Multiple academic studies now reinforce the claim that OpenAI’s GPT models, including GPT-4, memorized substantial portions of copyrighted content during training, raising high-stakes legal questions about AI data sourcing, model accountability, and the definition of “memorization” in the context of copyright law.
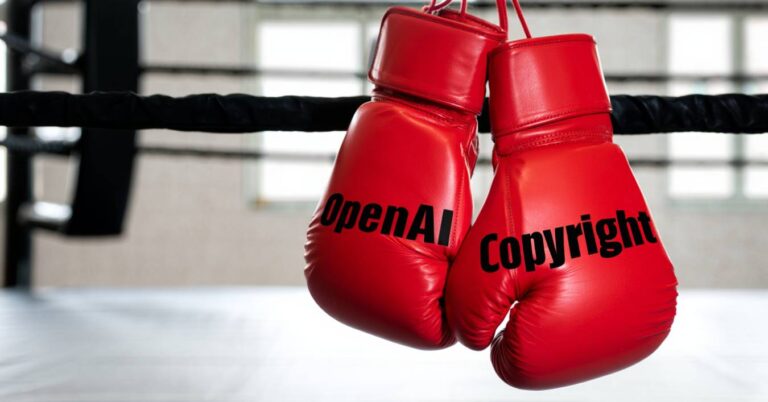
Study Proves ChatGPT Memorized Copyrighted Content – Key Points
Study by Top Institutions:
Researchers from the University of Washington, University of Copenhagen, and Stanford developed a method to assess whether large language models (LLMs) like OpenAI’s have memorized training data. The method centers on “high-surprisal” words—rare terms within sentences—to identify memorization through masked word prediction. The technique gained wide traction across research and social platforms, signaling its resonance beyond academia.
Testing GPT-4 and GPT-3.5:
The models were prompted to guess missing high-surprisal words in excerpts from fiction books and New York Times articles. Correct predictions indicated that the models had likely seen and retained those excerpts during training. GPT-4 performed notably well on these masked tests, suggesting memorization. The test dataset included material from BookMIA (a collection of copyrighted fiction) and journalistic content behind paywalls, such as New York Times articles.
Results Confirm Memorization:
The models’ success in these prediction tasks confirmed the presence of memorized content. GPT-4, in particular, reproduced near-verbatim phrases from protected literary works. Researchers emphasized that this behavior went beyond pattern recognition and statistical approximation—suggesting actual retention of source material.
Refined Definition of Memorization:
A companion legal-technical paper (“The Files are in the Computer,” arXiv:2404.12590) defined memorization as occurring when a model can reconstruct a near-exact copy of a substantial portion of its training data. This framework provides clearer boundaries for distinguishing legal liability under copyright law.
Differentiating Key Concepts:
The paper distinguishes:
Extraction: When users deliberately prompt the model to output copyrighted content.
Regurgitation: When the model does so unprompted.
Reconstruction: Any method that retrieves content, intentional or not.
These distinctions are central to upcoming legal debates about AI-generated content and its attribution.
Training Choices Determine Memorization:
Memorization is not an incidental byproduct but the consequence of deliberate training decisions, including dataset composition, model scale, and fine-tuning strategies. The amount and type of memorized content embedded in a model reflect its developmental philosophy and technical thresholds.
Implications for Copyright Lawsuits:
The findings bolster the legal cases brought by The New York Times, authors, and software developers against OpenAI and Microsoft. Plaintiffs argue that U.S. copyright law currently lacks a fair use exemption for model training. This undermines OpenAI’s reliance on “transformative use” defenses and places new legal focus on the nature of AI outputs.
Call for Transparency and Auditing Tools:
Abhilasha Ravichander, a lead researcher, stressed the urgency of developing standardized tools to probe and audit models post-training. Transparent reporting and inspection methods are essential to evaluating whether models comply with ethical and legal standards.
OpenAI’s Advocacy for Fair Use Reform:
OpenAI continues to lobby for global legislation that expands the fair use doctrine to cover AI training data. Despite licensing deals and opt-out mechanisms for rights holders, the company’s stance remains controversial in light of these findings. Past reports alleging use of proprietary materials (such as paywalled O’Reilly Media books), compound the scrutiny.
Why This Matters:
The convergence of empirical results, legal analysis, and public concern is reshaping AI governance. With strong indications that OpenAI’s models memorized copyrighted material, including works under active legal protection, the debate over acceptable AI training practices is no longer hypothetical. Legal rulings influenced by these findings could establish precedents that reshape how models are built, how companies license data, and how users interact with generative tools. The future of ethical AI development now hinges on transparency, informed consent, and legal clarity over the boundaries of machine learning.
Explore AI ethics via Aristotle’s philosophy, focusing on human-centered design, enriched ethical values, and democracy’s role in AI development, as detailed in the “Lyceum Project” white paper.
Read a comprehensive monthly roundup of the latest AI news!