method is designed to withstand noise in the images and can handle different shooting angles by adjusting the UAV’s position to capture optimal “key frames” for better detection accuracy.
- Practical Application: The detection system supports power supply enterprises by identifying damaged insulators and providing data on the number of remaining insulator caps. This allows for timely replacement decisions, improving safety and efficiency in power transmission maintenance.
Why This Matters:
The development of this machine learning-based detection system represents a significant advancement in the automated inspection of power lines. By ensuring accurate identification of missing insulator caps, this technology enhances the reliability of power transmission systems, reducing the risk of accidents. The integration of UAVs and AI ensures that inspections are more efficient, less labor-intensive, and provide real-time insights into transmission line conditions.
Researchers have developed a new type of magnet that does not rely on rare-earth metals
Original Article Title:
A Radical New Magnet Without Rare-Earth Metals Is About to Change Motors Forever
Source: Popular Mechanics
Date: 18 June 2024
Key Takeaway: Researchers have developed a new type of magnet using AI that does not rely on rare-earth metals, potentially reducing environmental and geopolitical issues.
Key Points:
- The magnet is composed of iron and nickel, eliminating the need for rare-earth elements.
- AI was instrumental in identifying the optimal composition and structure of the magnet.
- This innovation could impact industries reliant on magnets, such as renewable energy and electronics, by providing a more sustainable and cost-effective alternative.
Why This Matters: The development of rare-earth-free magnets can mitigate environmental damage and reduce reliance on geopolitically sensitive materials, promoting sustainable technological advancements.
Explore the U.S. government’s strategic push in semiconductor manufacturing, featuring TSMC’s and Samsung’s major investments and technological leaps.
AI is Helping Discover Stronger, More Flexible Materials
Original Article Title:
A Radical New Magnet Without Rare-Earth Metals Is About to Change Motors Forever
Source: Science Advances
Date: 2 February 2024
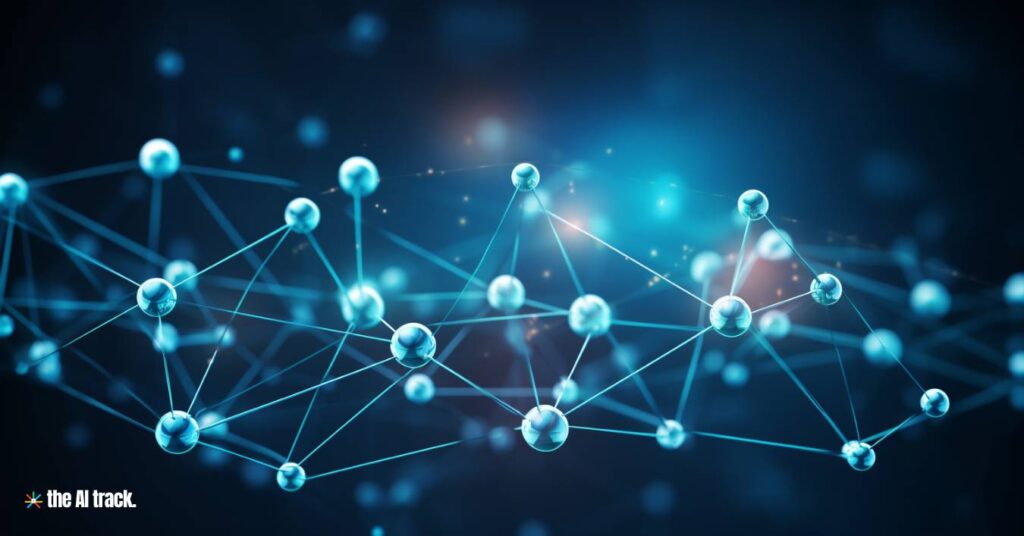
Key Takeaway:
Researchers have developed a cutting-edge AI-powered computational pipeline that discovers microstructured composites with optimal stiffness and toughness trade-offs. This breakthrough, led by a team from MIT, addresses a long-standing challenge in engineering material design, leveraging neural networks and simulations to efficiently find ideal material structures without prior expert knowledge.
Key Points:
- Stiffness-Toughness Trade-Off: Stiffness and toughness are key material properties in engineering, but they typically conflict. This new computational pipeline overcomes the challenge by finding microstructured composites that offer the best balance between these two properties.
- AI and Simulation Integration: The system integrates physical experiments, numerical simulations, and artificial neural networks. A nested-loop proposal-validation approach accelerates the discovery process, allowing neural networks to act as surrogate models for faster simulation and optimization.
- Efficient Material Discovery: The pipeline efficiently narrows down materials with optimal properties, running far fewer simulations compared to traditional methods. This approach drastically improves sample efficiency, finding experimentally validated designs after only a few dozen physical tests.
- Advanced AI Techniques: The research team applied convolutional neural networks (CNNs) and a multi-objective optimization algorithm (NMO) to navigate the design landscape, making the discovery process faster and more accurate.
- Applications Beyond Mechanics: While focused on solid mechanics, the methodology has broad potential, extending into fields such as polymer chemistry, fluid dynamics, and even robotics, providing a blueprint for computational design across multiple disciplines.
- Real-World Testing: The materials were fabricated using advanced 3D printing techniques, and their physical properties were tested for strength and flexibility. The simulations closely matched experimental results, validating the AI-driven approach.
- Future Automation: The team aims to further streamline and scale the process, envisioning fully automated laboratories where material discovery, testing, and refinement can be done with minimal human intervention.
Why This Matters:
This breakthrough showcases how AI and computational simulations can revolutionize material science, discovering high-performance materials with unprecedented efficiency. The integration of AI into material design enables the discovery of composites that are both stiff and tough, unlocking new possibilities for applications in automotive, aerospace, and other industries. The methodology can also be adapted to a wide range of scientific fields.
AI Is Helping U.S. Water Utilities Detect Leaks and Improve Quality
Original Article Title:
Adoption of Artificial Intelligence in Drinking Water Operations: A Survey of Progress in the United States
Source: ASCE Library
Date: 12 May 2023
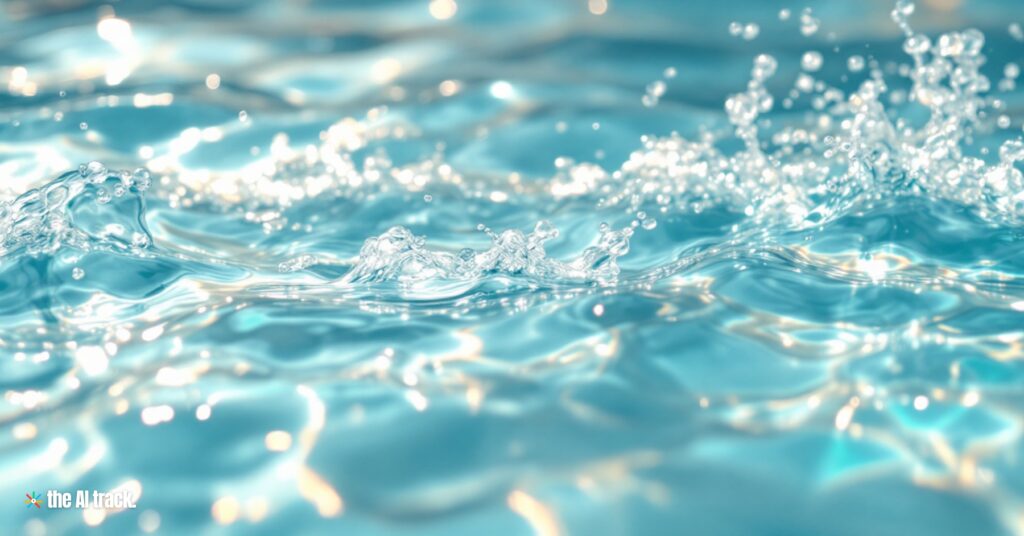
Key Takeaway:
Artificial Intelligence (AI) offers significant potential to optimize drinking water utility operations by improving leak detection, water quality monitoring, and system automation. Despite its promise, AI adoption in the U.S. water industry remains limited, with only 24% of large utilities having experimented with AI technology. Most implementations are still in experimental or manual phases, rather than fully integrated systems.
Key Points:
- AI Usage in Water Utilities: Only 12 of 49 surveyed water utilities in the U.S. have implemented AI, representing 24%. These utilities are experimenting with AI for tasks like leak detection, water quality improvement, and automating complex processes. However, full-scale adoption is still rare, and AI usage remains in early experimental stages rather than fully integrated solutions.
- Planned AI Adoption: Of the 76% of respondents who have not yet used AI, 68% are open to adopting the technology in the next five years. Their interest centers on AI’s potential to enhance leak detection, predict water demand, and optimize infrastructure like water main replacements.
- Motivations for Using AI: The primary motivators for AI adoption include saving money, detecting leaks, and improving water quality. Other drivers include energy savings, enhanced water conservation, and integration with existing technologies like Supervisory Control and Data Acquisition (SCADA) systems.
- Barriers to Adoption: Major barriers to AI adoption include concerns over financial investments, lack of AI expertise, and uncertainties about payback. Some water utilities are hesitant to adopt AI due to the high costs of implementation and challenges in finding qualified personnel to manage these systems.
- Human Element Remains Critical: Respondents emphasized that AI should complement rather than replace human operators. Water utilities continue to rely on experienced staff to manage critical systems, and AI’s role is seen as assisting in non-critical, data-heavy tasks. There is skepticism about allowing AI to make crucial operational decisions, especially in emergency situations.
- Future Research and Development Needs: The study recommends more collaboration between researchers and water utilities to create convincing case studies for AI implementation. Leak detection appears to be a high-potential area for future research, but issues such as staff expertise and return on investment need to be addressed to encourage broader AI adoption.
Why This Matters:
As water utilities become more complex due to urbanization, climate challenges, and increasing regulatory demands, AI holds promise for optimizing operations and improving sustainability. However, without overcoming barriers like cost and expertise, widespread adoption of AI in the water sector may be slow. Researchers and utility operators need to collaborate more closely to demonstrate AI’s tangible benefits and ensure its responsible integration into essential water systems.
Microsoft’s MAIA-100: A Breakthrough in Generative AI and Computing Power
Original Article Title:
Microsoft introduces Maia 100
Source: The AI Track
Date: 1 August 2024
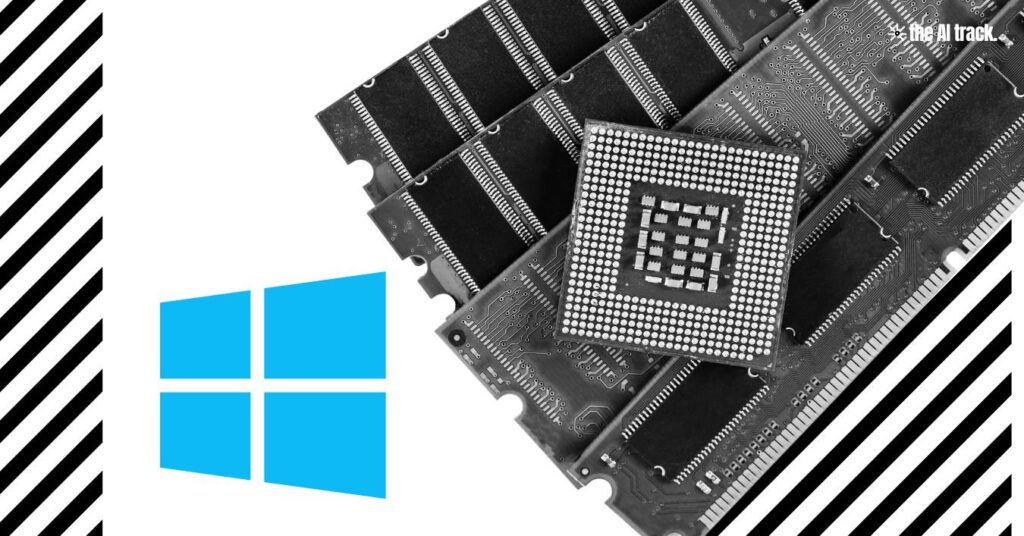
Summary:
Microsoft’s MAIA-100 represents a significant leap in the field of generative AI, combining advanced computing power with innovative AI models designed to transform industries.
Key Points:
- MAIA-100’s Core Capabilities: The MAIA-100 platform is designed to power a new generation of AI applications by delivering unprecedented computational speed and efficiency. This hardware-software synergy enables generative AI models to handle complex tasks, such as natural language processing, image generation, and real-time decision-making, with increased precision and speed.
- AI-Powered Revolution in Multiple Sectors: Microsoft envisions MAIA-100 revolutionizing various sectors, including healthcare, finance, manufacturing, and creative industries. In healthcare, MAIA-100’s AI models can accelerate drug discovery and personalized treatment plans, reducing the time and cost associated with developing new therapies. In finance, the platform can enhance risk assessments, fraud detection, and automated trading strategies. Creative industries benefit from the platform’s ability to generate high-quality visuals, music, and other content rapidly, streamlining production workflows.
- Efficiency and Sustainability Focus: One of the standout features of MAIA-100 is its emphasis on sustainability and energy efficiency. The platform is designed to reduce energy consumption without compromising on performance, addressing one of the significant challenges of AI computing.
- Collaboration with Industry Leaders: Microsoft has partnered with industry leaders and research institutions to refine the MAIA-100 platform further. These collaborations aim to expand the platform’s capabilities and explore new applications across different fields.
- Driving the Future of AI with Scalability and Security: Scalability and security are at the forefront of MAIA-100’s design, ensuring that businesses can scale their AI operations as needed without compromising data integrity.
Why It Matters: MAIA-100 represents a pivotal advancement in generative AI, pushing the boundaries of what AI can achieve across various industries. By combining powerful computing capabilities with innovative AI models, Microsoft’s platform is set to redefine how businesses leverage AI for enhanced decision-making, creativity, and operational efficiency.
What Are AI Chips and Why Do They Matter
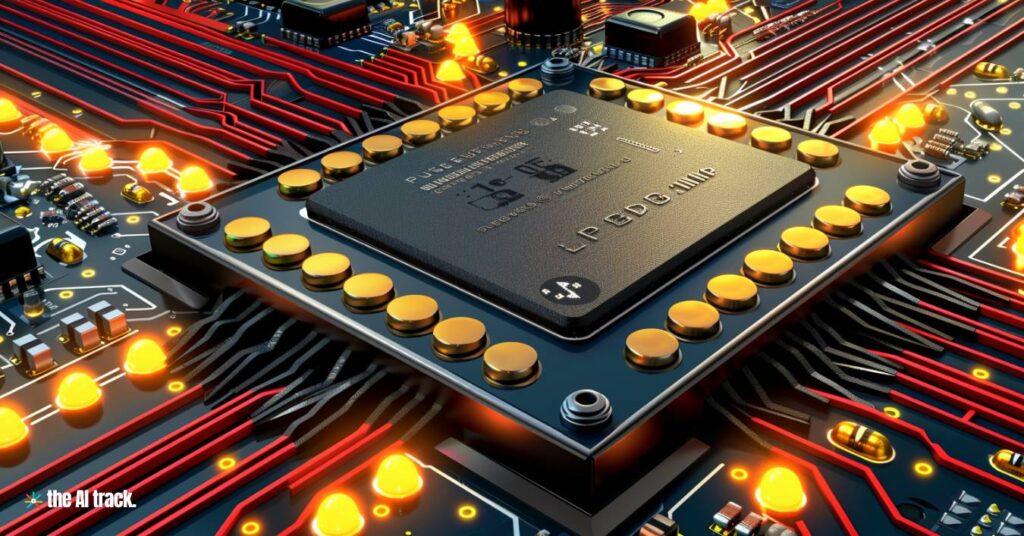
The rise of AI has ignited an insatiable demand for cutting-edge AI chips capable of fueling revolutionary advancements in fields like autonomous vehicles and natural language processing.
IBM has launched the world’s largest quantum computer, IBM Condor
Original Article Title:
IBM Condor: The Quantum Computing Breakthrough
Source: The AI Track
Date: 1 August 2024
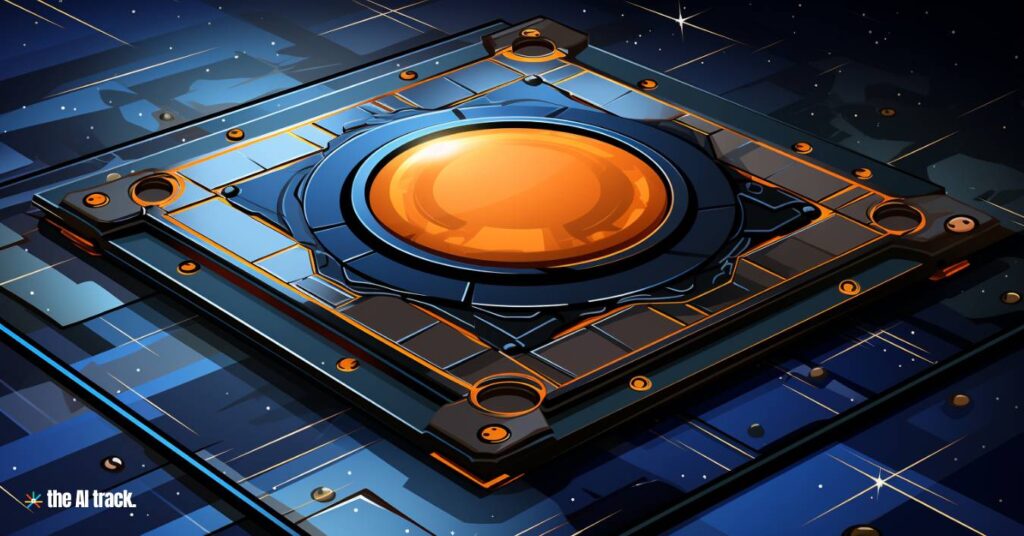
Summary:
IBM has launched the world’s largest quantum computer, IBM Condor, with 1,121 qubits, marking a significant leap in quantum computing. This milestone moves quantum technology from experimental to practical, enabling solutions to complex problems beyond classical computing capabilities.
Key Points:
- IBM Condor’s Breakthrough: IBM Condor, the first quantum computer to exceed 1,000 qubits, signals the readiness of quantum technology for real-world applications, allowing previously unimaginable computations.
- Revolutionizing Various Fields: Condor’s power will transform industries like cryptography, material science, and pharmaceuticals, optimizing complex tasks like molecular simulations and logistics.
- Scalability and Practical Use: Condor’s scalable design sets the stage for larger systems, integrating quantum computing into existing infrastructures to solve complex problems.
- Quantum Advantage: Condor is key to achieving quantum advantage, outperforming classical computers in tasks like portfolio optimization and fraud detection.
- Technical Innovations: Overcoming challenges in error correction and stability, Condor advances the reliability of quantum computing for scientific and industrial applications.
- Future Prospects: Condor is part of IBM’s roadmap to develop even more powerful quantum systems, integrating quantum with classical computing for enhanced efficiency.
- Sustainable Development: Quantum computing, as exemplified by Condor, could drive advancements in energy efficiency, climate modeling, and sustainable agriculture.
Why It Matters: IBM Condor represents a future where quantum computing drives innovation, reshaping how industries tackle complex challenges.
New Algorithm Could Slash Energy Use by 95%
Original Article Title:
Integer Addition Algorithm Could Reduce Energy Needs of AI by 95%
Source: TechXplore
Date: 13 September 2024
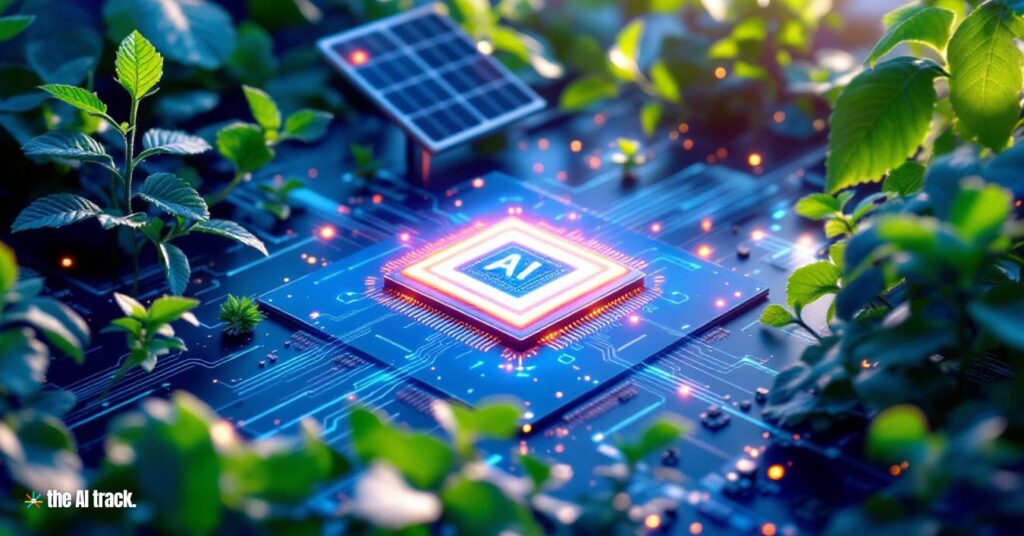
Key Takeaway:
BitEnergy AI has developed a new method called Linear-Complexity Multiplication that replaces energy-intensive floating-point multiplication with integer addition, reducing AI applications’ energy needs by 95%.
Key Points:
- Energy Consumption: AI models like ChatGPT consume immense amounts of energy—around 564 MWh per day, equivalent to powering 18,000 homes.
- New Method: The new technique replaces floating-point multiplication with integer addition, significantly lowering energy demand without reducing performance.
- Hardware Requirements: Adoption requires different hardware, but prototypes have already been built and tested.
- Impact: Nvidia’s response to this innovation could impact market adoption.
Why This Matters:
The reduction in energy usage can lead to more sustainable AI operations, lowering costs and environmental impacts.
Brain on a Chip: Boosting AI Efficiency by 460x!
Original Article Title:
New Brain-on-a-Chip Platform Promises 460x Efficiency Boost for AI Tasks
Source: NetworkWorld
Date: 13 September 2024
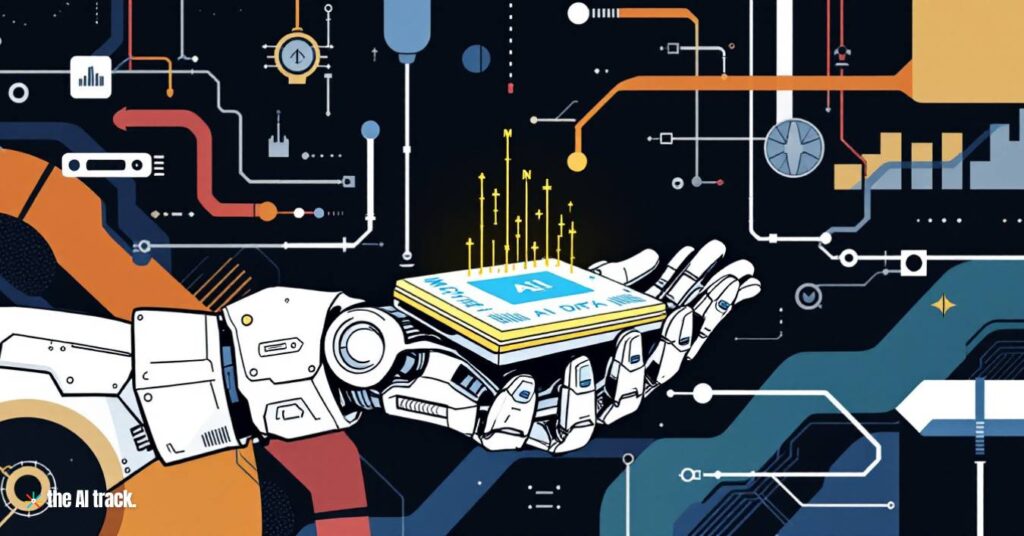
Key Takeaway:
The Indian Institute of Science (IISc) has developed a groundbreaking neuromorphic computing platform that dramatically enhances energy efficiency and processing speed for AI tasks. This brain-inspired technology, capable of handling 16,500 conductance states, is set to revolutionize AI hardware by working alongside existing systems to achieve unprecedented performance improvements.
Key Points:
Neuromorphic Computing Innovation:
The new platform developed by IISc mimics the human brain’s structure and processes, allowing it to store and process data across 16,500 conductance states in a molecular film. This is a significant leap from traditional digital systems, which are limited to binary states (on and off).
Efficiency and Speed Improvements:
The platform reduces the steps required for core AI operations, such as vector-matrix multiplication, from n² steps to just one, greatly boosting speed and energy efficiency. It delivers 4.1 TOPS/W, making it 460 times more efficient than an 18-core Haswell CPU and 220 times more efficient than an Nvidia K80 GPU, commonly used in AI workloads.
Potential Applications:
The platform’s ability to process complex data with high precision (14-bit accuracy) makes it ideal for advanced AI tasks, including machine learning, data analysis, and robotics. It is also expected to enhance brain-computer interfaces by accurately decoding specific brain patterns related to movements or mental states.
Integration with Existing AI Hardware:
Unlike many emerging technologies, IISc’s neuromorphic platform is designed to work alongside existing AI systems rather than replace them. It is particularly suited to offloading repetitive tasks like matrix multiplication, complementing the strengths of GPUs and TPUs to create faster, more efficient AI models.
Developing Indigenous Neuromorphic Chips:
IISc is advancing towards creating a fully indigenous neuromorphic chip supported by India’s Ministry of Electronics and Information Technology. This effort covers the complete development cycle from materials to systems, positioning the platform as a crucial home-grown technology.
Demonstrated Capabilities:
The team has already showcased the platform’s power by recreating NASA’s “Pillars of Creation” image from the James Webb Space Telescope on a tabletop computer, achieving results that would traditionally require a supercomputer, but with much less time and energy.
Impact on Future AI Hardware:
As AI tasks become increasingly demanding, traditional silicon-based processors are reaching their limits. IISc’s platform offers a new path forward, leveraging brain-inspired analog computing to deliver faster, more energy-efficient solutions, potentially transforming industries such as cloud computing and autonomous systems.
Why This Matters:
IISc’s neuromorphic platform represents a significant technological breakthrough in AI hardware, offering vast improvements in energy efficiency and computational speed. By integrating this new technology with existing AI systems, the platform has the potential to drive future advancements in AI, making high-performance AI applications more accessible and sustainable.
Voices of AI: Insights and Quotes from the Top’ offers a unique compilation of quotes and perspectives from top AI executives and thinkers.
IBM & UNDP Launch AI Tools to Empower Global Energy Decisions
Original Article Title:
UNDP and IBM Launch New Tools to Forecast Energy Access and Model Energy Equity
Source: IBM
Date: 17 September 2024
Key Takeaway:
IBM and the United Nations Development Programme (UNDP) have introduced two groundbreaking models—Electricity Access Forecasting and the Clean Energy Equity Index—through UNDP’s GeoHub platform. These tools leverage advanced AI and geospatial data to support global energy equity, offering insights that can inform policymakers and promote a fair transition to clean energy.
Key Points:
Electricity Access Forecasting Model:
This AI-powered model, built using IBM’s watsonx platform, IBM Cloud, and satellite data, forecasts electricity access across 102 countries in the Global South. It evaluates factors like population, infrastructure, and land use to predict energy availability through 2030. Unlike current-day estimates, this model provides a forward-looking perspective on electricity access, especially in Africa, Asia Pacific, Latin America, and the Middle East.
Clean Energy Equity Index (CEEI):
Developed in collaboration with Stony Brook University, this geospatial model combines environmental, economic, and social factors to generate a Clean Energy Equity score, ranging from 0 to 1. The score assesses opportunities for clean energy development and highlights areas where a just transition is most urgent. The CEEI covers 53 African countries, empowering users to evaluate factors like greenhouse gas emissions and wealth distribution.
Global Impact and Accessibility:
These models are freely available to the public via UNDP’s GeoHub platform, democratizing access to advanced energy data that traditionally wasn’t widely accessible. Policymakers, journalists, and organizations can now use this information to address clean energy challenges and accelerate efforts toward a just energy transition.
IBM Sustainability Accelerator Program:
This initiative emerged from IBM’s Sustainability Accelerator program, which supports global projects focused on sustainability issues like clean energy. Over the past two years, IBM and UNDP worked closely to develop and scale these tools, combining AI and data analytics to offer actionable insights for clean energy deployment.
Supporting Sustainable Development Goals (SDGs):
Both models contribute directly to the Sustainable Development Goals (SDGs), particularly around clean energy and reducing inequality. By providing data-driven insights, the tools aim to help countries make more informed decisions regarding energy equity and sustainability, promoting long-term resilience and environmental stewardship.
Why This Matters:
These AI and geospatial models represent a significant step forward in making complex energy data accessible to a wide range of stakeholders. They not only forecast future energy access but also highlight where clean energy efforts should be focused to ensure equity. This initiative plays a crucial role in supporting a global shift toward renewable energy, aligning with broader goals to combat climate change and promote sustainable development.
Our AI Books hub offers comprehensive guides to essential AI literature, providing key insights and actionable takeaways. Explore the transformative potential of AI through expertly summarized books.