Jump to Sections
 Pioneering Cardiac Care: The Deep Learning Breakthrough of HeartBEiT
In the realm of cardiovascular care, a groundbreaking development has emerged in the form of HeartBEiT. This innovative deep learning model interprets Electrocardiograms (ECGs) with an unprecedented accuracy, providing clinicians with essential insights into cardiac health.
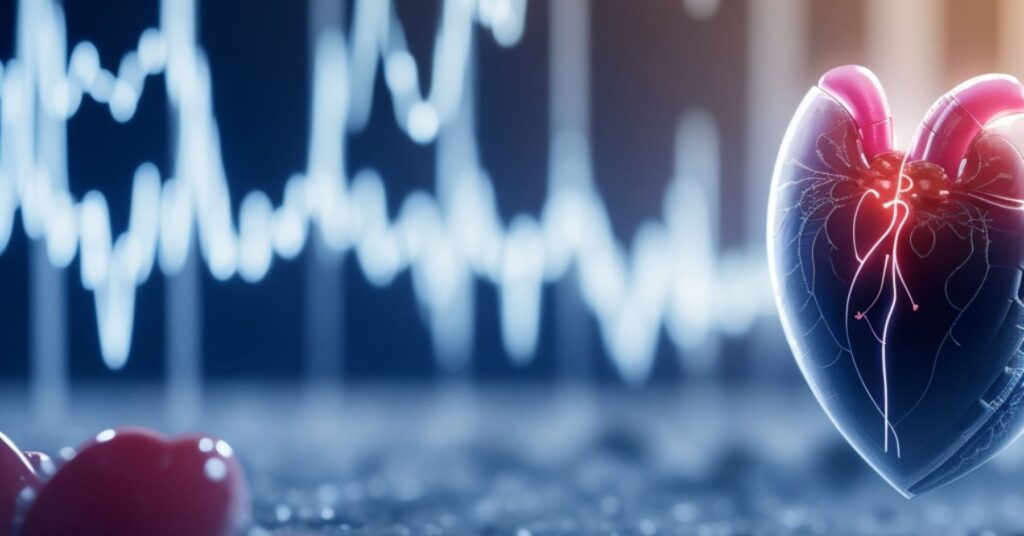
The HeartBEiT Innovation: A New Era in Cardiac Diagnostics
HeartBEiT introduces a revolutionary approach to cardiac diagnostics, underpinned by the power of deep learning.
Through the application of sophisticated algorithms, HeartBEiT unlocks the potential of ECG data for precise medical interpretation.
Beyond Conventional Methods: HeartBEiT’s Performance
Extensive comparisons have highlighted HeartBEiT’s superior performance over conventional diagnostic methods.
Significantly, this performance enhancement is achieved with remarkable efficiency, even when operating with smaller training datasets.
The key to HeartBEiT’s efficiency with smaller datasets is its use of transfer learning:
- The model fine-tunes a general-purpose image classifier (ResNet-18) on the MIT-BIH arrhythmia dataset, rather than training from scratch.
- This transfer learning approach allows HeartBEiT to leverage the knowledge gained from pre-training on large, general image datasets and adapt it to the ECG domain, even with limited data.
Comparison to Convolutional Neural Networks (CNNs)
- HeartBEiT outperforms conventional CNN-based approaches for ECG analysis, which typically require larger training datasets to achieve good performance.
- This highlights the efficiency of HeartBEiT’s transfer learning approach compared to training models from scratch on limited ECG data.
Modeling Approach
Leveraging Transformers vs. Convolutional Neural Networks (CNNs)
- HeartBEiT uses a transformer-based architecture, which treats ECG data as a “language” to be understood, rather than the more common CNN-based approaches.
- This allows HeartBEiT to better capture the complex, sequential relationships within ECG signals, compared to the more spatially-focused CNNs.
Specialization to ECG Data
- While CNNs are often pre-trained on large, general image datasets, HeartBEiT is specifically pre-trained on a large corpus of ECG data from the Mount Sinai Health System.
- This specialized pre-training allows HeartBEiT to better learn the unique patterns and features within ECG signals, compared to generic CNN models.
Transparent Insights
- Unlike many “black box” machine learning models, HeartBEiT is designed to provide transparent insights into its decision-making process.
- The model can identify specific ECG elements that contribute to its diagnoses, allowing clinicians to better understand the underlying pathology.
- This contrasts with the more opaque explanations typically provided by CNN-based models.
Empowering Clinicians with Clear Insights
HeartBEiT not only diagnoses but also empowers clinicians with transparent insights.
The model identifies specific ECG elements contributing to diagnoses, allowing for a comprehensive understanding of its decision-making process.
The Impact of Deep Learning in Healthcare Evolution
HeartBEiT signifies the transformative potential of deep learning in healthcare innovation.
Other deep learning applications are emerging, with potential for reshaping diagnostic paradigms across medical fields.
Shaping Cardiac Care’s Future: HeartBEiT’s Legacy
HeartBEiT’s impact extends beyond diagnostics, offering a new era of personalized cardiac healthcare.
As technology evolves, models like HeartBEiT will play a pivotal role in shaping a patient-centric healthcare landscape.
Integration with Clinical Workflows
Integrating new AI/ML models like HeartBEiT into clinical practice can be challenging, but there are strategies to facilitate adoption:
- Using a small team of nurses to communicate the model’s outputs to a larger group of physicians can help mitigate issues like “alert fatigue” that can arise from direct alerts to providers.
- Developing easy-to-use interfaces, like tablet applications, can make the model more accessible to clinicians.
- Providing training and education about how the model works can build trust and understanding among healthcare teams.
Key Takeaway
HeartBEiT stands as a testament to the potential of deep learning in transforming cardiac diagnostics. By converting complex ECG data into actionable insights, HeartBEiT paves the way for more accurate, efficient, and patient-centered cardiac care.